AI model predicts joint damage in RA; only meant to complement, ‘not replace the human’
SAN DIEGO — An automated deep learning model accurately identified and predicted joint space narrowing and erosion based on hand radiographs of patients with rheumatoid arthritis, according to data presented at ACR Convergence 2023.
“Radiographic damage is one of the core outcome measures for rheumatoid arthritis, and as clinicians, we usually do serial plain X-rays so we can follow and detect joint damage in a patient over time, and that helps influence our management decisions,” Carol Hitchon, MD, FRCPC, MSc, an associate professor at the University of Manitoba, said during a press conference. “One of the more commonly used metrics used for evaluating joint damage, [the Sharp-Van der Heijde score] tells us how much joint space narrowing there is and how much joint erosions there are — but it is not really feasible in clinical practice.
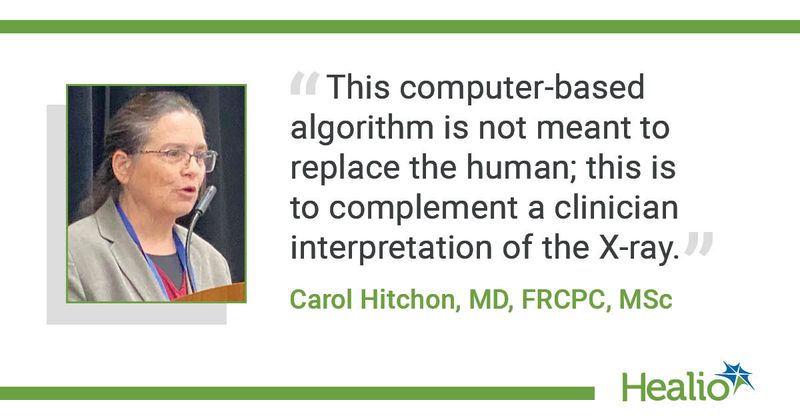
“It takes a lot of time to score these joints using these scores,” she added. “You need a fair amount of expertise to do it, because there is a lot of intra- and inter-observer variability, and, oftentimes, the expertise to actually do these scores and evaluate radiographs is not available in many centers.”
To develop a deep learning system that would automatically detect joints and predict Sharp-Van der Heijde scores in patients with RA based on hand X-rays, Hitchon and colleagues first trained a convolutional neural network-based algorithm to detect joints in 240 training and 89 test pediatric hand X-rays from the Radiologic Society of North America database.
“What we wanted to do was develop a model in which the computer can look at an X-ray, find a joint, tell us which joint it is, and then having done that, tell us if that joint is damaged and what is the score for erosions and the score for joint space narrowing,” Hitchon said.
Throughout training, the researchers boxed and labeled the numerous joints of interest for this patient group, including the proximal interphalangeal, metacarpophalangeal, wrist, distal radius and distal ulna. The joint detection model was validated with 54 clinician-annotated radiographs from four adult patients with RA who had been followed for more than 10 years, with approximately 10 to 12 images for each patient.
Hitchon and colleagues then applied a vision transformer model to forecast the erosion and joint space narrowing score for each joint. This model was validated using more than 2,200 hand X-rays from 381 patients with RA who had physician-assigned Sharp-Van der Heijde scores.
According to results, the deep learning model was able to accurately identify the target joints, with a pediatric data F1 score of 0.991 and adult data F1 score of 0.812. Predictions from the vision transformer model for erosion and joint space narrowing were likewise highly accurate, with a root main squared error of 0.93 and 0.91, respectively.
“When we compared what the computer predicted as a joint space narrowing score, or an erosion score, to what our expert clinicians said was a joint space narrowing score or erosion score, they were very similar and very accurate,” Hitchon said. “We feel that this is a very significant advance, because it could enable clinicians working in areas without radiographic expertise to evaluate how much joint damage a patient has and how it changes over time.”
Hitchon emphasized that this deep learning model may also have applications in larger studies, such as randomized clinical trials or drug trials with large cohorts in which the outcome measure is joint damage.
“If there are lots of X-rays that need to be scored, this is a much more feasible way of doing it,” she said.
However, Hitchon cautioned that, at this early stage, the model still falls short of human radiologists in detecting certain joints, particularly the wrist.
“This computer-based algorithm is not meant to replace the human; this is to complement a clinician interpretation of the X-ray,” she said. “Down the road, we may be able to have things that the computer can see that our human eyes can’t see that could give us more insights into who, at baseline, will have a lot of erosions and who will not.”