Models may predict chronic opioid use in patients with osteoarthritis
Click Here to Manage Email Alerts
Models based on electronic health records may estimate the risk for chronic opioid use in patients with osteoarthritis, according to data published in Arthritis Care & Research.
“We had interest in doing something to address outcomes in patients with osteoarthritis, in part because it is so widespread and challenging to manage that it creates a major burden for our members,” Jeff T. Mohl, PhD, of the American Medical Group Association, in Alexandria, Virginia, told Healio. “Eventually we settled on creating a predictive model to help avoid chronic opioid use — another important public health issue — because ultimately the management of pain for patients with OA is one of the most pressing and complex challenges.
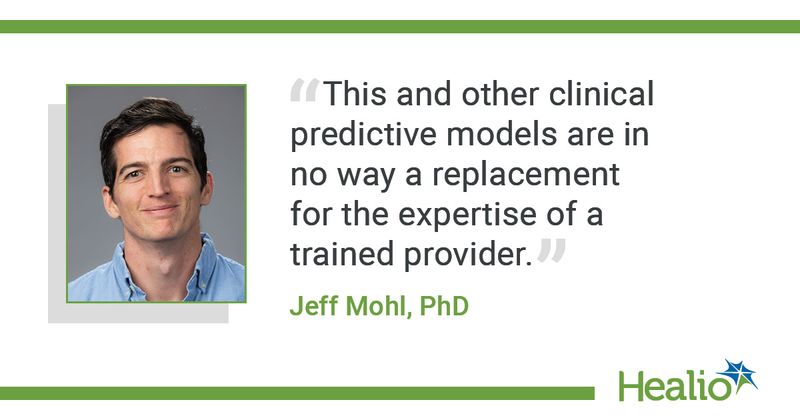
“This allowed us to leverage our dataset to provide personalized risk assessments that could provide information to help support these tricky decisions between the provider and the patient,” he added.
To test a model’s ability to predict the likelihood that patients with OA might begin chronic opioid use, Mohl and colleagues extracted EHR data from 13 diverse health centers. The analysis included patients aged 18 to 89 years who had at least one opioid prescription between March 1, 2017, and Feb. 28, 2019, and no record of opioid use within the previous 6 months. Patients were also required to have been diagnosed with OA before the date the prescription was given. Individuals were excluded if they had no EHR activity in the previous 12 months.
The primary outcome was the development of chronic opioid use, defined as one or more periods spanning more than 84 days in which 3 prescriptions were filled, within 12 months of the incident prescription date. Prescriptions were excluded if they were prescribed within 3 days of an inpatient visit, observational stay or surgical procedure.
The researchers authors developed four models to estimate the risk for chronic opioid use. These models were assessed using the area under the receiving operating characteristic curve (AUC) to compare each model’s data discrimination ability.
The study population included 33,894 patients with OA, of whom 2,925 developed chronic opioid use within 1 year of the incident prescription. The best-performing model, called “random forest,” achieved an AUC of 0.728 (95% CI, 0.711-0.745). Meanwhile, a regression model achieved an AUC of 0.717 (95% CI, 0.699-0.734). Models demonstrated “highly variable discrimination” across different organization datasets (AUC range = 0.571-0.842).
Mohl and colleagues noted that “care should be taken” when extending the model into communities it was not trained on.
“The main takeaway is that from the perspective of risk from opioids, all patients are unique,” Mohl said. “We found almost a 10-fold difference in risk between the top and bottom 10% of patients, which might mean that it was totally reasonable to prescribe a short course of opioids to manage pain for one patient, while for another it might result in them still taking them a year later. I’d like to emphasize that this and other clinical predictive models are in no way a replacement for the expertise of a trained provider.”