Artificial neural network accurately detects sacroiliitis in suspected axial SpA
Click Here to Manage Email Alerts
A newly developed artificial neural network can accurately detect radiographic sacroiliitis in patients with suspected axial spondyloarthritis, according to data presented at ACR Convergence 2021.
Based on the data, this trained convolutional neural network could potentially assist non-specialized centers in interpreting sacroiliac radiographs as part of the diagnostic process, the presenter noted.
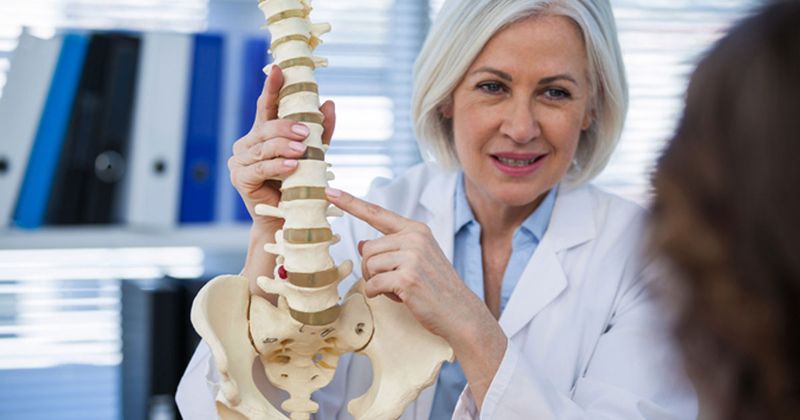
“The problem of the diagnosis of this disease is we need to differentiate nonspecific back pain or mechanical induced back pain from back pain caused by spondyloarthritis, caused by inflammation,” Denis Poddubnyy, MD, MSc, of Charité-Universitätsmedizin Berlin, told attendees at a virtual press conference. “In daily clinical practice, it is often virtually impossible to diagnose the inflammatory disease without visualization of inflammation. There is a big problem of X-rays of sacroiliac joints, and this is really poor reliability. For one image, you can get different opinions, ranging from absolutely nothing to sacroiliitis.”
For this reason, Poddubnyy and colleagues turned to artificial intelligence and convolutional neural networks, which can “imitate the work of the human brain,” he said.
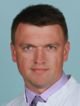
“You give the neural network an image and corresponding label,” Poddubnyy said. “For example, an image of a patient without radiographic sacroiliitis and one with radiographic sacroiliitis, and then the neural network, with substantial number of those images, tries to extract features, which result in a conclusion of the presence or absence of radiographic sacroiliitis. If you have just two or three images, there is a substantial risk that it doesn't work, but if you have thousands of images, with corresponding reliable labels, then it might work.”
To assess the performance of convolutional neural networks in detecting radiographic sacroiliitis, Poddubnyy and colleagues analyzed sacroiliac radiographs of patients with chronic back pain and suspected axial SpA who presented to a rheumatologist as part of the OptiRef study. In all, 361 patients received a structured work-up from a rheumatologist that resulted in a final positive or negative diagnosis.
The researchers examined sacroiliac joint radiographs according to the modified New York criteria, with the consensus judgement of the rheumatologist and radiologist on the presence of radiographic sacroiliitis used as a reference. They then compared this reference judgement to the predictions of the deep convolutional neural network’s inference on all available pelvic radiographs.
Pelvic radiographs from 340 of the patients were available for the neural network’s evaluation. Among these patients, 110 were ultimately diagnosed with axial SpA, including 61 with radiographic and 49 with non-radiographic disease. The researchers used a balanced cutoff of 0.724 for the model predictions, previously derived from the training and validation.
According to the researchers, the deep neural network achieved a sensitivity of 79% for the diagnosis of radiographic axial SpA, with a detection specificity of 94% for radiographic sacroiliitis. The area under the receiver operating characteristics curve for the prediction of definite radiographic sacroiliitis was 88%.
There was an 85% absolute agreement on the classification of radiographic or non-radiographic axial SpA between the neural network and the reference.
“Overall, we see that using the artificial intelligence approach, this trained neural network, is one of the potential ways to increase the reliability of assessment, even of such a difficult imaging modality like X-rays of sacroiliac joints,” Poddubnyy said. “We are, of course, very much looking forward to the next step of this work, and this will include evaluation of MRI sacroiliac joints.”