Universal risk prediction model may better estimate CVD for primary, secondary prevention
Key takeaways:
- A universal model predicted CV events for people with and without baseline atherosclerotic CVD.
- Some patients with established ASCVD had lower CV risk than some patients without ASCVD.
Researchers reported that a universal risk prediction model incorporating traditional risk factors and cardiac biomarkers accurately estimated risk for adults both with and without baseline atherosclerotic CVD.
The model, which included risk enhancers recognized in cholesterol guidelines, predictors of HF and measurements of high-sensitivity cardiac troponin and N-terminal pro-B-type natriuretic peptide, could streamline clinical risk discussions between clinicians and patients in the transition from primary to the stable phase of secondary prevention, as well as identify people without ASCVD who have “CVD-equivalent” risk, Kunihiro Matsushita, MD, PhD, professor in the departments of epidemiology and cardiology at Johns Hopkins Bloomberg School of Public Health, and colleagues wrote.
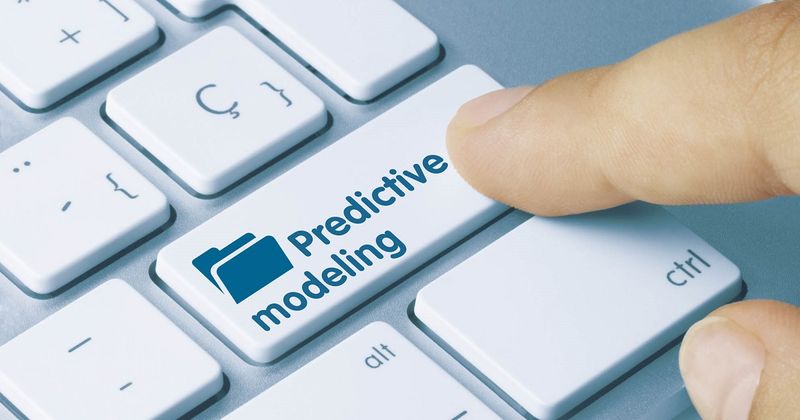
Image: Adobe Stock
“It is possible to create a universal risk prediction model that can be used for both people without prior ASCVD and those with prior ASCVD,” Matsushita told Healio. “Some people without prior ASCVD actually have higher risk for developing CVD than some patients with prior ASCVD.”
Adding cardiac biomarkers, risk enhancers to model
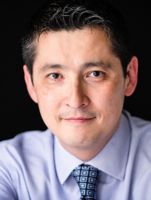
Matsushita and colleagues analyzed data from 9,138 adults who participated in the Atherosclerosis Risk in Communities (ARIC) study with ASCVD (n = 609) and without ASCVD (n = 8,529) at baseline (1996-1998). Researchers defined ASCVD as a history of MI, ischemic stroke or symptomatic peripheral artery disease.
Researchers examined established predictors in the risk classification systems and other predictors, such as BMI, high-sensitivity troponin and NT-proBNP, along with assessing major adverse CV events, defined as MI, stoke and HF.
“We considered predictors in pooled cohort equation and the dichotomous secondary risk classification system from the 2018 American College of Cardiology/American Heart Association Cholesterol Guideline as established CVD predictors: age, sex, race, diabetes, systolic blood pressure, antihypertensive medication, total cholesterol, HDL, statin use, estimated glomerular filtration rate, smoking status and a history of HF,” the researchers wrote.
The researchers also included risk enhancers from the cholesterol guideline, including family history of premature ASCVD, high-sensitivity C-reactive protein, lipoprotein(a), triglycerides and apolipoprotein B, as well as BMI, NT-proBNP and high-sensitivity cardiac troponin T.
Researchers also evaluated the model’s performance.
The findings were published in the Journal of the American College of Cardiology.
During a median follow-up of 20 years, there were 3,209 major adverse CV events, including 2,797 events among participants without prior ASCVD.
Most predictors showed similar associations with MACE regardless of baseline ASCVD status. A universal risk prediction model with the established predictors and cardiac biomarkers showed good discrimination for both groups, with C statistics of 0.747 and 0.691, respectively. The researchers also found the model showed “excellent” calibration, irrespective of ASCVD status.
“More specifically, when we used our universal prediction model, individuals without prior ASCVD but in the top 20% of predicted risk had a higher observed risk than those with prior ASCVD but in the bottom 40% of predicted risk,” the researchers wrote. “Although some preventive therapies, such as [PCSK9] inhibitors or aspirin, are predominantly recommended in individuals with prior ASCVD, if we purely implement a risk-centered approach, our data demonstrate that the separation of primary vs. secondary prevention based on history of ASCVD is overly simplified.”
In external validation with a cohort of 5,322 participants in the Multi-Ethnic Study of Atherosclerosis (MESA), the universal prediction approach also identified people without ASCVD who had a higher CV risk than some people with ASCVD. With the MESA cohort, C statistics of universal model were 0.766 and 0.617 in participants with no ASCVD and with ASCVD, respectively.
“We probably should rethink the current approach of dividing primary prevention and secondary prevention simply based on clinical history of CVD,” Matsushita told Healio. “For any risk calculators to be used in clinical practice, endorsement from expert organizations will be important. For example, the American Heart Association together with experts recently released a new risk calculator, PREVENT, for guiding primary prevention. Thus, collaborative studies will be preferable to demonstrate that this new approach of universal risk prediction model perform well in broader populations.”
Redefining CV risk assessment
In a related editorial published in the Journal of the American College of Cardiology, Pier Sergio Saba, MD, PhD, a consultant in cardiology at University Hospital of Sassari, Italy, and colleagues noted that an ideal prediction model should be easy to use, provide clear information with readily available parameters and maintain good discrimination and calibration in diverse populations.
“Before the universal approach can be used in clinical settings, there needs to be significant external validation efforts in prospective cohort studies and real-world patient data, including comparisons with current risk models, and clearer definitions of ASCVD risk history,” Saba and colleagues wrote. “Additionally, given the incorporation of biomarkers, careful cost-benefit analyses may also be needed before broad use.”
Reference:
For more information:
Kunihiro Matsushita, MD, PhD, can be reached at kmatsus5@jhmi.edu; X (Twitter): @KuniMatsushita.