Machine learning method could be ‘practice-changing’ for diagnosing gout
Key takeaways:
- Machine learning models diagnosed gout and calcium pyrophosphate deposition with accuracies similar to trained observers.
- The models could be applied at point of care without specific training.
Machine learning can be combined with Raman spectroscopy to objectively identify gout-causing crystals in synovial fluid and diagnose the condition with high certainty, according to data published in Rheumatology.
A machine learning model also accurately detected crystals leading to calcium pyrophosphate deposition disease. The technique, which can be applied at point-of-care, could “be a practice-changing method for rheumatologists worldwide,” the researchers wrote.
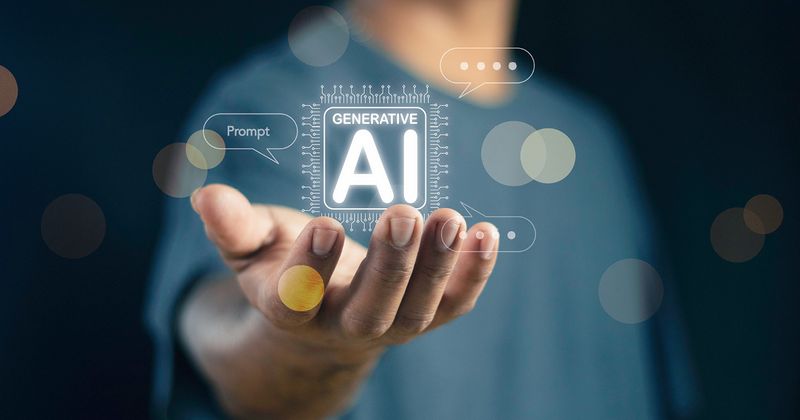
“It has long been known that synovial fluid analysis with polarized light microscopy is flawed as a tool for the diagnosis of gout and calcium pyrophosphate associated arthritis (CPPD),” first author Tom Niessink, MSc, of the department of bioengineering and technology at University of Twente, in the Netherlands, told Healio. “This method requires extensive training, is subjective, and has low interrater reliability.
“Raman spectroscopy has been proposed as a novel tool for synovial fluid analysis, as it has perfect specificity and requires no sample processing,” he added. “It is a new tool for clinicians, however, and interpretation of Raman spectra is therefore a barrier for clinical implementation.”
To help make the technique more accessible and objective, Niessink and colleagues developed a machine learning classification system and tested it on synovial fluid samples. The samples originated from 446 patients with various rheumatic diseases across three centers.
Using Python programming, the researchers developed a one-against-all classifier for monosodium urate crystals, and another for calcium pyrophosphate crystals, each of which consisted of a principal component analysis followed by a support vector machine. The model was trained on 246 fluid samples and validated against another 200.
Trained observers first used a Raman spectroscope to classify all spectra as monosodium urate crystals, calcium pyrophosphate crystals or other. Their classification was then compared with that of the machine learning algorithm.
Both the gout and CPPD models demonstrated diagnostic accuracies similar to Raman spectroscopy conducted by a trained observer, according to the researchers. There was 96% accuracy in the classification of calcium pyrophosphate crystals using the 2023 ACR/EULAR CPPD classification criteria (95% CI, 92.3-98.3), and 92.5% accuracy with monosodium urate crystals using the 2015 American College of Rheumatology/EULAR gout classification criteria (95% CI, 87.9-95.7).
The overall accuracy for the classification of pathological crystals was 88% (95% CI, 82.7-92.2). According to the researchers, processing a sample with Raman spectroscopy could be completed in 15 minutes, while the machine learning classification could be applied instantly.
“This is significant for clinicians, as our tool eliminates the burden of giving the proper crystal identification completely,” Niessink told Healio. “Our method provides a binary outcome — yes or no — and does so with great specificity.
“Our model is now validated for monosodium urate and calcium pyrophosphate crystals, but can also easily be applied for basic calcium phosphate crystals, such as hydroxyapatite,” he said. “Additional studies are necessary for validation of our model for those crystals.”
For more information:
Tom Niessink, MSc, can be reached at t.niessink@utwente.nl.