Clinical trials for rare diseases need new, specific methodological approaches
ROME — The total estimated costs from inherited retinal diseases in the United States amounts to $31.8 billion. And yet, research barriers slow down the progress toward innovative treatment pathways, such as gene and cell therapies.
“Rare disease is costly and is difficult to get into. But there is an immeasurable benefit to cure ... that we must continue to strive for,” Suber S. Huang, MD, MBA, said at the FLORetina-ICOOR meeting.
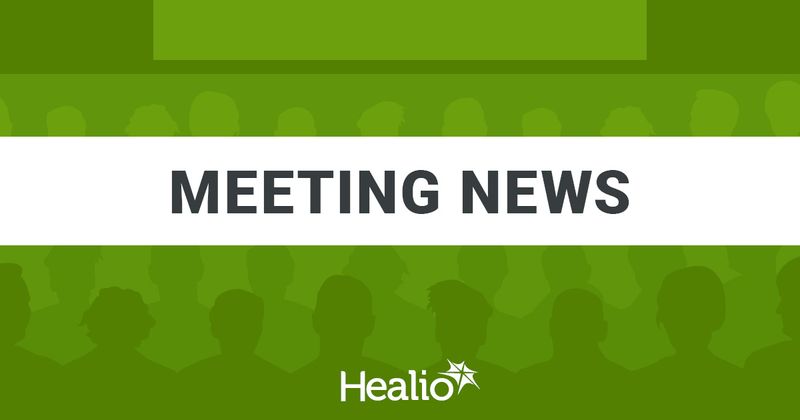
Clinical trials for rare diseases are challenging to implement. The treatments are expensive, with limited return on investments and a large financial risk for the sponsors. The patient populations are small, dispersed and difficult to recruit.
“Treatment-naive patients with [inherited retinal diseases] are rare, and enrollment in one gene therapy may have the unfortunate consequence of barring participation in a future therapy. So, recruitment is actually very difficult,” Huang said.
Regulatory authorities, due to inconsistent and evolving regulatory requirements, often stand in the way, leading to the failure of trials evaluating “perfectly reasonable alternatives for treatment,” Huang said. New pathways and disease-specific methodological approaches are therefore needed.
Huang is the founder and chair of the Future Vision Forum, a collaborative multidisciplinary meeting involving ophthalmology, visual science industry, advocacy and regulatory stakeholders. In September, rare diseases were discussed, and five recommendations were issued to improve the efficiency of clinical trials.
“The first is to develop clear standards for de-risking drug-, gene- and cell-based approaches to ocular therapeutics. We should all have the same playbook. No. 2, we should define a pathway by which unpublished, failed or non-statistically significant studies can contribute to a universal knowledge base for natural history or why things fail,” he said.
The third and fourth recommendations were related to the use of artificial intelligence and machine learning to aggregate and mine deidentified data for hypothesis and adverse event signals, to identify and screen patients, and to model future therapies.
“Let’s make sure that people could actually respond to therapy. AI could help with that. And lastly, we need to consider therapies for a spectrum of needs rather than a gene-specific model,” he said.