Risks for bias high, unclear in 80% of AI models for predicting RA treatment response
Click Here to Manage Email Alerts
Key takeaways:
- Nearly 80% machine learning models for predicting RA treatment response showed high or unclear risk of bias.
- Models show “high predictive accuracies” but “inadequate” metrics to examine performance.
The use of machine learning models to predict treatment response in rheumatoid arthritis is increasing, though approximately 80% show “unclear and high risk of bias,” according to data published in Seminars in Arthritis and Rheumatism.
“Machine learning (ML) is a combination of algorithms exploring how computer systems can learn rules from multiple examples without explicit programming,” Huaiya Xie, of the department of pulmonary and critical care medicine at Peking Union Medical College Hospital, in Beijing, China, and colleagues wrote. “The computer gradually improves its performance at a task by learning from increasing amounts of data. ... In recent decades, ML applications in health care have expanded to include the prediction of RA treatment response.”
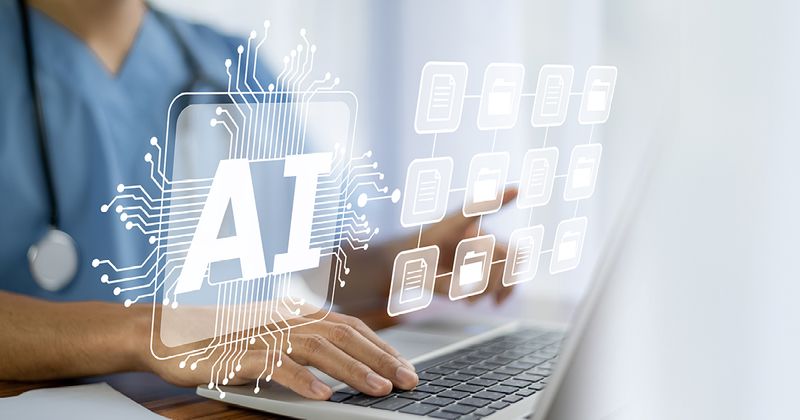
To outline the current status — and performance — of machine learning for that purpose, Xie and colleagues conducted a systematic review of 29 studies that “derived and/or validated” machine learning models for RA treatment response. Each study’s risk for bias, as well as concerns about model applicability, was assessed using the Prediction Model Risk of Bias Assessment Tool (PROBAST). The studies were also “critically appraised” for their adherence to the Transparent Reporting of a Multivariable Prediction Model for Individual Prognosis or Diagnosis (TRIPOD) guidelines, the researchers wrote. They then conducted a narrative synthesis of the findings.
Ten of the included studies involved the development of a predictive model, with a mean adherence to TRIPOD guidelines of 45.6% (95% CI, 38.3-52.8), according to the researchers. The other 19 studies, which developed models and validated them externally, showed mean TRIPOD adherence of 42.9% (95% CI, 39.1-46.6).
The PROBAST results showed that 41.4% of the articles had “unclear” risk of bias while 37.9% demonstrated “high” risk. Six articles (20.7%) demonstrated “low” bias risk.
“The application of ML algorithms to RA treatment response has expanded, with ML model evaluations reporting high predictive accuracies but inadequate essential metrics and attributes to examine model performance,” Xie and colleagues wrote. “To improve the generalizability, standardization, reproducibility and reporting quality essential for clinical practice, future ML reports will require training and testing on large, multicentric datasets, more validation on external datasets, complete predictive performance metrics and adherence to reporting recommendations.”