Machine-learning model may predict which antiseizure medications work best
A deep-learning model has the potential to predict an individual’s response to antiseizure medications and may inform treatment selection in patients with newly diagnosed epilepsy, researchers reported in JAMA Neurology.
Haris Hakeem, MD, of the department of neuroscience at Monash University in Melbourne, Australia, and colleagues developed and validated a machine-learning model using readily available clinical information to predict treatment success of the first prescribed antiseizure medication given to patients.
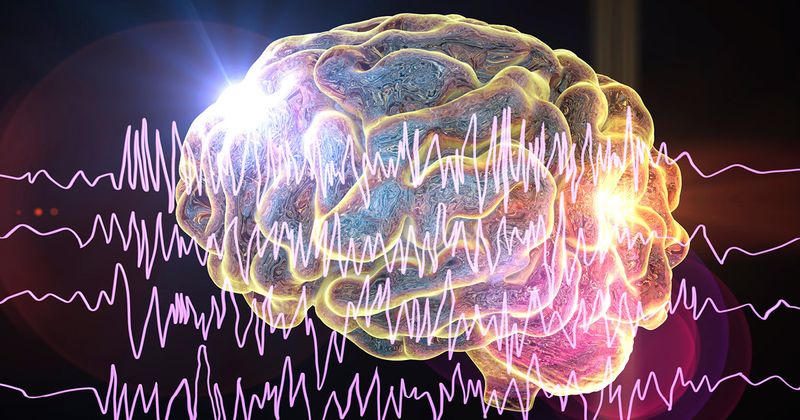
According to the authors, currently it is not possible to predict which antiseizure medication will work best, and because most are tested through a trial-and-error approach, patients may experience multiple ineffective treatments before the right drug is found.
Hakeem and colleagues used clinical data from patients treated with antiseizure medications from 1982 to 2020, all of whom were monitored for a minimum of 1 year or until failure of the first antiseizure medication. A total of 2,404 adults with epilepsy treated at five specialty centers in Scotland, Malaysia, Australia and China were considered for inclusion, with the final cohort comprising 1,798 adults (mean age, 34 years; 54.5% women).
To train and test the model, researchers used 16 clinical factors and information from seven antiseizure medications. Treatment success was defined as complete seizure freedom for the first year of treatment while taking the first medication.
Researchers included five independent cohorts and initially pooled all cohorts for model training and testing. The model was trained a second time using the largest cohort and externally validated on the other four cohorts. Researchers compared the performance of their deep-learning model, known as the transformer model, with other machine-learning models.
The transformer model that was trained with the entire pooled cohort had an area under the receiver operating characteristic curve (AUROC) of 0.65 (95% CI, 0.63-0.67) and a weighted balance accuracy of 0.62 (95% CI, 0.60-0.64) on the test set. The AUROCs of the model that was trained using the largest cohort ranged from 0.52 to 0.60, with weighted balanced accuracy of 0.51 to 0.62 in the external validation cohorts. The transformer model that was developed using the pooled cohort performed better than two of the other five models that were tested.
“Although its current performance is modest, our model serves as a foundation to further improve clinical applicability to and comparison with expert consensus decision-making algorithms,” the authors wrote. “Because our model may predict an individual’s response to a range of [anti-seizure medications], it can potentially be used as a clinical decision support tool to inform drug selection.”
In a related editorial, Sharon Chiang, MD, PhD, and Vikram R. Rao, MD, PhD, both of the Weill Institute for Neurosciences at the University of California, San Francisco, noted the study provides “useful appraisal,” but still questioned whether the model “exceeds the intuition of experienced clinicians.”
“The fact that two-thirds of people with epilepsy currently achieve seizure control with pharmacotherapy using clinician experience alone suggests that additional improvements will be needed before [machine-learning] methods for personalized prediction of [antiseizure medication] response are considered ready for primetime clinical practice,” they wrote.
Reference:
Chiang S, et al. JAMA Neurol. 2022;doi:10.1001/jamaneurol.2022.2441.