Machine-learning models help differentiate alcohol-associated hepatitis, acute cholangitis
Alcohol-associated hepatitis and acute cholangitis were accurately diagnosed by machine-learning algorithms, which also outperformed physicians in distinguishing the two diseases, according to a study in Mayo Clinic Proceedings.
“Our study is a proof of concept demonstrating how [machine-learning algorithms] using a few simple variables and routinely available structured clinical information may serve as highly potent prediction tools,” Joseph C. Ahn, MD, a third-year gastroenterology and hepatology fellow at Mayo Clinic in Rochester, and colleagues wrote. “With a similar approach to our [acute cholangitis] vs. [alcohol-associated hepatitis] prediction model, it might be feasible to develop comprehensive MLA-based software that can guide medical providers treating patients with liver enzyme abnormalities and aid in decision-making.”
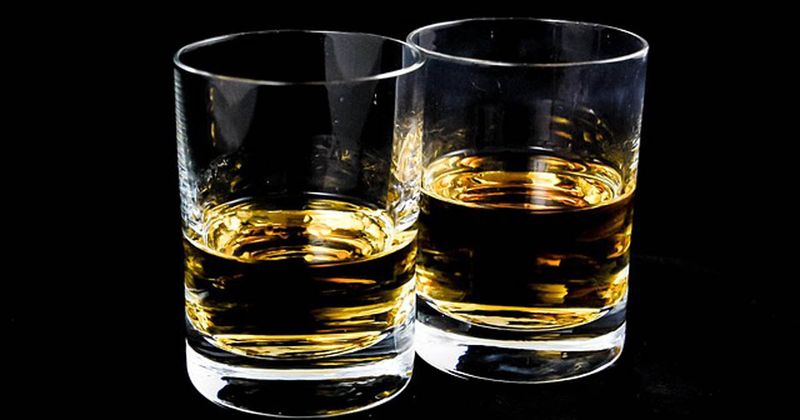
Ahn and colleagues identified 194 adult patients with acute cholangitis (AC) and 260 with alcohol-associated hepatitis (AH) who were admitted to Mayo Clinic between 2010 and 2019. At the time of admission, 10 routinely available laboratory values were collected from each patient: white blood cell count, hemoglobin, mean corpuscular volume, platelet count, aspartate aminotransferase, alanine aminotransferase, alkaline phosphatase, total bilirubin, direct bilirubin and albumin.
Researchers used these values to train eight MLAs to distinguish AC from AH, which included decision tree, naive Bayes, logistic regression, k-nearest neighbor, support vector machine, artificial neural networks, random forest and gradient boosting. The most relevant 5-variable subsets were identified using Jensen-Shannon divergence and Hilbert-Schmidt independence criterion (HSIC).
Researchers externally validated the results using an ICU cohort and compared MLA predictive performance with that of 143 physicians, who were presented the same 10 laboratory variables and completed an online survey.
According to study results, all eight MLAs demonstrated excellent performance in distinguishing AC from AH with accuracies up to 0.932 and area under the curve (AUC) up to 0.986. Similar accuracy also was observed in external validation up to 0.909 and AUC up to 0.970.
The 5-variable subsets with the highest J-S divergence and HSIC were mean corpuscular volume, AST, ALT, total bilirubin and direct bilirubin, and mean corpuscular volume, albumin, ALT, total bilirubin and direct bilirubin, respectively.
Of the eight MLAs, six had accuracies above 0.90, with the lowest performing model still demonstrating an accuracy of 0.868, while physicians had a mean accuracy of 0.79.
“The study highlights the potential for machine-learning algorithms to assist in clinical decision-making in cases of uncertainty,” Ahn said in a related Mayo Clinic press release. “For patients, this would lead to improved diagnostic accuracy and reduce the number of additional tests or inappropriate ordering of invasive procedures, which may delay the correct diagnosis or subject patients to the risk of unnecessary complications.”
The authors added, “If made easily accessible in the form of an online calculator or a smartphone application, our MLAs might also be helpful to midlevel providers or subspecialists who may not be too familiar with how to approach an acutely ill patient with liver enzyme abnormalities.”
Reference:
- Machine-learning algorithms can help health care staff correctly diagnose alcohol-associated hepatitis, acute cholangitis. newsnetwork.mayoclinic.org/discussion/machine-learning-algorithms-can-help-health-care-staff-correctly-diagnose-alcohol-associated-hepatitis-acute-cholangitis/. Published June 29, 2022. Accessed July 8, 2022.