AI model correctly predicts FVC, FEV1 from chest X-rays
Key takeaways:
- AI model lung function estimations agreed with spirometry.
- With the model, there were high area under the receiver operating characteristic curve values for classifying FVC and FEV1 less than 80% predicted.
With just chest X-rays, an AI model estimated FVC and FEV1 and had “excellent agreement” when compared with spirometry results, according to results published in The Lancet Digital Health.
“Highly significant is the fact that just by using the static information from chest X-rays, our method suggests the possibility of accurately estimating lung function, which is normally evaluated through tests requiring the patients to exert physical energy,” Daiju Ueda, MD, associate professor at Osaka Metropolitan University’s Graduate School of Medicine, said in a press release.
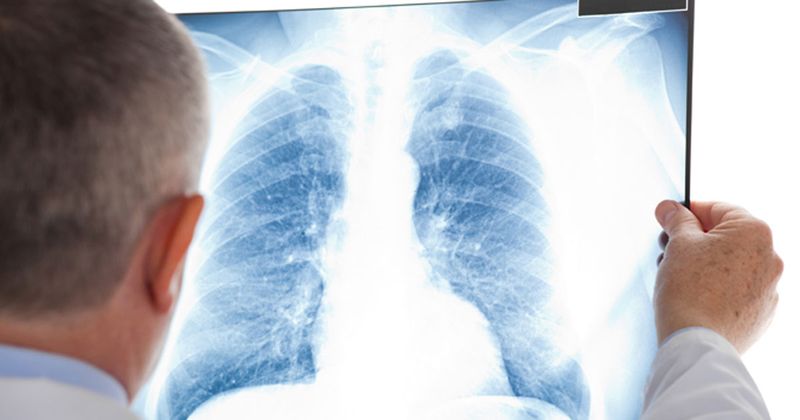
In an effort to see if a deep learning-based AI model could estimate FVC and FEV1 from chest X-rays, Ueda and colleagues trained, validated and internally tested the model using 134,307 X-ray and spirometry pairs (n = 75,768; mean age, 56 years; 50% female) from three institutions in Japan.
Researchers then externally tested their model against spirometry results using 2,137 X-ray and spirometry pairs (n = 1,861; mean age, 65 years; 40% female) from a fourth institution (institution D) and 5,290 X-ray and spirometry pairs (n = 4,273; mean age, 63 years; 46% female) from a fifth institution (institution E).
In the institution D cohort, the Pearson’s correlation coefficient for estimating FVC was 0.91, and this was similar to the Pearson’s correlation coefficient of 0.9 in the institution E cohort. These values demonstrate high agreement with spirometry results, according to the release.
When estimating FEV1, researchers observed comparable results to those above with a Pearson’s correlation coefficient value of 0.91 in both institutions.
Additionally, the AI model’s estimation of FVC had high reliability compared with spirometry in both institution D (intraclass correlation coefficient [ICC] = 0.91) and institution E (ICC = 0.89). This was also the case when estimating FEV1 (institution D and E, ICC = 0.9).
Other measures of AI model performance vs. spirometry included mean square error, root mean square error and mean absolute error.
During FVC estimation, both institution D and E had the same mean square error value (0.17 L2), root mean square error value (0.41 L) and mean absolute error value (0.31 L). During FEV1 estimation, researchers found slight differences between the two institutions in mean square error (0.13 L2; 0.11 L2), root mean square error (0.37 L; 0.33 L) and mean absolute error (0.28 L; 0.25 L).
Compared with the Pearson’s correlation coefficient values reported in the overall population, the AI model vs. spirometry results had lower Pearson’s correlation coefficient values when estimating FVC (institution D, 0.81; institution E, 0.78) and FEV1 (institution D and E, 0.83) in patients with COPD.
In a different subgroup of patients with asthma, these values were closer to those found in the overall population when estimating FVC (institution D, 0.89; institution E, 0.87) and FEV1 (institution D, 0.9; institution E, 0.87).
Based on the area under the receiver operating characteristic curve, researchers observed high values for classifying FVC less than 80% predicted with the AI model in both institution D (0.88) and E (0.85). A similar elevated area under the curve result was found for classifying FEV1 less than 80% predicted with the model in both institutions (0.87).
“Future studies should investigate the performance of this AI model in combination with clinical information, and across more diverse populations, to enable more appropriate and targeted use,” Ueda and colleagues wrote.
Reference:
- Innovative highly accurate AI model can estimate lung function just by using chest X-rays. https://www.eurekalert.org/news-releases/1050507. Published July 8, 2024. Accessed July 8, 2024.