Machine learning model demonstrates ‘excellent distinction’ between active, inactive UC
A machine learning model using the endoscopic Mayo Score was able to distinguish between active and inactive disease in ulcerative colitis, according to results presented at the Congress of the European Crohn’s and Colitis Organization.
“There are acknowledged limitations to endoscopic scoring of bowel inflammation in IBD, most notably the subjective nature of interpretation of the findings which can result in a number of challenges,” David T. Rubin, MD, AGAF, chief of gastroenterology, hepatology and nutrition at the University of Chicago Medicine, told attendees. “The well described discordance between symptoms and endoscopic findings and some remaining uncertainties in the prognostic implications of specific grades of inflammation to guide treatment.”
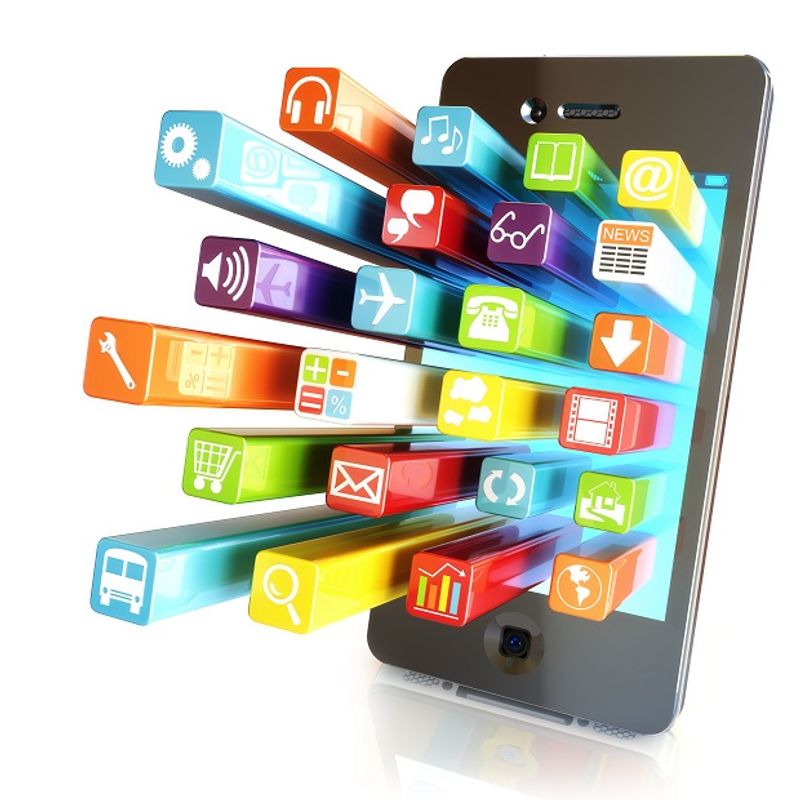
Source: Adobe Stock.
Unlike previous studies, in which machine learning models were focused on predicting how human readers would score disease activity in UC, Rubin noted that “the investigators in this study are using machine learning to build algorithms trained at the feature level of the endoscopic findings that replicate the two primary scoring systems used in Crohn’s and UC.”
Rubin reported that the primary dataset consisted of 793 full-length videos acquired from patients with UC (n = 249) who participated in a phase 2 clinical trial of mirikizumab for moderate to severe ulcerative colitis, with a single reader endoscopic Mayo Score (eMS). Machine learning workflow involved annotation, segmentation and classification, with human image classification and segmentation put through quality control judged by one of three IBD specialists, generating more than 60,000 eMS-relevant annotation labels.

The model was then assessed with a test set of 147 videos using the centrally read eMS and a consensus set of 94 test videos, in which centrally read eMS and annotator-reported eMS agreed without the need for adjudication.
“We have demonstrated a machine-learning predictive model of the endoscopic Mayo Score in ulcerative colitis using centrally read videos as our ground truth, and, separately, a second reading,” Rubin said.
According to study results, using the full test set, the machine learning model predicted inactive disease vs. active disease with an accuracy of 84%, a positive predictive value (PPV) of 80% and a negative predictive value (NPV) of 85%. In the smaller subset with centrally read eMS and annotator-reported eMS consensus, the model was able to predict inactive disease vs. active disease with an accuracy of 89%, a PPV of 87% and NPV of 90%.
For the secondary objectives, researchers found that, in the full set, the model predicted endoscopic healing and severe disease with an accuracy of 90% and 80%, PPVs of 44% and 86%, and NPVs of 95% and 86%, respectively. In the subset, the model predicted endoscopic healing and severe disease with an accuracy of 95% and 85%, PPVs of 86% and 82% and NPVs of 95% and 87%, respectively.
“We demonstrated excellent distinction between active and inactive disease, and a clear discrimination between other levels of endoscopic activity,” Rubin said. “We propose that this unique machine-learning might replace central reading in clinical trials, but I would also add that this may be very useful for future clinical practice and standardization of reporting and management decisions.”