AI-enabled video of skin on face, hands may detect high blood pressure, diabetes
Click Here to Manage Email Alerts
Key takeaways:
- A non-contact, AI-enabled test successfully used biometrics to detect hypertension and diabetes.
- The approach has potential to improve health care access and disease prevention.
CHICAGO — An AI-enabled tool utilizing machine learning, high-speed images and video accurately detected hypertension and type 2 diabetes among Japanese adults compared with continuous BP monitoring and HbA1c testing, researchers reported.
The AI-powered algorithm, which analyzes video of skin on the face and hands to detect high BP and type 2 diabetes, could offer a noninvasive method to test for two highly prevalent diseases, researchers wrote in an abstract presented at the American Heart Association Scientific Sessions.
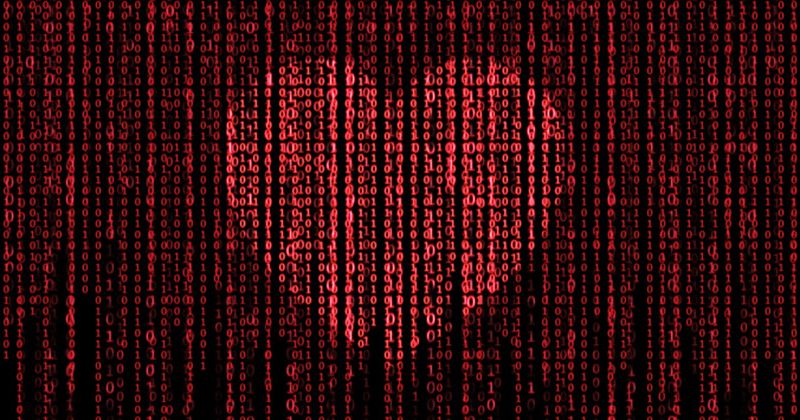
“Our research shows that it may be possible to detect hypertension and diabetes in the near future in a non-contact, noninvasive manner without going to the hospital,” Ryoko Uchida, BCs (Pharm), a project researcher in the department of advanced cardiology at the University of Tokyo, Japan, told Healio. “If lifestyle-related diseases such as hypertension and diabetes can be detected by non-contact devices without a medical visit, it could lead to early detection and early treatment of diseases in people who are indifferent to their health. This could also lead to the prevention of serious diseases and the reduction of medical costs. Furthermore, being non-contact could greatly reduce the risk for infection for both medical staff and patients in the case of a pandemic such as COVID-19.”
For the prospective study, researchers analyzed data from 200 adults who consented to have images taken of their faces and hands with a high-speed camera at a rate of 150 images per second, with BP measurements taken simultaneously for correlation. A machine learning algorithm analyzed skin blood flow captured via video and spectral features from the images to identify potential diseases, with a focus on type 2 diabetes and hypertension. Researchers defined hypertension according to Japanese Society of Hypertension guidelines — systolic BP greater than 115 mm Hg or diastolic BP greater than 75 mm Hg. Diabetes was defined as an HbA1c of 6.5% or higher or a previous diagnosis.
Researchers found the algorithm was 94.2% in alignment with AHA guidelines for stage 1 hypertension (defined as 130/80 mm Hg) compared with a continuous BP monitor, based on pulse wave features. Among the 40% of people who had early hypertension, accuracy rates were 86.2% for 30-second data segments and 80.9% for 5-second segments when compared with measurements from the continuous BP monitor.
Researchers used algorithm-to-video data for detection of type 2 diabetes, which achieved 75.3% accuracy utilizing blood flow patterns as markers compared with an HbA1c test.
“To implement this system as a medical device in society, its accuracy needs to be further improved and it must be examined in a larger-scale clinical study,” Uchida told Healio. “In our study, the participants were required to sit still for 5 or 30 seconds, but it would be useful if the disease could be detected even when they are moving. It is also necessary to test whether the same results are obtained in people from diverse backgrounds.”