Machine learning tool catches rapidly progressing knee osteoarthritis in younger patients
Key takeaways:
- Machine learning models could aid early diagnosis of rapidly progressing knee OA among younger patients.
- The approach could be applied to other complex degenerative conditions, such as Parkinson’s.
A novel, automated machine learning tool reliably diagnosed rapidly progressing knee osteoarthritis in younger patients, according to data published in Annals of the Rheumatic Diseases.
The tool was designed with “transparency and reliability” in mind, making it more likely to be accepted by clinicians and patients than other “black box” methods, the researchers wrote.
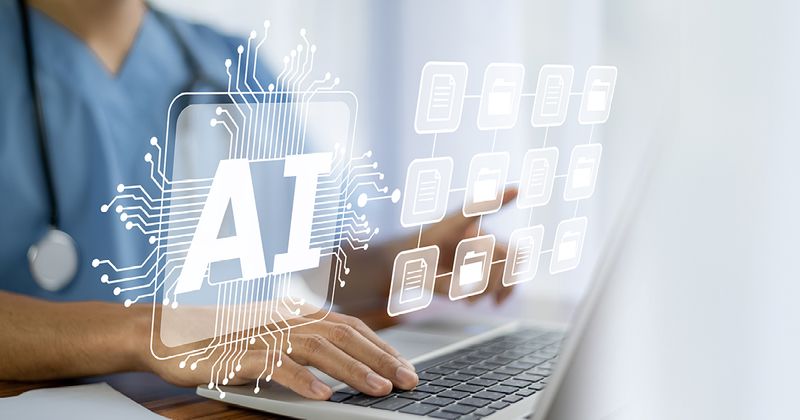
“Identifying patients at risk for rapid OA progression is crucial for accurate patient stratification, particularly in the early stages of the disease and among younger patients, a demographic increasingly affected by OA,” Simone Castagno, MBBS, a PhD candidate at the University of Cambridge, in the United Kingdom, and colleagues wrote. “... Younger individuals frequently face a ‘treatment gap’, where conservative management often falls short in managing symptoms and arthroplasty, though potentially beneficial, may not suit their active lifestyles and carries a higher risk of aseptic loosening and future revision surgery.
“We hypothesize that machine learning, a branch of artificial intelligence that uses algorithms to learn from data and make predictions without being explicitly programmed to do so, can be leveraged to identify individuals with OA who are at risk of rapid progression, especially in early stages of disease,” they added.
To test that hypothesis, Castagno and colleagues developed machine learning models using AutoPrognosis 2.0. The models were trained with data from a nested case-controlled study within the Osteoarthritis Initiative and externally validated against data from another. The researchers additionally used Post-hoc interpretability tools in AutoPrognosis to identify key attributes predicting rapid OA progression. They then conducted a “thorough validation” of the models, with multiple performance metrics and methods including stratified 10-fold cross-validation, hold-out validation and external validation, they wrote.
The researchers developed two types of models. The first was multiclass in nature, which predicted whether progression was pain-only, radiographic-only or both. The second model was binary, which classified patients as “non-progressors” or “progressors.” Each model’s performance was evaluated using area under the precision-recall curve (AUC-PRC).
Overall, there was robust external validation of relatively simple models that included only clinical variables, with multiclass predictions having an AUC-PRC of 0.727 (95% CI, 0.726-0.728) and binary predictions having an AUC-PRC of 0.764 (95% CI, 0.762-0.766), according to the researchers.
Predictive performance of the multiclass models was best for early-stage OA (AUC-PRC ranging from 0.724 to 0.806), while binary models demonstrated better reliability among patients aged younger than 60 years (AUC-PRC ranging from 0.617 to 0.693).
“We believe our approach is not only applicable to OA but could also serve as a model for other complex degenerative conditions (such as multiple sclerosis and Parkinson’s disease) which share common challenges, including chronicity, unmet clinical needs and difficulties in early diagnosis,” Castagno and colleagues wrote.
“By tailoring data inputs and fine-tuning models to these diseases, our method holds significant potential for the prediction and monitoring of such conditions,” they added. “This [machine learning] application represents a step toward a more tailored and precise approach to health care, addressing on the one hand the personalized needs of the individual patient while on the other hand delivering impact on a societal scale.”