AI-driven data initiative aims to improve urban heart health
A new initiative using artificial intelligence and data analytics could help urban policymakers in New York City better target health resources toward the interventions that could have the greatest impact on heart health.
The AI4HealthyCities Health Equity Network, created by the Novartis Foundation and developed in collaboration with Microsoft AI for Health, aims to bring together data from the health and health influencing sectors and apply data science techniques to provide decision-makers with insights about the main drivers of CVD.
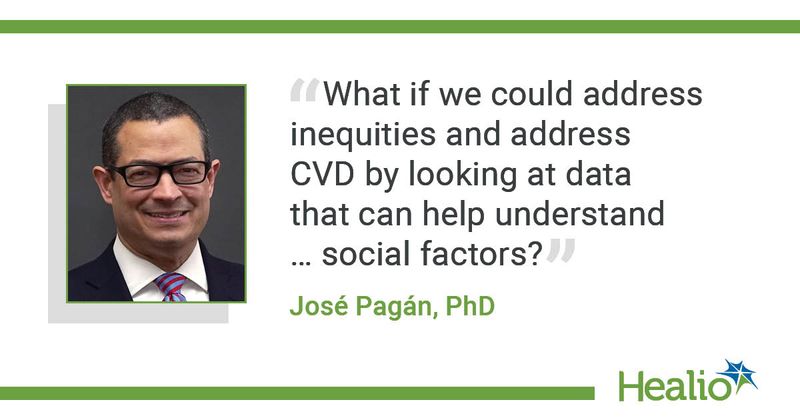
Data metrics that will be assessed include social determinants of health like housing, access to healthy food, physical exercise or green space, education, professional occupation, pollution, migration and the influence of structural racism and agism.
Healio spoke with Jose Pagán, PhD, professor of public health policy and management at the NYU School of Global Public Health and chair of the board of directors of NYC Health and Hospitals, about how social factors influence health outcomes, how physicians might use data analytics in the clinic, and the goal of creating “digestible” data that can be used quickly and easily.
Healio: How did this partnership come together?
Pagán: Novartis and Microsoft have been working together for quite some time now. There is substantial data in New York City and disparities that need to be addressed. This provides an opportunity. We met with Microsoft and Novartis and worked together on a general idea that the use of data analytics — data provided by the health system and the community, together with the analytical power, tools and expertise that Microsoft has — can help us to address CVD. If you ask any physician, they will tell you health care is important, but social factors are important also. Approximately 20% of the variation in health outcomes is connected to health care and 80% is connected to social factors. What if we could address inequities and address CVD by looking at data that can help understand those social factors at the neighborhood level?
Healio: What types of data are being analyzed and why?
Pagán: The city of New York puts out data on transportation, everything from subway stops to parking meter data, health care data, anything of which you can think. Then, there is data collected by the federal government and at the local government level. The first step is understanding what information is out there. The next step is how can we put these data together in a way that it can be analyzed with AI and data science? We want to bring all that information together to present it to community members, to health systems, to anyone who is interested in knowing why, for example, hypertension is higher in one neighborhood vs. another. Or, if I have a patient, how can I help them by better understanding what life is like where they live and their social circumstances?
Healio: Why does a metric like subway stop data matter? What do these types of data points have to do with CV health?
Pagán: I could ask someone questions that tell me whether they might be food insecure, or if they have trouble paying rent. If you are food insecure and are managing diabetes, that is impacting your diet. If you live in an unsafe area, you may exercise less, walk less. There is data you can collect from individuals. Then, there is aggregated social data that gets into the context of those answers. Maybe a patient does not feel food insecure, but they live in an area known as a food desert. Those are two distinct pieces of information that when brought together, can tell you a lot about a person’s circumstances. If you know that these things are connected to the outcomes you are looking at, say diabetes or hypertension, you can incorporate that information into their visit. There is a lot of complexity and having a tool to sift through that data is exciting.
Healio: What is the goal with this data?
Pagán: If we identify certain patterns, we want to take that information and develop a social risk index that tells you something about that individual and associates it with data from their health system. We hope this is just the beginning. We want to make this data and this analysis useful for clinicians, for people planning where social services should be located. Then, we want to take it a step further and try to look at other sectors that may be interested in this information and partner with them. It is easier to start with the health care system, because they are large and integrated in New York City. I can see, for example, a local nonprofit that addresses food insecurity or housing using this information to develop programs tailored to specific New York City neighborhoods.
Healio: What are the next steps?
Pagán: We need to identify the different data sources. The next step is curating the data and understanding it, to collaborate with Microsoft to analyze it, look for patterns and decide how that information should be shared and in what format. We want to make it digestible and useful, quickly. We have talked about creating maps at the ZIP code or neighborhood level that give you a sense of risk across neighborhoods. Getting feedback from different organizations and individuals that may find this information useful is critical. Many times, it may seem like you think you know what people need, but that is a huge assumption. You may miss the obvious. Data like these will help provide better answers.
Reference:
- Kennedy S. Microsoft, NYU, Novartis launch AI-driven heart health equity network. HealthITAnalytics. Available at: healthitanalytics.com/news/microsoft-nyu-novartis-launch-ai-driven-heart-health-equity-network. Published Sept. 19, 2022. Accessed Oct. 26, 2022.
For more information:
Jose Pagán, PhD, can be reached at jose.pagan@nyu.edu.