Machine learning model bests MELD score for predicting mortality in hepatic encephalopathy
Key takeaways:
- Artificial neural networks model better predicted 28-day mortality in hepatic encephalopathy vs. MELD, MELD-Na score.
- Independent risk factors – mechanical ventilation and acute kidney injury – crucial for predicting mortality.
A machine learning model using artificial neural networks demonstrated “superior performance” compared with MELD score for predicting 28-day mortality among patients with hepatic encephalopathy, according to data in BMC Gastroenterology.
“Though hepatic encephalopathy (HE) patients have improved outcomes over the past decade, several studies have indicated that the prognosis and quality of life still remain poor,” Zhe Zhang, from the department of gastroenterology at Tangdu Hospital in China, and colleagues wrote. “Specifically, HE typically heralds hepatic decompensation, and its development is usually associated with high morbidity, implying the need for liver transplantation.”
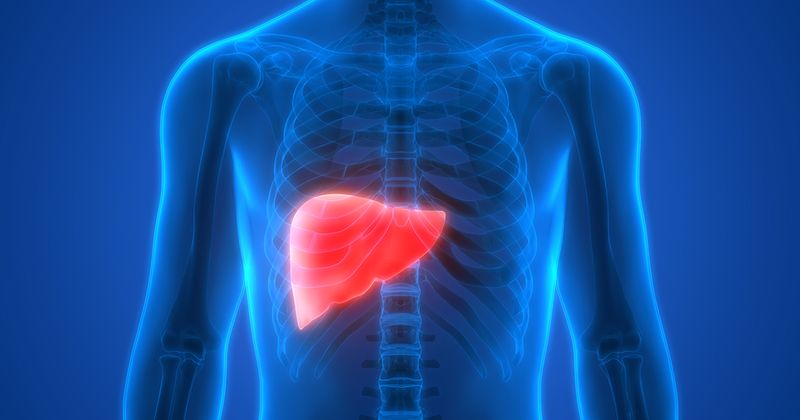
The researchers noted: “it is critical to determine an easily accessible and simple model to estimate the risk of 28-day mortality in patients with HE. Many existing scoring systems have been used to evaluate the prognosis of liver cirrhosis patients, but none of them are targeted at HE patients.”
In a retrospective cohort study, the researchers trained and validated machine learning models to predict mortality among 601 patients with HE from the Medical Information Mart for Intensive Care database; among these patients, 18.64% died within 28 days.
Zhang and colleagues used four different machine learning algorithms to develop their models: artificial neural networks, gradient boosting machine, ‘random forest’ and ‘bagged trees’ algorithms. Independent risk factors were identified as acute physiology score III (APSIII), sepsis-related organ failure assessment (SOFA), international normalized ratio (INR), total bilirubin (TBIL), albumin, blood urea nitrogen (BUN), acute kidney injury (AKI) and mechanical ventilation.
Results showed the neural networks model exhibited the highest area under the curve ([AUC] = 0.837; 95% CI, 0.774-0.901) while ‘bagged trees’ demonstrated the lowest discriminative ability (AUC = 0.741; 95% CI, 0.654-0.829). Further, the neural networks model was also “well calibrated,” which indicated better prediction for 28-day mortality and bested previously established MELD and MELD-Na score AUCs of 0.728 (95% CI, 0.677-0.779) and 0.711 (95% CI, 0.658-0.765), respectively.
“We demonstrated that APSIII, SOFA, mechanical ventilation, INR, TBIL, albumin and AKI are crucial for predicting 28-day mortality,” Zhang and colleagues wrote. “The model in our study had superior performance to MELD score or MELD-Na score predicting 28-day mortality. In the future, real-time prediction of mortality risk among HE patients might be realized, which, in turn, will optimize treatment to improve clinical prognosis.”