Machine learning model most accurately predicts levels of cognitive impairment
Key takeaways:
- Researchers analyzed speech from 91 community-dwelling adults with symptoms of Alzheimer’s disease.
- AI-based machine learning model predicted level of cognitive impairment with 75% accuracy.
An artificial intelligence-based machine learning model that diagnosed cognitive impairment in Alzheimer’s disease extracted information more accurately than standard screening or human analysis, according to a poster presentation at CTAD.
“Timely recognition and monitoring of early cognitive decline is crucial for Alzheimer's disease clinical trials to facilitate accurate diagnosis, establish baselines, track disease progression, and measure treatment efficacy,” Catherine Diaz-Asper, PhD, associate professor of psychology at Marymount University, and colleagues wrote. “However, early cognitive decline can be difficult to diagnose and requires a comprehensive clinical examination, which is both time consuming and expensive.”
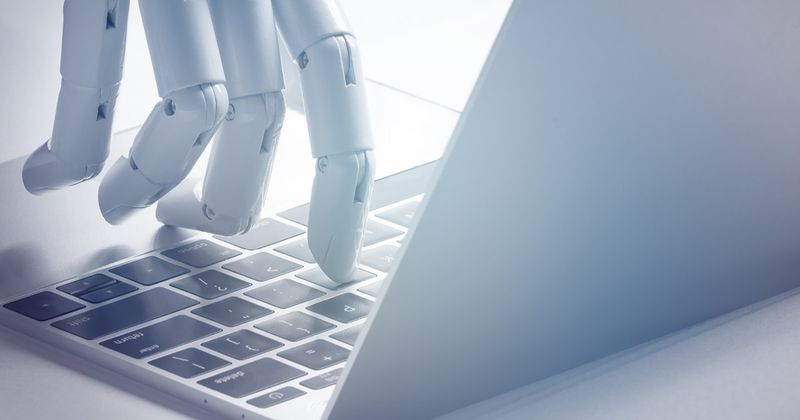
Diaz-Asper and colleagues aimed to examine effectiveness of a machine learning model, created from remote speech collection of community-dwelling adults, which may provide accurate metrics for clinical trial inclusion.
Their study included 91 native English-speaking individuals who were diagnosed as cognitively healthy (n = 29), with mild Alzheimer’s disease (n = 30) or with amnestic mild cognitive impairment (n = 32). All participants, who were evaluated using the Mini Mental State Exam (MMSE) within 6 months of inclusion, were called at their residence and completed a 20-minute interview that was digitally recorded. The researchers analyzed content based on modified Telephone Interview for Cognitive Status (TICS) criteria, as well as generating as many animal names as possible in one minute, speech patterns, autobiographical recall as well as satisfaction ratings. Additionally, language (including lexeme, syntactic and semantic) and acoustic features from the interviews were extracted to formulate a multimodal machine learning model to classify diagnostic groups.
The researchers reported that multimodal accuracy among groups were as follows: cognitively healthy vs. MCI vs. AD (75%); MCI vs. AD (79%); cognitively healthy vs. MCI (80%); cognitively healthy vs. cognitive decline (87%); cognitively healthy vs. AD (88%).
Data further showed that a machine learning model outperformed other metrics in terms of diagnostic accuracy (75%) compared with MMSE (55%), clinician analysis (49.5%) and modified TICS (45%). Additionally, 40% of clinicians expressed strong interest in adopting the AI-based telephone screening test, while an additional 33% agreed that the tool would be valuable in practice.
“Combining advances in AI with the convenience of the telephone allows for regular, accurate and unbiased screening and monitoring of early cognitive decline,” Diaz-Asper and colleagues wrote.