AI system distinguishes UC remission, activity with up to 87% accuracy
A computer-aided diagnosis system successfully distinguished disease remission from activity and predicted the risk for subsequent flare among patients with ulcerative colitis, according to results published in Gastroenterology.
“Computer-aided diagnosis (CAD) systems based on artificial intelligence (AI) are increasingly used to simplify and standardize the evaluation of medical imaging. ... These technologies hold promise to enhance assessment, simplify interpretation and resolve discrepancies between pathologists,” Marietta Iacucci, MD, PhD, FASGE, AGAF, of the Institute of Immunology and Immunotherapy at the University of Birmingham and the University College Cork, and colleagues wrote. “To the best of our knowledge, in the field of UC pathology, only two AI models have been developed.”
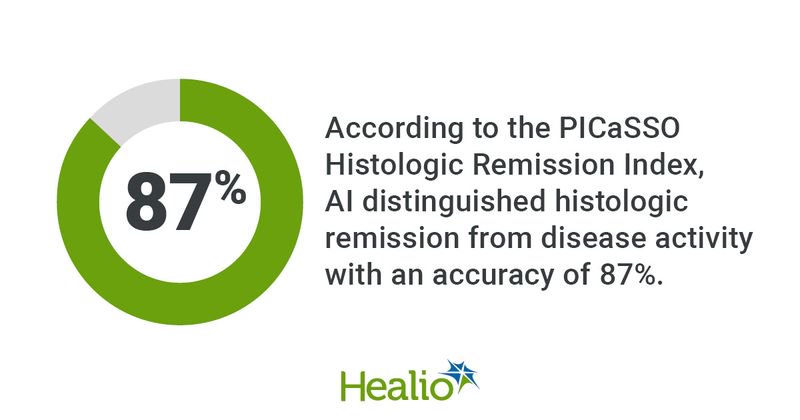
Seeking to develop and validate a CAD system to assess UC biopsies and aid prognosis, Iacucci and colleagues evaluated 535 digitized biopsies from 273 patients with UC (40.7% women; mean age, 48.1 years). Of those, researchers used a subset of 118 biopsies to train the model, 42 to calibrate it and 375 to test it. They also used 154 biopsies from 58 patients for external validation.
Disease activity was defined by the following histologic indices: PICaSSO Histologic Remission Index (PHRI), Robarts Histologic Index (RHI) and Nancy Histologic Index (NHI).
According to PHRI, the CAD system distinguished histologic remission from disease activity with a sensitivity of 89% (95% CI, 0.84-0.94), a specificity of 85% (95% CI, 0.8-0.89), a positive predictive value (PPV) of 75% (95% CI, 0.69-0.8), a negative predictive value (NPV) of 94% (95% CI, 0.9-0.96), an accuracy of 87% (95% CI, 0.83-0.9) and area under the receiver operating curve (AUROC) of 87% (95% CI, 0.83-0.9).
Using RHI and NHI cutoffs, the system differentiated histologic remission from disease activity with sensitivities of 94% (95% CI, 0.87-0.98) and 89% (95% CI, 0.81-0.97), respectively; specificities of 76% (95% CI, 0.71-0.81) and 79% (95% CI, 0.73-0.83); PPVs of 53% (95% CI, 0.48-0.58) and 60% (95% CI, 0.54-0.65); NPVs of 98% (95% CI, 0.95-0.99) and 95% (95% CI, 0.92-0.97); accuracies of 80% (95% CI 0.76-0.84) and 81% (95% CI, 0.77-0.85); and AUROCs of 85% (95% CI, 0.82-0.89) and 86% (95% CI, 0.83-0.9).
Researchers reported hazard ratios for disease flare-ups between histological activity/remission groups were 3.56 for pathologist-assessed PHRI and 4.64 for AI-assessed PHRI. They confirmed all outcomes in the external validation cohort.
“Our CAD system in real-time accurately distinguished disease remission from activity as defined by PHRI, RHI and NHI and provided a good prediction of the corresponding endoscopic activity and the risk of flare. These results were confirmed in an external validation cohort,” Iacucci and colleagues concluded. “Future directions are to include dysplasia detection and to combine histologic and endoscopic AI models into an integrated tool to further improve disease monitoring and prediction.”