Machine learning model able to better predict AD from driving data, biomarkers
Key takeaways:
- The study included 139 adults aged 65 years and older to drive vehicles for 1 year.
- When a machine learning model was applied, more variables meant greater prediction accuracy for preclinical Alzheimer’s.
PHILADELPHIA — A machine learning model was better able to predict preclinical Alzheimer’s disease from participant biomarkers as well as driving data from a year-long study, according to a speaker.
“As we age, there are declines in sensory and motor abilities and when we think about driving, it really is one of the most complex activities that most of us do,” Ganesh M. Babulal, PhD, OTD, MSCI, an associate professor in the department of neurology at Washington University School of Medicine, said during his presentation at the American Neurological Association annual meeting. “It requires sustained and dynamic engagement.”
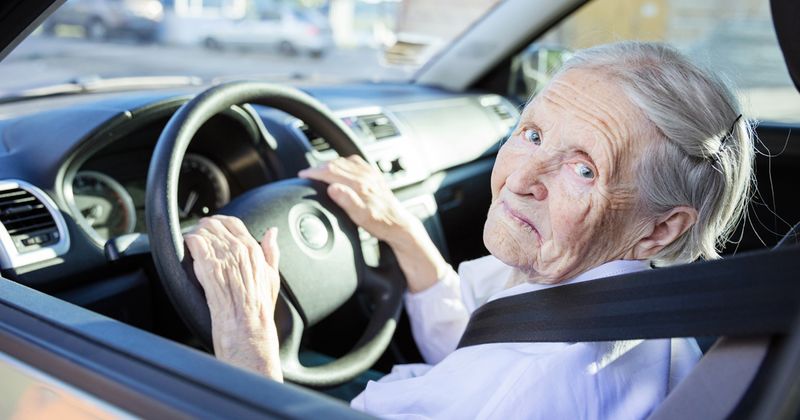
Babulal and colleagues sought to identify older drivers at risk of cognitive decline and at risk for accidents and crashes, and to do so before cognitive decline occurs.
In the first 4 years of their research, the researchers found those with preclinical AD as measured by positive readings on amyloid or cerebrospinal fluid (CSF) biomarker testing made 2.5 more errors on a standard road test and were faster to fail a road test although remaining cognitively normal.
Their current study involved the DRIVES program, in which each 139 adults aged 65 years and older who were deemed cognitively normal as measured by the Clinical Dementia Rating scale, required to drive a non-adaptive vehicle with a valid driver’s license at least once per week.
Each participant had their vehicle fitted with a chip that for 1 year measured latitude and longitude for each vehicle as well as the number of trips, miles, unique destinations along with speed, the number of instances of hard braking and sudden acceleration. An accelerometer also measured the kind of accident impact as well as level of accident impact as either minor or major. All participants with CSF data were categorized as positive or negative and logistic regression models were employed to measure area under the curve. A machine learning model was applied with data to further analyze AUC with respect to biomarkers predicting preclinical disease.
According to results, when more variables were added to analysis, the more closely the AUC approached 1, indicating greater accuracy for preclinical disease prediction. When driving, baseline age, APOE, e4 status, race and gender were factored, AUC reached 0.963, compared with 0.774 when only driving was analyzed.
“Plasma biomarkers have really been the holy grail for Alzheimer’s disease,” Babulal said. “These are data that’s easily captured on any patient.”