Machine learning model accurately identifies tertiary lymphoid structures up to nearly 98%
Click Here to Manage Email Alerts
A machine learning model may aid the detection and classification of tertiary lymphoid structures associated with gastrointestinal cancers based on routine tissue slides, according to research in JAMA Network Open.
“Currently, the most common and well-accepted approach to [tertiary lymphoid structure] detection is tissue staining for markers of immune cell lineages by multiplex immunohistochemistry or immunofluorescence techniques,” Zhe Li, BS, of the School of Computer Science and Engineering at Northwestern Polytechnical University in China, and colleagues wrote. “On the other hand, hematoxylin-eosin (H&E) staining is widely available and remains the clinical standard in histopathology.
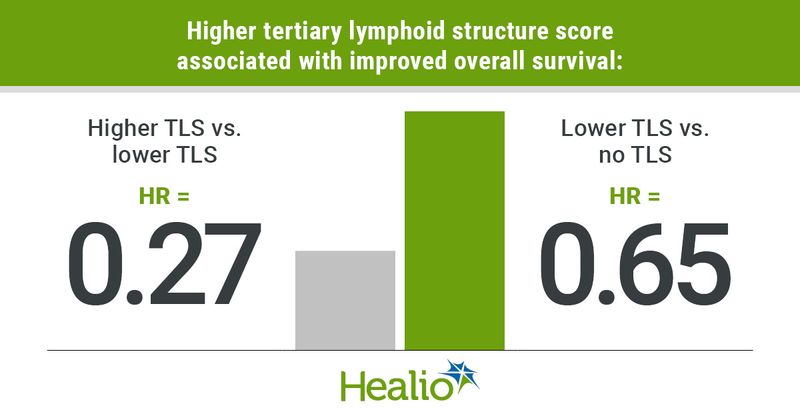
“Several groups have evaluated TLSs in routine H&E-stained slides based on pathologist assessment. However, this approach is time and labor intensive. ... There is an unmet need for validated methods that allow standardized and quantitative evaluation of TLSs in H&E images.”
In a multicenter, international diagnostic/prognostic study, Li and colleagues developed a machine learning model for automated and quantitative evaluation of TLSs based on routine H&E-stained whole-slide images. They enrolled 1,924 patients (median age, 57-68 years) from seven independent cohorts with esophageal, gastric, colon, rectal, liver or pancreatic cancer.
Outcomes of interest included diagnostic accuracy for classification of maturation stages and association of TLS score with survival.
According to results, the machine learning model demonstrated high accuracy in the testing data set of TLS stage 1 (97.7%; 95% CI, 96.4-99), stage 2 (96.3%; 95% CI, 94.6-98) and stage 3 (95.7%; 95% CI, 93.9-97.5), with TLSs detected in 40% of esophageal cancers and up to 75.6% of gastric cancers.
Results also showed higher TLS scores were associated with improved overall survival compared with lower TLS scores (HR = 0.27; 95% CI, 0.18-0.41); in addition, patients with lower TLS score had better survival outcomes vs. those without TLSs (HR = 0.65; 95% CI, 0.56-0.76).
After adjusting for clinicopathologic variables and tumor-infiltrating lymphocytes, TLS score remained an independent predictor for survival in all seven cohorts (eg, for colon cancer: HR = 0.11; 95% CI, 0.02-0.47).
“In this multicenter diagnostic/prognostic study of 1,924 patients, we developed a machine learning-based computational tool for automated detection and quantitative evaluation of TLSs on routine H&E slides and confirmed the association of TLSs with survival in gastrointestinal cancers,” Li and colleagues concluded. “The proposed TLS scoring system may complement the current staging system and be associated with refinements in risk stratification.”
They added, “Prospective validation studies may be warranted to confirm that our results are reproducible and generalizable across broader patient populations.”