Machine learning models may predict risk of prolonged opioid use after hand surgery
Click Here to Manage Email Alerts
Key takeaways:
- A machine learning model may identify patients’ risk for persistent opioid use after hand surgery.
- Machine learning may help physicians tailor treatment for patients at risk for addiction.
Use of machine learning models may successfully predict postoperative pain experiences and risk for prolonged opioid use among patients who undergo hand surgery, according to published results.
Natalie B. Baxter, BSE, medical student and clinical research associate at the University of Michigan Medical School, and colleagues used electronic health records and patient-reported data to train four machine learning algorithms to predict persistent opioid use among patients who underwent surgery at a single institution between 2015 and 2018.
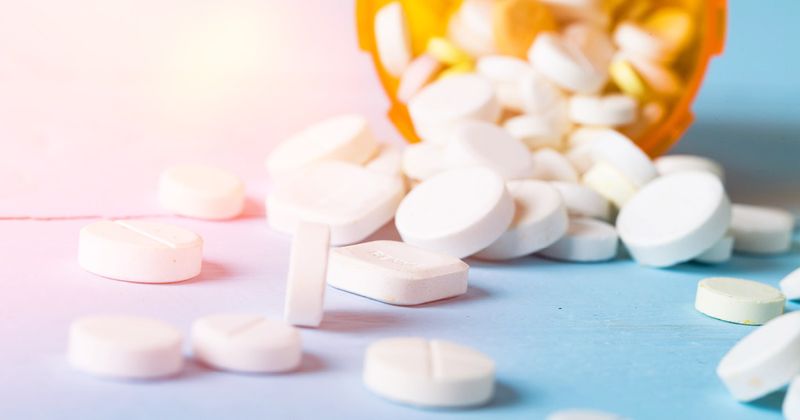
“We ultimately trained and tested four machine learning models using two preexisting, published algorithms: [Michigan Genomics Initiative] MGI model trained on full data, claims model trained on full opioid-naive data, MGI model trained on hand patients, and claims model trained on opioid-naive hand patients,” Baxter and colleagues wrote in the study.
They noted the four models were then tested using data from 889 patients who underwent hand surgeries including soft-tissue surgery (n = 493), fracture surgery (n = 175), other bone procedures (n = 31), joint replacement or fusion (n = 66), other joint procedures (n = 56) and amputation (n = 26).
Overall, Baxter and colleagues found 49% of patients were opioid-naïve. The MGI model showed 21% of patients developed persistent opioid use, and the claims model showed 10% of patients developed persistent opioid use, according to results. Researchers found the MGI model had an area under the receiver operating curve (AUC) of 0.84 when trained on data from hand surgeries and an AUC of 0.85 when trained on data from all surgeries. They found the claims model had an AUC of 0.69 when trained on data from hand surgeries and an AUC of 0.52 when trained on data from all surgeries.
For the MGI model trained on all surgeries, Baxter and colleagues found preoperative opioid prescription (53%), overall body pain in the past week (25%) and hydrocodone prescription (21%) were factors with the greatest variable of importance. For the claims model trained on hand surgeries only, they found high school diploma (31%), college education (23%), bachelor’s degree (15%) and Charlson Comorbidity Index (10%) were factors with the greatest variable of importance.
“Our study demonstrated that machine learning models can assess numerous relevant variables and provide data-driven results,” Baxter and colleagues wrote in the study. “In practice, these models could be implemented as decision-support tools to help clinicians efficiently identify patients who are most vulnerable to addiction and in need of tailored pain management or counseling.”