SLIViT algorithm detects biomarkers of progression in non-neovascular AMD
ROME — A deep learning algorithm developed at University of California, Los Angeles, showed superior performance compared with other algorithms and human graders in detecting early biomarkers of age-related macular degeneration progression.
“It is important to define and detect early biomarkers for AMD progression because we want to develop drugs targeting earlier stages of non-neovascular AMD,” Giulia Corradetti, MD, said at the FLORetina-ICOOR meeting.
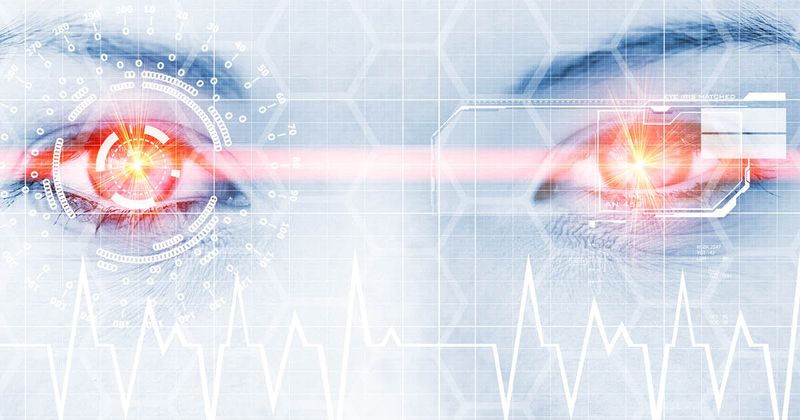
Image: Adobe Stock
SLIViT, short for Slice Integration by Vision Transformers, is a deep learning framework able to measure disease-related risk factors in various volumetric medical imaging modalities, such as MRI, OCT and ultrasound.
“The input is 3D volumetric data. And then this 3D volumetric data is split into 2D images,” Corradetti said. “We use an aggregator to get the information from each image, and then this information is aggregated to generate the output. We use three different data sets, the first one for training, validation and testing and other two independent data sets just for testing.”
In the setting of non-neovascular AMD, SLIViT was evaluated for the ability to detect high-risk biomarkers for AMD progression, including high central drusen volume, drusen with hyporeflective core, intraretinal hyperreflective foci and subretinal drusenoid deposits.
When compared with other deep learning algorithms, SLIViT showed greater performance in the identification of each biomarker. When compared with seven human graders, it performed equally well but was significantly faster.
“In conclusion, SLIViT can improve health care by reducing the cost of the time-consuming process of image annotation, and the advantage is that we can leverage transfer learning. Basically, the pretraining can be done on a large data set using a different domain while the fine-tuning can be performed using a smaller data set in the domain of interest. For example, we used for training less than 700 cases,” Corradetti said.
Identifying the subset of population with high risk for developing late AMD is important for recruitment in clinical trials, she said. In addition, it can be employed in underserved populations, increasing diversity in recruitment for clinical trials.