Liquid biopsy assay model identifies high-risk CRC to avoid unnecessary surgeries
A novel risk-stratification model developed for noninvasive identification of T1 colorectal cancer may help avoid unnecessary surgeries for high-risk patients.
“Pending validation in future prospective studies, our findings highlight the potential clinical impact of our model for improved selection of patients with high-risk T1 CRC, which will reduce the overall burden of unnecessary surgeries and expense associated with these procedures, and improve the overall management of patients with this malignancy,” Yuma Wada, from the Center for Gastrointestinal Research, Baylor Scott & White Research Institute and Charles A. Sammons Cancer Center, Baylor University Medical Center, Dallas Texas, and colleagues wrote in Gastroenterology.
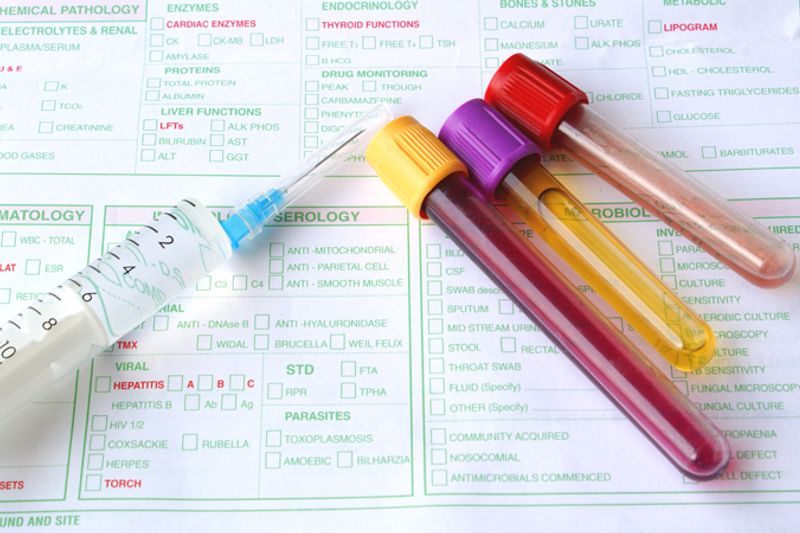
Wada and colleagues assessed 330 specimens from patients with high-risk T1 CRC. This included 188 serum samples from clinical cohorts (training cohort n = 46; validation cohort n = 142) and 142 matched formalin-fixed paraffin embedded (FFPE) samples.
“We recently reported use of tissue-based transcriptomic biomarkers (miRNA or mRNA) for identification of lymph node metastasis (LNM) in patients with invasive submucosal colorectal cancers (T1 CRC),” the investigators wrote. “In this study, we translated our tissue-based biomarkers into a blood-based liquid biopsy assay for noninvasive detection of LNM in patients with high-risk T1 CRC.”
The researchers developed an integrated transcriptomic model and a risk stratification model after conducting real-time quantitative reverse transcription polymerase chain reaction followed by logistic regression analysis.
They used expression profiling of a training cohort of LNM-positive and -negative serum specimens to determine the optimized transcriptomic panel of four miRNAs (miR-181b, miR-193b, miR-195 and miR-411) and five mRNAs (AMT, FOXA1, PIGR, MMP1 and MMP9). Investigators wrote this method “robustly identified patients with LNM (area under the curve = 0.86; 95% CI, 0.72–0.94).”
“Our risk-stratification model was more accurate than the panel and an independent predictor for identification of LNM (AUC = 0.9; univariate [OR] = 37.17, 95% CI, 4.48–308.35, P < .001; multivariate OR = 17.28, 95% CI, 1.82–164.07, P = .013),” Wada and colleagues wrote.
Wada and colleagues reported the model limited the potential of overtreatment to only 18% of patients, which is superior compared with the currently used pathological features (92%).