Q&A: Newly identified genetic loci may allow future prediction of type 2 diabetes risk
Key takeaways:
- Researchers identified 145 novel loci associated with the development of type 2 diabetes.
- Eight mechanistic clusters were formed to link genetic associations with type 2 diabetes complications.
A set of newly identified genetic risk factors tied to the development of type 2 diabetes could eventually lead to the discovery of genotypes that allow providers to identify people at highest risk for complications.

In a genome-wide association study, published in Nature in February, researchers found 1,289 distinct genetic signals mapped to 611 loci that are associated with type 2 diabetes. Of these, 145 had not been previously associated with the disease in genome-wide association studies. The discovery was a big leap forward, according to Marijana Vujković, PhD, MSCE, research assistant professor of medicine in the division of translational medicine and human genetics at University of Pennsylvania Perelman School of Medicine.
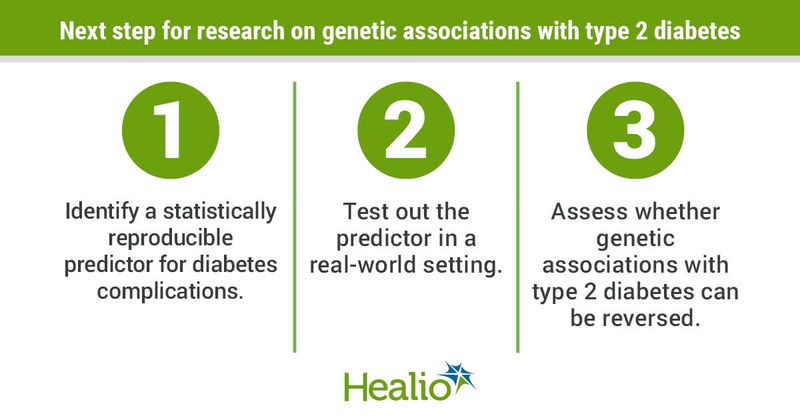
“While other disease areas might only identify tens or dozens of genetic variants due to smaller sample sizes, the diabetes research community has, since the start of the genome-wide association study era, been at the forefront in amassing vast datasets and combining efforts that has enabled the identification of hundreds of genetic variants,” Vujković told Healio. “We are currently helping advance our understanding of disease risk by annotating all of these variants based on proposed mechanisms.”
Researchers placed each newly identified genetic association into one of eight mechanistic clusters that they hope might help explain how a person’s type 2 diabetes developed and their risk for complications. For example, genetic signals in one of two beta-cell dysfunction clusters are associated with increased fasting glucose, 2-hour glucose and HbA1c and decreased fasting insulin.

While genetics is not ready for use in clinical practice today, the work being done in genome-wide association studies could help pave the way for precision care in the future, according to Benjamin Franklin Voight, PhD, associate professor in the department of systems pharmacology and translational therapeutics and department of genetics at the University of Pennsylvania Perelman School of Medicine.
“The idea that perhaps you can see differences between who may or may not progress to complications using a subset of genetic data, that could be important, but more work is clearly needed,” Voight said.
Healio spoke to Voight and Vujković about the novel loci identified in their study, how they determined mechanistic clusters associated with type 2 diabetes and how providers could use genetics to improve type 2 diabetes treatment in the future.
Healio: Where do things stand in terms of research on genetics and type 2 diabetes? Why was it important to conduct this study?
Voight: We tend to operate at the early preclinical stages. Initially, we just want to know the answer to a simple question: Does the DNA sequence that one carries at a particular place in the genome — a sequence that may differ between you and I — even matter for your diabetes risk? If so, can we simply find all the places in the genome where that matters?
We’re at the early stage on the prediction side. The place where this work probably has the most potential meaning is giving us leads to try to figure out what the genes are and what the biological mechanism is, hopefully, to tease out leads that may be modifiable therapeutically one day.
Vujković: The genetic community in cardiometabolic diseases has historically been a leader in terms of discovery and innovation. Our community holds a strategic advantage to conduct large-scale studies because of the high prevalence and rising incidence of chronic diseases. Because of that, we have a study of 2.5 million individuals. When we evaluate the progress made over the last year or 2, the advancements might seem incremental at first glance; however, this perspective overlooks the broader impact of these discoveries. Each new genetic locus potentially represents a step forward in our understanding of the genetic architecture of type 2 diabetes.
Healio: In your study, you found 145 loci that were not previously reported to be associated with type 2 diabetes. How important was this finding?
Voight: I’m always greatly impressed that we pull these things together at some level. It’s an exercise in statistical faith and community logistics. But the approach is sound and we know that our potential to discover new loci relates to statistical power from adding the new cohorts we can bring together. We pulled together 1 million more people and increased the number of loci that we found by 30%.
What changes the way I think about this is that we are starting now to have enough data to go after the genetic sub-clustering experiment. That is not a surprise because these clustering experiments for type 2 diabetes have been previously explored, so we knew we were going to find clusters. Our investigation for complications is one potentially interesting direction for this. We know that diabetes is complicated. Maybe different genetic components of the disease give you different risks for heart attack, peripheral artery disease or end-stage kidney disease.
Vujković: We were both expecting to find novel variants. In quantitative research, a larger study size is synonymous with greater discovery potential. The guiding principle is “more is more,” by increasing your study size you know you will find novel genetic associations. What is different compared to studies performed a decade ago is that we are finding variants with smaller effect sizes and variants that have a low prevalence in the population. Back then, the studies were small, so we were statistically powered to find discoveries of big effects. We’re currently identifying many new variants with tiny effects, which is a challenge on its own. You find 600 variants and now what?
