Monitoring algorithm predicts association of seizure burden with functional outcomes
Key takeaways:
- The study analyzed 334 cases of point-of-care EEGs in the SAFER-EEG clinical trial.
- Overall, 79% of those with a seizure burden of at least 50% were discharged to a long-term care facility.
DENVER – A machine-learning seizure burden monitoring algorithm was able to predict the level of poor functional outcomes in those with neurological conditions, according to new research.
“Several decades of research highlighted the significant correlation between seizure burden and unfavorable outcomes of patients with various neurological conditions,” according to Masoom J. Desai, MD, FACNS, neurophysiologist and assistant professor of neurology at the University of New Mexico Health Sciences Center, in an AAN-sponsored press conference held prior to the meeting. “Our study addresses the critical need for automation in monitoring epileptiform activity and seizure burden.”
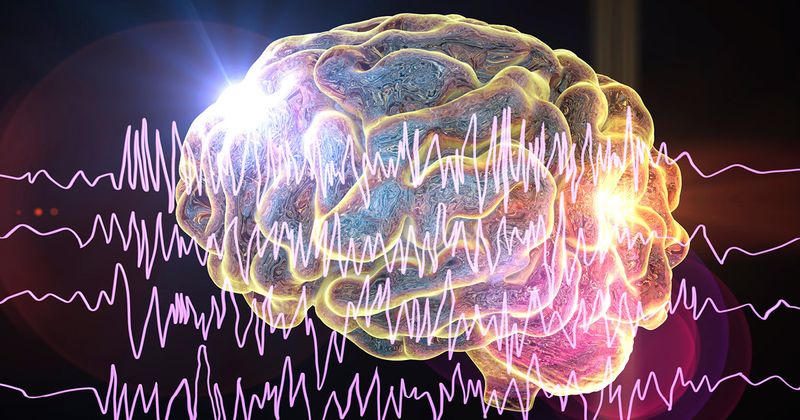
Desai and colleagues sought to determine the association of seizure burden (SzB), estimated via an automated machine-learning algorithm, Clarity, with functional outcomes in neurological disorders including epilepsy. They hypothesized that higher SzB will be associated with poor outcomes, as measured by the modified Rankin scale (mRS) score, as well as discharge to a long-term care facility (LTCF).
They performed a subanalysis on data collected from the retrospective SAFER-EEG trial, on 334 cases from point-of-care electroencephalogram (POC-EEG) cohorts. Clarity was run post-hoc in the POC-EEGs and outputted the maximum 5-minute SzB per hour, in nonoverlapping bins. Researchers additionally analyzed outcomes in patients with a maximum SzB of at least 50% toward the end of the recording, including those with a suspected status epilepticus alarm (SzB of at least 90%). A total of 178 (52%) cases posted a SzB of zero throughout the recording, and 41 (12%) had suspected status epilepticus.
Researchers found that patients with a max SzB of at least 50% in the last quarter of the recording (n = 31) were more likely to have unfavorable mRS outcomes compared with those without seizure activity (77% vs. 57%).
In the subanalysis of those status epilepticus alarms (n = 17), data showed that a higher proportion of patients with SzB of at least 50% toward the end of the recording (n = 24) were discharged to LTCF (79% vs. 54%)
“The implications of our research are profound, indicating a shift toward integrating AI and machine learning-guided interpretation in management of critically ill patients with seizures,” Desai noted.