Automatic polyp detection shows promise for assisting colonoscopy
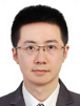
ORLANDO — Deep-learning, a computational strategy for automatic object detection in images and video, was effective for automatically detecting polyps during colonoscopy, according to new research presented at the World Congress of Gastroenterology at ACG 2017.
“While deep-learning has achieved some success in diabetic retinopathy detection as published in JAMA, and skin cancer classification as published in Nature earlier this year, ideally with an endoscopist-level automatic polyp detection system, we could enjoy accurate real-time visual assistance on the monitor,” Pu Wang, MD, Sichuan Academy of Medical Sciences and Sichuan Provincial People’s Hospital in China, said during his presentation.
To validate the clinical use of deep-learning for polyp auto-detection during colonoscopy, Wang and colleagues developed a deep-learning algorithm using 5,545 colonoscopy images collected from 1,290 patients in whom polyps were detected from 2007 through 2015. Endoscopists annotated images in the development data set, outlining the boundaries of each polyp.
They validated the algorithm using 27,113 colonoscopy images collected in 2016 (5,541 with polyps from 1,138 patients [mean age, 57 years; 37% women]) and 289 colonoscopy videos collected in 2017, which were used for simulated real-time video validation (156,337 frames, 60,914 with polyps from 151 patients with polyp history [mean age, 57 years; 42% women]). A panel of five endoscopists evaluated the algorithm’s accuracy for detecting polyps.
For the still-frame analysis, “the system predicted ... polyp presence in each validation image,” Wang said. “For the simulated real-time video analysis, a video player simulated real-life colonoscopy procedures, and in real-time the system acquired the [video] streams processed with the algorithm and displayed [the results].”
Researchers excluded images if polyps lacked histology, if there was unqualified intestinal preparation or inadequate air inflation, or a giant colorectal cancer mass exceeding 2 cm, Wang noted.
Overall, the still-frame analysis included 1,495 polyps (1,044 adenomas), while the simulated real-time video analysis included 138 polyps (77 adenomas).
For Yamada type 1 isochromatic polyps and those larger than 0.5 cm, the still-frame analysis showed the algorithm performed with 96.93% precision and 91.65% sensitivity. For all polyps, it performed with 94.38% sensitivity, 94.28% specificity, and an area under the receiver operating characteristic curve (AUROCC) of 0.991.
“These numbers are slightly different from the abstract because we have excluded all those validation images without histology confirmation,” Wang noted.
The simulated real-time video analysis showed it performed with 91.64% sensitivity, 96.3% specificity, a 100% detection rate and 26.86% refresh rate. Additionally, it performed with latency (how many frames the system requires to detect a polyp after its first appearance) of less than two frames on average, temporal coherence (time consistency of detection) of about 89%, and a refresh rate above 26 frames per second.
These results were like the still-frame analysis, and the numbers “showed adequate reaction speed and detection accuracy for real-time and real-world clinical application,” Wang said.
Study limitations include some false alarms on water bubbles and annular vessels, exclusion of non-polypoid precancerous lesions, and that the validation was not performed in a real-world clinical setting, he noted.
He concluded that these data show he and colleagues have improved the system’s performance, adding that they have also performed a randomized comparative study in September. – by Adam Leitenberger
Reference:
Wang P, et al. Abstract 4. Presented at: World Congress of Gastroenterology at American College of Gastroenterology Annual Scientific Meeting; Oct. 13-18, 2017; Orlando, FL.
Disclosures: The researchers report no relevant financial disclosures.