EHR data helps predict COPD readmissions
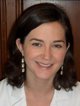
NATIONAL HARBOR, Md. — A prediction tool that exclusively used readily available electronic health record data successfully identified patients with COPD at high risk for readmission, according to preliminary data presented at Hospital Medicine 2019.
“Patients admitted for exacerbations of COPD are frequently readmitted over the following 1 to 3 months,” Valerie G. Press, MD, MPH, assistant professor of medicine and pediatrics and executive medical director of specialty value-based care at the University of Chicago, told Healio Primary Care Today. “In fact, initial COPD exacerbations requiring admissions are the third leading cause of 30-day readmissions. Identifying patients at high risk for readmission while they are hospitalized allows for enhanced opportunities to provide patients with care and resources they need during and after their hospital admission to reduce their readmission risk.”
“Current readmission risk scores were not developed for use in ‘real-time,’ meaning they require data available after discharge and did not use machine learning methods, which our team has found to produce prediction models with increased accuracy over the more standard logistic regression-based models,” she added. “Further, it is not clear whether COPD-specific tools would be superior to general readmission risk scores.”
Press and colleagues sought to create a COPD-specific risk prediction tool using real-time EHR data to help identify patients with COPD at high risk for readmission.
The researchers included 1,328 patients who had a primary or secondary ICD-9-CM diagnosis code of COPD or were treated for a COPD exacerbation. The participants were randomly assigned to either the derivation set (60%), which was used to train a random forest machine learning model, or the validation set (40%), which was used to assess the performance of the random forest machine learning model using the area under the curve statistic.
A total of 306 participants had a 90-day readmission.
The researchers found that maximum creatinine value during the encounter, minimum red blood cell count and minimum body temperature were the most important predictors for 90-day readmission. The results of respiratory cultures and whether the patient was admitted during the winter months were also predictors of readmission.
The random forest machine learning model had an AUC of 0.78 in the derivation set and 0.74 in the validation set at the 90-day prediction horizon.
“Since numerous risk prediction tools exist, it is critical that any new tool provide advances over existing tools,” Press said. “To be useful for improving care for patients, the tools need to be highly accurate across diverse patient populations and need to be useful to the direct care providers using the tools.”
“Our data show that we can use data available in the EHR to predict 90-day readmissions with similar or even increased accuracy over current general 30-day readmission risk tools and COPD-specific 90-day readmission risk tools that include data not always readily available in the electronic health record,” she added. “Further studies are needed to determine whether our methods can further improve accuracy for COPD-specific 30-day readmission risk, feasibility of wide-scale implementation of the tool into everyday practice, and whether our prediction tool generalizes to diverse patient populations.” – by Alaina Tedesco
Reference:
Hemmrich M, et al. Deprivation and validation of a COPD readmission risk prediction tool. Presented at: Hospital Medicine 2019. March 25-27, 2019; National Harbor, Md.
Disclosure: Press reports no relevant financial disclosures.