Q&A: AI tool identifies a larger, ‘justifiable’ prevalence of long COVID
Key takeaways:
- An AI tool showed that approximately one-fifth of the general population may have long COVID.
- The AI tool showed greater accuracy vs. ICD-10 codes with less bias.
An AI-based algorithm accurately diagnosed unidentified cases of long COVID using patient health records, according to study results published in Med.
The findings also revealed a significantly higher percentage of the population experiencing long COVID symptoms than what has been previously reported.
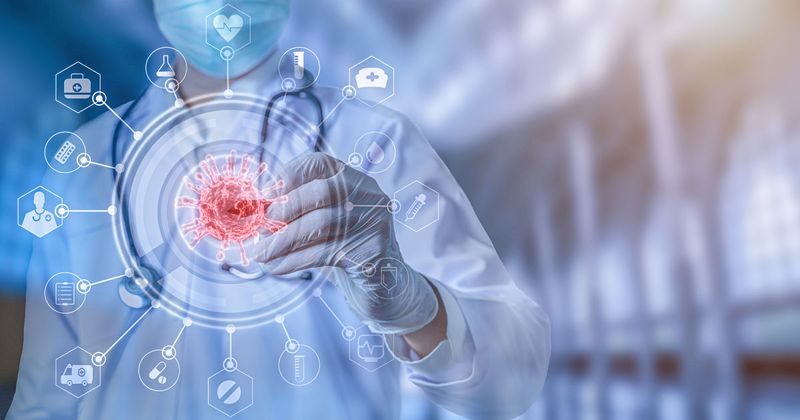
“Our AI tool could turn a foggy diagnostic process into something sharp and focused, giving clinicians the power to make sense of a challenging condition,” Hossein Estiri, PhD, a professor of medicine at Harvard Medical School, said in a press release. “With this work, we may finally be able to see long COVID for what it truly is — and more importantly, how to treat it.”
In the study, Estiri and colleagues developed their tool using the electronic health record data of over 295,000 patients across 14 hospitals and 20 community health centers and tested it in a cohort of over 24,000 patients.
They found the tool was 2.7% more accurate than ICD-10 codes while also being less biased.
Additionally, the algorithm estimated the prevalence of long COVID in the general population based on the results at 22.8%, much greater than previously estimated figures of around 7%.
Estiri spoke with Healio and talked about the inner workings of the AI-based tool, if it could be used for other conditions and more.
Healio: How does the AI tool work?
Estiri: We look at things that are associated with SARS-CoV-2 infection in a case-controlled study and we then look at the attention mechanism, which looks at how these electronic medical records are associated with each other temporally. For example, say coughs usually come around or after an influenza infection. Then comes the algorithm that applies these to individual patients. It looks at the individual patient and then looks at their health data longitudinally and adjudicates whether the condition that happened [within] 12 months of the SARS-CoV-2 infection can be considered long COVID.
We followed the National Academies’ definition of long COVID, which is that it has to be a diagnosis of exclusion. In addition to that, it also had to be a SARS-CoV-2 infection-associated chronic condition. On top of that, there is a fine-tuning algorithm that looks at a set of validated data. Essentially, it’s a bunch of algorithms working together — that’s what we refer to as precision phenotyping. It’s a novel way of trying to identify health care problems and phenotypes.
Healio: Did you expect the algorithm to perform as well as it did?
Estiri: As a scientist, I can say I neither expected it. nor was I surprised. You learn to expect the unexpected. I think that the improvement in accuracy is not the most important aspect of this work because it brought in a much, much larger cohort [with long COVID]. If you look at ICD-10 codes, there are a small number of patients who do have ICD-10 codes compared with what we identified. So, not only were we able to improve the accuracy, but we also captured a wider number of patients, which is [reflective of] an underestimated condition that is going on in the health care systems’ blind spots.
Healio: What are your thoughts on the prevalence of long COVID that the AI identified?
Estiri: Going back to the fact that I believe long COVID has largely been overlooked, I think that 22% is justifiable [for several reasons]. One is that we don’t fully understand what long COVID even is. It is not one singular, well-defined condition. It’s a patchwork of different things that are interactive with human biology. Another thing is where those [reports of] less than 10% prevalences usually come from ICD-10 code assignment to patients. The population that receives ICD-10 codes has a lot of access to care [and] is usually not representative of the general population. The same thing [applies to] surveys.
Our health care systems aren’t really equipped to assign long COVID ICD-10 codes but designed to focus on measurable problems and ways to treat it. They’re not designed to connect the dots, to go back and [look at] what could be the underlying roots of these issues. Because of all of that, I think that 10% is really an underestimation.
Healio: Could this algorithm be used for other conditions or diseases?
Estiri: Anything that is a diagnosis of exclusion [could apply] with the caveat that we need to consider there are some genetic underpinnings. If they’re not stored in the [medical record] data, it’s hard to understand them.
Healio: Where does further work on the algorithm go from here?
Estiri: There is the future research on long COVID, and then there is the future research on the algorithm and AI. On the long COVID side, I think we’re now doing studies that were not previously possible with the broad definitions of long COVID in different cohorts. I think the cohort that we had access to is going to allow collaboration with other [researchers] and allow our collaborators to do a lot of other studying into the genomics and other potential roots of long COVID. On the AI side, one of the areas that we are really interested in and doing research on is how can we make it more robust and include more confounding factors.
Healio: Do you have anything else to add?
Estiri: I think that primary care providers will be interested in looking at algorithmic solutions to things that take a lot of time, including diagnoses of exclusion. It’s hard [for them] with all the [lack of] time and burnout that they already have. So, such algorithms might be really interesting to them.
References:
- Azhir A, et al. Med. 2024;doi:10.1016/j.medj.2024.10.009.
- New medical AI tool identifies more cases of long COVID from patient health records. Available at: https://www.massgeneralbrigham.org/en/about/newsroom/press-releases/new-medical-ai-tool-identifies-more-cases-of-long-covid-from-patient-health-records. Published Nov. 8, 2024. Accessed Nov. 20, 2024.