Artificial neural networks detect radiographic sacroiliitis, aid axial SpA diagnosis
Artificial neural networks can assess and detect radiographic sacroiliitis, helping physicians to diagnose and classify axial spondyloarthritis, according to data presented at the American College of Rheumatology 2020 Annual Meeting.
“Assessment of radiographs of sacroiliac joints is important for both diagnosis and classification in axial spondyloarthritis,” Denis Poddubnyy, MD, of the Charité Universitätsmedizin, in Berlin, told Healio Rheumatology. “There is, however, a large inter-reader variability of the radiographic sacroiliitis assessment that might result into a wrong diagnosis and improper classification of patients.”
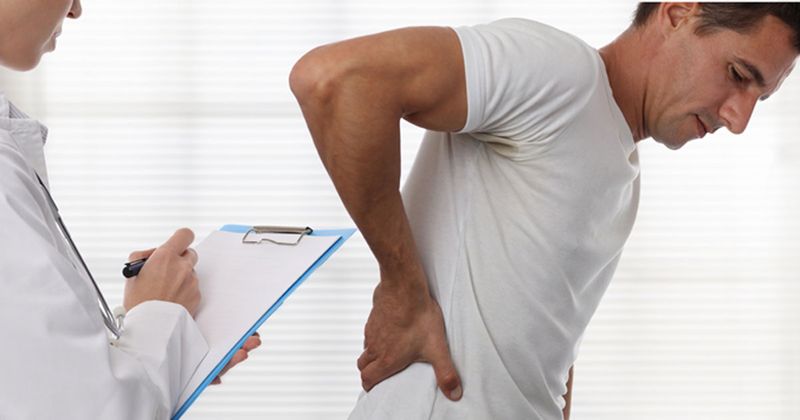
To develop an artificial intelligence-based aid for recognizing definite radiographic sacroiliitis, as a manifestation of axial SpA, Poddubnyy and colleagues used a dataset of nearly 2,000 radiographs of sacroiliac joints from two independent cohorts. The first cohort, PROOF, included 1,669 radiographs and was used for training and validating the neural network. The second cohort consisted of 100 randomly selected radiographs from GESPIC and acted an independent test dataset.
All radiographs in both cohorts underwent central reading. The researchers used the radiographic criterion of the modified New York criteria as a reference when making final decisions on the presence or absence of definite radiographic sacroiliitis. To evaluate the neural network’s performance, they calculated areas under the receiver operating characteristic curves (AUROC). Sensitivity and specificity for the prediction cut-offs were also determined. Lastly, Poddubnyy and colleagues assessed agreement between the neural network and the human readers using Cohen’s Kappa and absolute agreement.
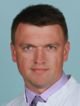
According to the researchers, the neural network demonstrated excellent performance in recognizing definite radiographic sacroiliitis, with an AUROC of 0.97 for the validation dataset and 0.96 for test dataset. Meanwhile, sensitivity and specificity for the cut-off weighting both measurements equally were 0.9 and 0.93, respectively, for the validation set, and 0.87 and 0.97, respectively, for the test set. The Cohen’s kappa between the neural network and the reference judgements were 0.8 for both the validation and test data sets. In addition, the absolute agreement on the classification yielded 91% and 90%, respectively.
“Our work shows that the artificial intelligence approach, using a trained neural network, can help in deciding on complex imaging that would be comparable with — and most likely even better than — the performance of a trained and experienced central reader,” Poddubnyy said. “The trained neural network is able to assess radiographs of sacroiliac joints in a standardized and consistent way that might be helpful in decision making in diagnostic and classification processes.”