Healio: Can you discuss the eight mechanistic clusters you found in the study? How do these clusters inform us about the cause of type 2 diabetes?
Vujković: Because we have this list of 600 loci associated with diabetes, we decided to organize them into groups based on their associations with other cardiometabolic traits, such as glucose, cholesterol, BMI and liver enzymes. Using cluster analysis, we identified that these loci broadly separated into eight distinct clusters.
For example, we observed that a subgroup of variants that increase diabetes risk also increase levels of glucose, HbA1c and proinsulin and were categorized into a beta-cell cluster, indicative of their relevance to pancreatic function. Diabetes-associated loci that additionally increased BMI and other body size measures clustered together into an obesity grouping, while those linked to liver enzymes and lipid levels were classified into a liver/lipid metabolism cluster.
The cluster analyses would identify these eight clusters, but it was up to us to assign meaningful interpretation to understand their biological significance and provide deeper insights into the underlying causes of diabetes.
Voight: One way you can add meaning to clusters is to look at the distribution of traits associated with a clusters. We can look for annotations in the genome that are enriched in the associations we found within a cluster, but are measured in different tissues and cell types.
We know that cells make proteins that go off and do a lot of the work that a cell needs to function. However, in the last 20 to 30 years, we’ve learned that when, where and in what context those proteins are expressed matters a lot — more precisely, how genes are regulated — which differs across cells in different organs in the body. Intuitively, this makes a lot of sense: The cells in your brain, for example, are doing different things than cells in your liver and, as such, have different programs of genes operating.
Driven heavily by high-throughput sequencing technologies, the genomics community has gotten good at measuring the genome in ways that reflect this; for example, finding many of the regions across the genome that are accessible to the machinery that turns on and off cells in beta-cell islets, but is not accessible in liver, brain or, say, toenails.
We’ve learned that in part our biological complexity as an organism is drive by the way in which genes are regulated. Gene regulation is super complicated, diverse, sensitive to stimulation and is often specific to the type of cell. We took a plethora of these annotations generated by the community across many tissues, cell types related to diabetes and perhaps others without prior expectations of relationship to diabetes, all across different cell types in the body. Then we asked, for these clusters, what annotations do we often find there? That allows us to attach meaning.
Healio: Could the findings in this study be used to develop targeted interventions to slow the progression or prevent type 2 diabetes for people at high genetic risk?
Voight: My standing running line is: If your risk for diabetes is at all a concern to you, you should talk to your health care professional. They will look at your physiology, your family history, your lifestyle and behaviors and maybe one day your genetics, and almost surely will provide guidance that will help make good health decisions for you. Far better than what our genetic studies here have uncovered so far.
That said, one day — tantalizingly — I think this work opens a sliver of light from the doorway to where genetics can make an important, serious contribution. A clinician can tell you what you could do personally in your life to lower your risk of diabetes given the context of your family history, but may not be able to say decisively if you are fated to progress to a complication, like have diabetic retinopathy. If you’ve got an extreme form of diabetes and have uncontrolled glucose levels, morbid obesity and a deep family history, then you might be able to make a pretty good and educated guess. But if the patient was age 20 years, in many cases we may not know what the future holds for them later in life.
The tantalizing prospect is that with more work, genetics might have a place in that conversation and provide some insight and guidance.
Healio: What is the next step in terms of research in this area?
Voight: One direction is identifying a robust, statistically reproducible predictor from the genetic subtypes and making it better powered. For example, taking one of our clusters, refining it, making it meaningfully predictive, so it can distinguish twofold, threefold, fivefold difference in somebody’s individual risk of retinopathy or one of these complications. That’s hard work, but an angle worth potentially investigating.
A subsequent step, after you have a robust and tested predictor in a research context, is to test it out in a clinical context. Take what we do at the bench and try to implement it. Can we go to a health system, show it to a clinician, flag the importance in their patient pool of those that are most at risk, and see if that leads to actionable prospects? What are the challenges there that we have not yet considered? That’s also super hard work, but implementation science is just as important as getting the science right at the bench.
A third step is that you’d like to know if there a way of reversing the clock, of undoing the genetics for those people who have this elevated genetic risk. Is there a pathway that’s broken that we can target therapeutically? Again, super hard work and it requires forming different types of partnerships. But hopefully one can appreciate that there’s a lot to do that needs collaborative engagement with a variety of different stakeholders.
Vujković: Currently, a lot of research reports statistically significant associations of polygenic risk scores, but so far these associations have not translated to clinical relevance.
There is negligible improvement in prediction accuracy of adding genetic risk scores to established and readily available clinical risk models to predict who will develop diabetes. Ideally, awareness of one’s genetic predisposition, especially in early adulthood, could incentivize lifestyle modifications to mitigate the risk of obesity-induced diseases. Yet, decades of research have shown minimal efficacy of lifestyle interventions.
I see a bigger potential of genetics discovery for drug discovery and repurposing. For example, genome-wide association studies identified that individuals with certain PCSK9 genetic variants have naturally low levels of LDL cholesterol, which led to the development of PCSK9 inhibitors. This therapy substantially lowers cholesterol and cardiovascular risk, which is especially relevant in people who are intolerant to statins.
Finally, genome-wide association studies can help in identifying subgroups of patients that might benefit the most from what type of therapy, enabling more personalized and precise disease management strategies. We are conducting similar studies in the diabetes space, which will hopefully lead to the discovery and development of new therapies.
For more information:
Benjamin F. Voight, PhD, can be reached at bvoight@pennmedicine.upenn.edu.
Marijana Vujković, PhD, MSCE, can be reached at vujkovic@pennmedicine.upenn.edu.
Reference:
Suzuki K, et al. Nature. 2024;doi:10.1038/s41586-024-07019-6.