AI-based diagnostics ’holds promise’ in tracking symptoms for myasthenia gravis
Click Here to Manage Email Alerts
Key takeaways:
- Two observational studies compared videos of those with myasthenia gravis with healthy controls.
- A deep learning model demonstrated 76% accuracy in diagnostics and 80% accuracy in categorizing disease severity.
Artificial intelligence-based diagnostic tools may be valuable in accurately tracking myasthenia gravis symptoms and mitigating disease risk, according to a poster at the 2024 MDA Clinical & Scientific Conference.
“Artificial intelligence’s integration into medicine has revolutionized patient care, increased accessibility and transformed health outcomes,” Shaweta Khosa, MBBS, MD, a neurologist at Olive View-UCLA Medical Center, and colleagues wrote. “Recent AI-based models in neurology have enabled a multifaceted approach to evaluating myasthenia gravis.”
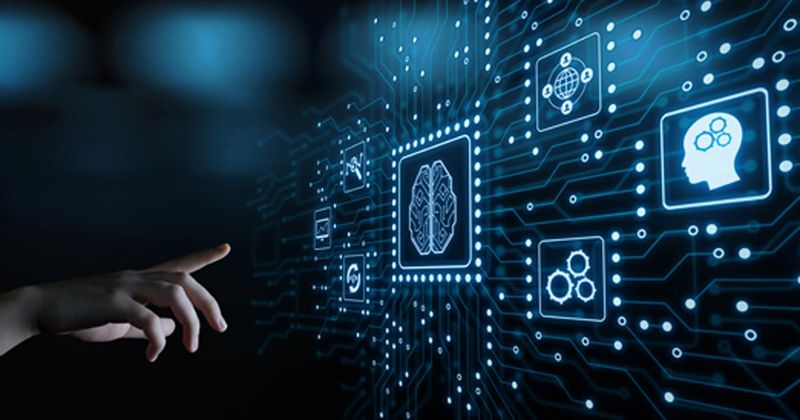
Ptosis, a common myasthenia gravis (MG) symptom, is often measured using margin-reflex distance 1 (MRD1) with a manual ruler, while newer AI-models have automated MRD1 evaluation via patient selfies on smartphones. As such, researchers sought to describe and evaluate novel AI-based tools for MG symptom management and trained a deep learning model to evaluate disease severity by quantifying facial weakness using facial recognition software.
In a 3-month prospective observational study, 82 individuals with MG produced 664 self-recorded smartphone videos taken during an eyelid fatigability exercise. Non-clinical annotators established ground truth for MRD1 and video frame quality. An artificial neural network, trained as an MRD1 measurement tool, analyzed the images.
A second observational study included video recordings of 69 healthy controls as well as 70 patients with MG. Utilizing FaceReader software, facial weakness was analyzed, quantifying six emotions. Then, a deep learning model was trained to classify MG diagnosis and severity using 50 videos from patients with MG and the same number from healthy controls.
Results showed a significant correlation between the MRD1 ground truth and predicted values.
Data further showed FaceReader findings indicated that patients with MG exhibited significantly lower expressions of anger (P = 0.026), fear (P = 0.003) and happiness (P < 0.001) compared with healthy controls. The deep learning model demonstrated 76% accuracy in diagnostic ability and 80% accuracy in categorizing disease severity.
“The emergence of AI-driven models for automated symptom assessment holds promise for precise, patient-focused tools to enhance MG management in the future,” Khosa and colleagues wrote.