Machine learning model using biomarkers, DAS28 data predicts MTX response in early RA
A machine learning model combining pharmacogenomic biomarkers with baseline DAS28 joint count predicted methotrexate response in early rheumatoid arthritis, according to data published in Arthritis Care & Research.
“Methotrexate remains the preferred initial DMARD for RA but many patients don’t fully benefit from treatment with methotrexate due to inadequate control of RA disease activity or side effects,” Elena Myasoedova, MD, PhD, of the Mayo Clinic, in Rochester, Minnesota, told Healio Rheumatology. “Predicting response to methotrexate early — ie, at 3-month follow-up — would inform timely escalation of treatment for improved control of RA disease activity, avoiding unnecessary risk of methotrexate-related side-effects in early RA.”
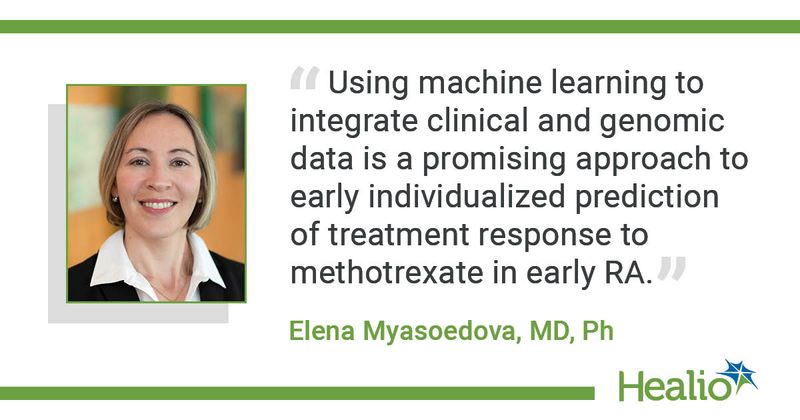
“Clinically useful predictive algorithms effectively identifying patients with early RA who are likely to respond to methotrexate are lacking,” she added. “There is a need to develop a precision medicine approach toward treatment in RA. In my everyday rheumatology practice, patients with newly diagnosed RA frequently ask, ‘Will this medication [methotrexate] help me?’ and ‘Is methotrexate the right medication for me?’ This study seeks to address these questions.”
To address this knowledge gap and apply machine learning to identify several clinical and genomic biomarkers that can predict MTX response in patients with early RA, Myasoedova and colleagues started with data from the combined Mayo Clinic and Pharmacogenetics of Methotrexate in Rheumatoid Arthritis (PAMERA) consortium. Demographic, clinical and genomic data from a total of 643 patients with early RA were subdivided into training and validation cohorts. Genomic data included 160 single nucleotide polymorphisms (SNPs) previously linked to RA or MTX metabolism.
The researchers trained supervised machine learning methods with five-repeats and 10-fold cross-validation with the training cohort, which included data from 336 patients. Meanwhile, prediction performance was assessed using the validation cohort, which included 307 patients. The researchers defined “good” or “moderate” MTX response at 3-month follow-up based on EULAR criteria.
According to the researchers, supervised machine learning methods that combined age, sex, smoking status, rheumatoid factor, DAS28 joint count and the 160 SNPs were able to predict EULAR response at 3 months. The area under the receiver operating curve was 0.84 (P = .05) in the training cohort, while the validation cohort achieved a prediction accuracy of 76% (P =.05), with a sensitivity of 72% and a specificity of 77%.
The intergenic SNPs rs12446816, rs13385025, rs113798271, and ATIC (rs2372536) demonstrated variable importance above 60 and, combined with baseline DAS28, were among the best predictors of MTX response in early RA.
“Pharmacogenomic biomarkers combined with baseline DAS28 can be useful in predicting response to methotrexate in patients with early RA,” Myasoedova said. “Applying machine learning methods to predict treatment response holds promise for guiding effective RA treatment choices, including timely escalation of RA therapies. Expanding research in individualized medicine in rheumatology may facilitate patient-tailored prediction of response to anti-rheumatic treatments in patients with RA.”
“Using machine learning to integrate clinical and genomic data is a promising approach to early individualized prediction of treatment response to methotrexate in early RA,” she added. “Evaluating the clinical impact and cost-effectiveness of our predictive model will be needed prior to implementing in clinical practice.”