Customized transcription linked to more accurate speech-based AD screening
Key takeaways:
- Researchers analyzed transcriptions from 103 cognitively impaired and unimpaired individuals.
- Research is needed to assess if further sensitivity can be obtained by disfluencies and filled pauses.
A customized speech transcription model logged roughly 30% fewer errors compared with manual or automated methods and may be a more effective tool in assessing verbal Alzheimer’s disease-related impairment.
“Cognitive function and clinical status are often assessed verbally,” Caroline Skirrow, PhD, principal scientist of clinical development at Novoic Ltd. in the U.K., and colleagues wrote in a poster presentation at CTAD. “Changes in speech patterns are noted in the early stages of Alzheimer's disease and may confer additional clinical information.”
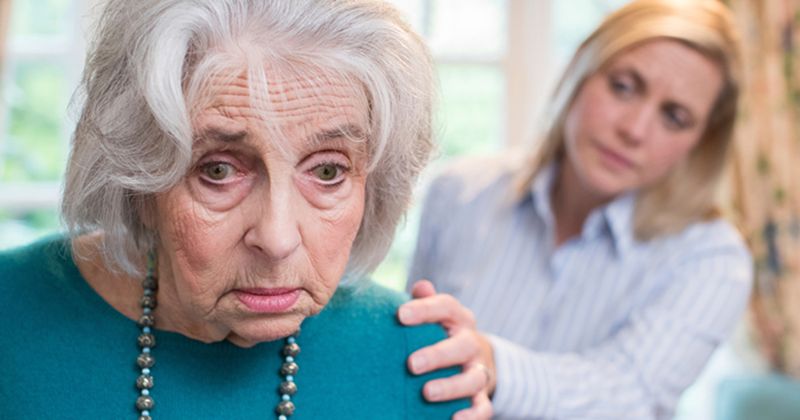
Skirrow and fellow researchers sought to evaluate a novel automated transcription system, intended to improve transcription accuracy and fidelity for better analysis of speech data related to AD assessment.
Their study included 103 adults (mild cognitive impairment/mild AD n = 47; cognitively unimpaired, n = 56) from the AMYPRED-US and AMYPRED-UK studies. All participants completed optional remote speech-based assessments for up to 8 days on their own smartphones or tablets, utilizing “Storyteller setup” which asks for immediate recall of two different stories from the Automatic Story Recall Task (ASRT) and delayed recall of the first story. Responses were transcribed by manual transcription, automated transcription via Google Speech-to-Text and a custom automated transcription multilingual encoder-decoder model.
Word Error Rate (WER) was calculated as the ratio of errors (additions + deletions + substitutions) to the number of words in the manual transcript reference, then averaged across transcriptions of ASRT task responses. The averages were calculated after removing disfluencies and filled pauses while still retaining these features.
The researchers analyzed effects of the above transcription methods on G-match, a measure of proportional recall calculated as the averaged cosine similarity between the textual embeddings of the source text and transcribed retelling for the three ASRT recalls. Prediction of MCI/mild AD from G-match was carried out with a logistic regression model and 5-fold cross-validation.
Skirrow and colleagues reported that the custom transcription method generated approximately 30% fewer transcription errors, regardless of data cleaning method, while performing better in detecting disfluencies including stutters, repetitions, false starts and filled pauses, compared with the off-the-shelf Google model.
The researchers additionally found a difference in WER between MCI/mild AD and cognitively unimpaired participants for both custom and off-the-shelf transcriptions (R = 0.27-0.35), with G-match prediction of MCI/mild AD consistent across all transcription methods (custom: AUC = 0.82; off-the-shelf: AUC = 0.81; manual: AUC = 0.82).
“Further research is now needed to evaluate if additional sensitivity may be conferred by disfluencies and filled pauses, which can be captured using this novel transcription method,” Skirrow and colleagues wrote.