Machine-learning algorithms may identify those at risk for opioid overdose
Researchers have found machine-learning algorithms that appear to accurately identify people at risk for opioid overdose, according to findings recently published in JAMA Network Open.
High-risk criteria are used to determine which Medicare beneficiaries are selected into the drug management programs established by the Comprehensive Addiction and Recovery Act that will soon be required for all Part D plans, according to researchers.
“Current measures of high [opioid overdose] risk were derived from studies that used traditional statistical methods to identify risk factors for overdose rather than predict an individual’s risk. However, individual risk factors may not be strong predictors of overdose risk. Moreover, traditional statistical approaches have limited ability to handle nonlinear risk prediction and complex interactions among predictors,” Wei-Hsuan Lo-Ciganic, PhD, of the department of pharmaceutical outcomes & policy at the College of Pharmacy at the University of Florida and colleagues wrote.
“Machine learning is an alternative analytic approach to handling complex interactions in large data, discovering hidden patterns, and generating actionable predictions in clinical settings. In many cases, machine learning is superior to traditional statistical techniques ... but to our knowledge, has not yet been applied to address the opioid epidemic,” they added.
The researchers identified 268 potential opioid overdose risk predictors and measured them in 3-month windows among 560,057 fee-for-service Medicare beneficiaries without cancer who filled more than one opioid prescription from Jan. 1, 2011, to Dec. 31, 2015. The mean age of these patients was 68 years, 82% were white, approximately 63% were women, 41% were dual eligible, 35% were disabled, and 0.6% had at least one overdose.
Lo-Ciganic and colleagues that found in the validation sample of patients, the deep neural network (C statistic = 0.91; 95% CI, 0.88-0.93) algorithms outperformed the other models at predicting opioid overdose. At optimized sensitivity and specificity, the deep neural network had sensitivity of 92.3%, specificity of 75.7%, number needed to evaluate of 542, positive predictive value of 0.18% and negative predictive value of 99.9%.
In addition, the gradient boosting machine model performed well (C statistic = 0.9; 95% CI, 0.87-0.94) and at optimized sensitivity and specificity, had a sensitivity of 86.8%, specificity of 81.1%, number needed to evaluate of 447, positive predictive value of 0.22%, and negative predictive value of 99.9%.
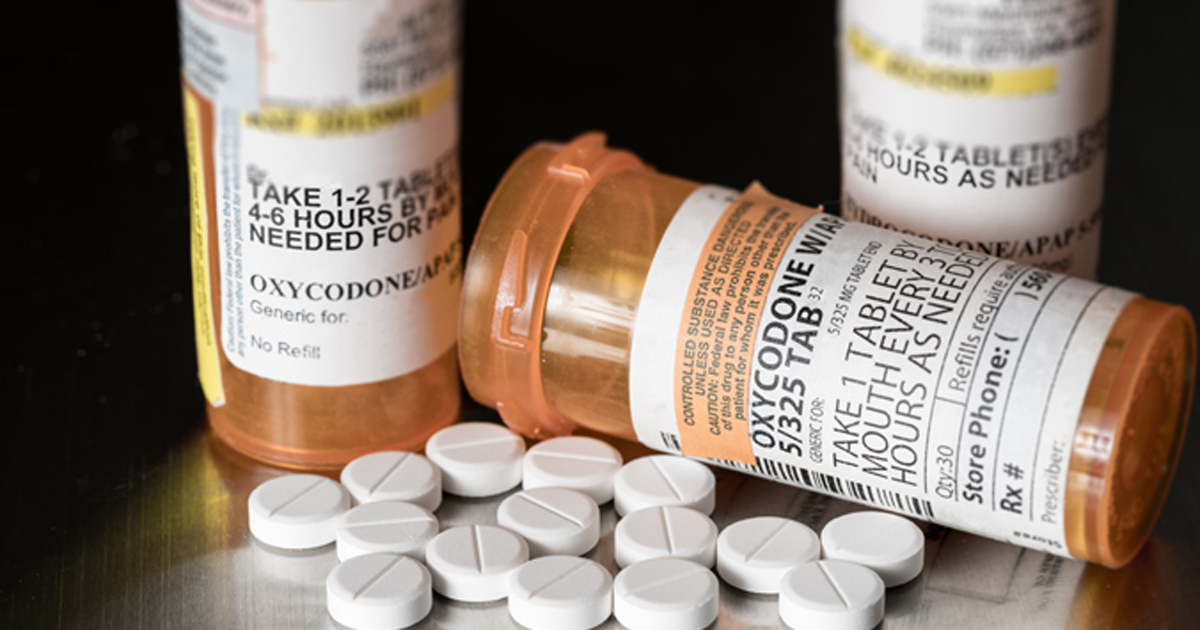
“As expected in a population with very low prevalence of the outcome, the [positive predictive value] of the models was low; however, these algorithms effectively segmented the population into three risk groups according to predicted risk score, with three-quarters of the sample in a low-risk group with a negligible overdose rate and more than 90% of individuals with overdose captured in the high- and medium-risk groups,” Lo-Ciganic and colleagues wrote.
“The ability to identify such risk groups has important potential for policymakers and payers who currently target interventions based on less accurate measures to identify patients at high risk,” they added. – by Janel Miller
Disclosures : Lo-Ciganic reports receiving grants from the NIH, National Institute on Drug Abuse, and the Pharmaceutical Research and Manufacturers of America Foundation during the course of the study. Please see the study for all other relevant financial disclosures.