Artificial intelligence review of physician notes discerns types of lower back pain
Researchers from the Icahn School of Medicine at Mount Sinai developed an artificial intelligence model that can scan physicians’ notes and distinguish between acute and chronic lower back pain, according to findings published in the Journal of Medical Internet Research.
“Several studies have documented increases in medication prescriptions and visits to physicians, physical therapists, and chiropractors for lower back pain episodes,” Ismail Nabeel, MD, MPH, associate professor of environmental medicine and public health at the Icahn School of Medicine at Mount Sinai, said in a press release. “This study is important because artificial intelligence can potentially more accurately distinguish whether the pain is acute or chronic, which would determine whether a patient should return to normal activities quickly or rest and schedule follow-up visits with a physician.”
“This study also has implications for diagnosis, treatment and billing purposes in other musculoskeletal conditions, such as the knee, elbow, and shoulder pain, where the medical codes also do not differentiate by pain level and acuity,” he added.
To examine the feasibility of a system that automatically distinguishes acute lower back pain based on free-text clinical notes, Nabeel and colleagues used a dataset of 17,409 clinical notes from various primary care practices in the Mount Sinai Health System. Of these clinical notes, 891 documents were manually annotated as acute lower back pain while 2,973 were generally associated with lower back pain through the recorded ICD-10 code.
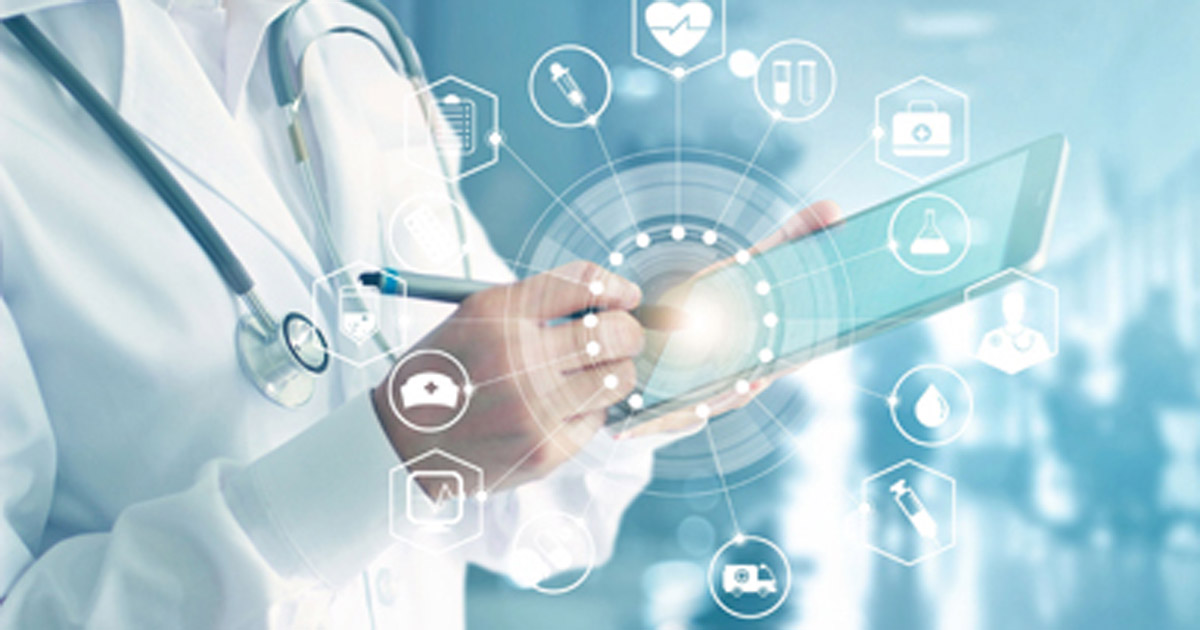
The researchers compared various supervised and unsupervised strategies for automated identification, including keyword search, topic modeling, logistic regression with bag of n-grams and manual features, and deep learning — a convolutional neural network-based architecture, referred to as ConvNet. Nabeel and colleagues then trained the supervised models using either manual annotations or ICD-10 codes as positive labels.
According to the researchers, ConvNet trained using manual annotations obtained the best results with an area under the receiver operating characteristic curve of 0.98, and an F score of 0.7. ConvNet’s results demonstrated robustness to reduction of the number of manually annotated documents. Additionally, in the absence of manual annotations, topic models performed better than methods trained using ICD-10 codes, which were unsatisfactory for distinguishing lower back pain types.
“This study demonstrates the feasibility of using machine learning to automatically identify acute LBP episodes from clinical reports using only unstructured free-text data,” Nabeel and colleagues wrote. “In particular, manually annotating a set of notes to use as a gold standard can lead to effective results, especially when using deep learning. Topic modeling can help in speeding up the annotation process, initiating an iterative process where initial predictions are validated and then used to refine and optimize the model.”
“This approach provides a generalizable framework for learning to differentiate disease acuity in primary care, which can more accurately and specifically guide the diagnosis and treatment of LBP,” they added. “It also provides a clear path toward improving the accuracy of coding and billing of clinical encounters for LBP.” – by Jason Laday
Disclosures: The researchers report no relevant financial disclosures.