Novel video imaging system classifies types of epileptic seizures
A novel 3D video imaging tool has shown high potential for the use of real-time classification of epileptic seizures in hospitals, researchers reported in Scientific Reports.
“Seizures are the defining symptom [of epilepsy], and their form is paramount for differential diagnosis and localization of the seizure onset zone in the brain,” Tamás Karácsony, MSc, a PhD candidate and machine-learning researcher at the Institute for Systems Engineering and Computers, Technology and Science in Portugal, and colleagues wrote.
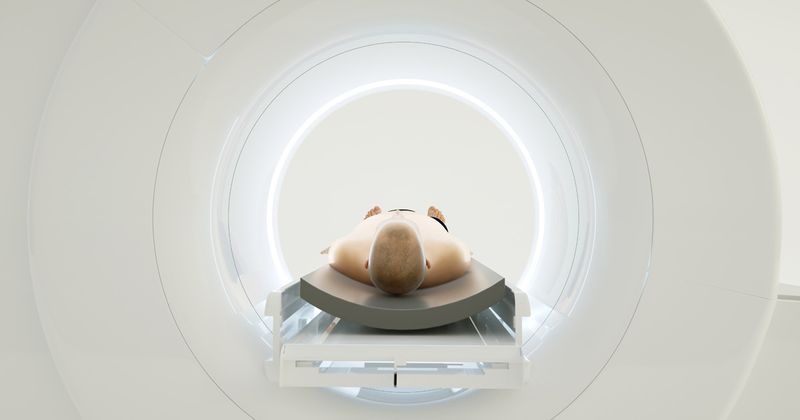
According to the researchers, seizure analysis is currently based on visual interpretation of 2D video-electroencephalogram data in epilepsy monitoring units by specialized clinicians, where semiology evaluation is limited.
Karácsony and colleagues demonstrated a novel learning-based approach for motion-based 3 class classification of seizures in frontal and temporal lobe epilepsies and a non-seizure class. Their tool uses 3D videos of seizures acquired at epilepsy monitoring units.
The authors implemented “intelligent cropping,” through the combination of Mask R-convolutional neural network video cropping and depth cropping based pre-processing, with a 96.52% and 95.65% success rate, respectively. Afterward, the authors used 3D depth cropping to remove occlusions and unrelated information from the scene, which “significantly improved” classification performance.
From there, a novel action-recognition approach was used for I3D feature extraction and long short-term memory-FC and I3D classification.
“To the best of our knowledge, [this approach] outperforms all previous deep learning-based approaches to video-based seizure classification, indicating a high potential to support physicians with diagnostic decisions,” the authors wrote. “Moreover, the research shows the feasibility of our action recognition approach to distinguish these three classes with only 2 s samples. It evaluated further temporal augmentation techniques, which suggest that larger datasets might benefit more from such augmentation, but in this case it compromises generalization, thus performance.”