Automated nailfold analysis distinguishes systemic sclerosis from normal capillaries
A fully automated analysis of nailfold images was able to distinguish patients with systemic sclerosis from those with normal capillaries at least as well as human experts, according to data published in Rheumatology.
“Abnormal capillaries are highly suggestive of an underlying systemic sclerosis (SSc)- spectrum disorder, whereas normal capillaries (in the absence of other clinical features pointing to connective tissue disease) are reassuring,” Praveen Gurunath Bharathi, MD, of the Center for Imaging Sciences in the division of informatics at the University of Manchester, in the United Kingdom, and colleagues wrote. “However, results of a recent U.K. survey suggest that almost 60% of rheumatologists do not currently perform or have access to capillaroscopy in their own departments.”
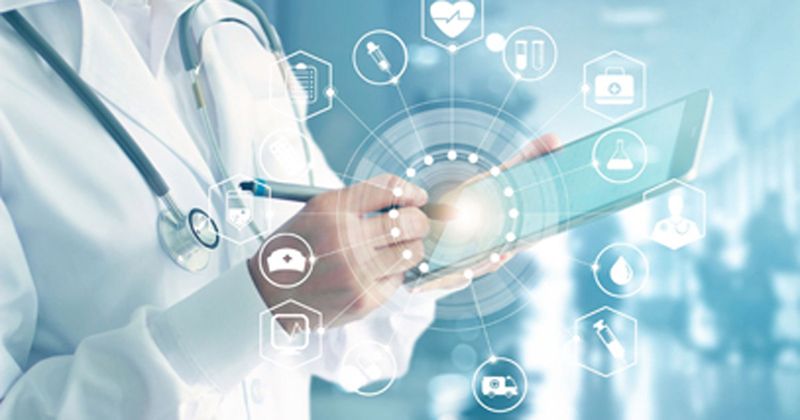
To develop and validate a fully automated system capable of interpreting nailfold capillaroscopy images, Bharathi and colleagues imitated strategies employed by expert interpreters via deep learning networks. These networks were used to identify each capillary in the distal row of vessels and perform morphological measurements. The researchers then combined measurements from multiple fingers to derive the probability of SSc.
The researchers trained the system on a series of high-resolution images from a total of 111 individuals. They later tested the system with another 198 individuals who were not part of the training set, with 132 imaged at high resolution and 66 imaged using a low-cost digital microscope. In each group, approximately half had confirmed SSc, while the other half were either healthy controls or demonstrated primary Raynaud’s phenomenon. All images were captured at a magnification power of x200 from roughly 10 mm.
To establish a benchmark with which to measure the performance of the deep learning software, the researchers also estimated the performance of SSc experts. After comparing the automated probabilities of the presence of SSc with the confirmed clinical diagnosis of patients in the sample, the researchers calculated receiver operating characteristic curves (ROCs).
According to the researchers, the deep learning system achieved results comparable to the expert human interpreters. For the high-resolution testing group, the area under the ROC was 97% (90% CI, 94%-99%). In this group, sensitivity and specificity were equal, at 91% (90% CI, 86%-95%). In the testing group imaged with the low-cost digital microscope, the area under the ROC was 95% (90% CI, 88%-99%). For these, the sensitivity and specificity were also equal, at 89% (90% CI, 82%-95%). Consensus reached by experts demonstrated 82% sensitivity and 73% specificity.
We have developed a complete automated system that analyses nailfold capillary images, and returns a subject-level probability of SSc, based on microvascular morphology,” Bharathi and colleagues wrote. “We have validated the system using high-quality images from subjects not involved in training the system, and have shown it can discriminate between patients with SSc and subjects with normal capillaries at least as well as human experts.
“Using a deep learning approach holds promise for accurate separation between patients with SSc and those with normal capillaries, bypassing the need for human interpretation,” they added. “Although not included in this study, it may also be applicable to secondary [Raynaud’s phenomenon] more generally, for example in [dermatomyositis] and [systemic lupus erythematosus]. Taking an approach that mimics the interpretation strategies of human experts, as we have here, can provide the basis for rheumatologist-friendly explanation of the results.”