Open-source algorithm may detect nailfold changes in systemic sclerosis
PHILADELPHIA — Vision Transformer, an open-source computer algorithm, may detect changes in nailfold capillaroscopy images in patients with systemic sclerosis, according to data presented at ACR Convergence 2022.
“We all know that a nailfold capillaroscopy is able to detect small vessel abnormalities and its role is well established in the diagnosis and prognosis of systemic sclerosis,” Alexandru Garaiman, a PhD candidate at the University of Zurich and a rheumatology resident at University Hospital, Zurich, told attendees. “Therefore, an adequate assessment of nailfold capillaroscopy is essential.
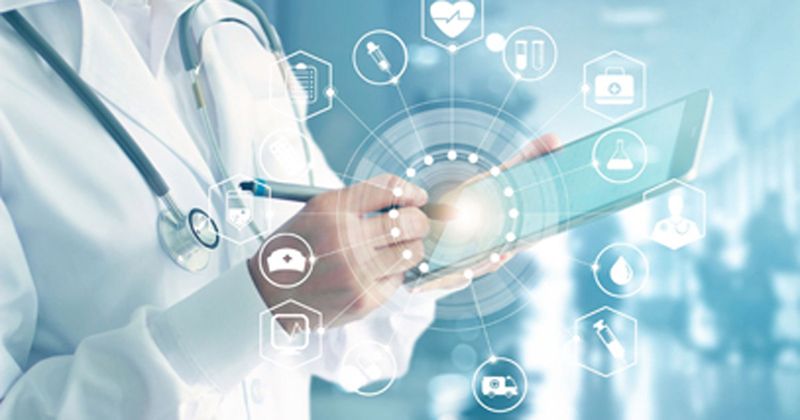
“However, this comes sometimes, or in most times, with some challenges, such as the need for assessor training, operator bias, observer bias or simply the assessment itself is time consuming, especially if we aim to consider two images per finger and we want to examine eight fingers from a patient,” he added. “All this has led to an interest in automating and standardizing capillaroscopy image assessment, using one of the most recent and advanced computer vision algorithms at our disposal, for free, and that requires no feature engineering — the Vision Transformer.”
To assess the performance and reliability of a Vision Transformer model in reading nailfold capillaroscopy (NFC) images, and to compare its performance to that of human rheumatologists, Garaiman and colleagues used 17,126 images from 289 patients with SSc. The images were sourced from patients enrolled in the European Scleroderma Trials and Research group (EUSTAR) and Very Early Diagnosis of Systemic Sclerosis (VEDOSS) local registries.
Routine NFC images — including all NFC images available of digit II to V of both hands, irrespective of any image artefacts — from patients aged 18 years or older with visits between 2012 and 2021, who also fulfilled either the 2013 American College of Rheumatology/EULAR classification criteria for SSc or the preliminary criteria for VEDOSS, were included.
The model was trained to identify NFC signs of microangiopathy, including enlarged capillaries, defined as an apex diameter between 20 m and 50 m; giant capillaries, defined as greater than 50 m; loss of capillaries, defined as capillaries measuring less than 7 mm; and microhemorrhages. If extremely lowered capillary density — 3 mm or less — or giant capillaries were detected, that image was labeled positive for “presence of scleroderma pattern,” the researchers wrote.
The researchers assessed the model’s performance via a cross-fold (k = 5 folds) validation setting, with the treating physician used as the “gold standard,” according to the researchers. Area under the ROC curve (AUC) indicated performance. Garaiman and colleagues created, from the first fold, a randomly sampled reliability set, which was used to compare the NFC assessment performance of four human rheumatologists, which included three invited and trained annotators and the treating physician, with that of the model.
According to the researchers, the Vision Transformer model demonstrated good mean performance in identifying the NFC changes in all five folds. The AUC ranged from 81.8% to 84.5% for detecting microangiopathic changes, and 83.2% for finding scleroderma pattern.
In the reliability set, which included 464 images, the researchers reported highest performance for diagnosing giant capillaries, with an AUC of 92.6%, followed by detecting enlarged capillaries, with an AUC of 90.2%. The researchers additionally reported good AUCs in demonstrating capillary loss, with an AUC of 86.7%, and microhemorrhages, with an AUC of 85%. The model achieved a similarly good performance in detecting the presence of scleroderma pattern, with an AUC of 88.5%.
However, the human rheumatologists achieved a generally higher performance in assessing NFC images, according to the researchers. That said, the model did outperform one rheumatologist in identifying capillary loss.
“When compared directly to the performance of the four rheumatologists, see saw that Vision Transformer does not perform worse than the human assessors — and it was quite a pleasant surprise that one of the rheumatologists was outperformed by it when it was tasked to predict capillary loss from the nailfold capillaroscopy images,” Garaiman said. “In addition, I would also like to mention that the Vision Transformer can assess 16 nailfold capillaroscopy images in less than 3 seconds, which should make Vision Transformer very attractive for the clinical setting.”