Machine Learning Effective for Detecting Flares in RA, Axial SpA
Click Here to Manage Email Alerts
Researchers successfully used machine learning to detect flares among patients with rheumatoid arthritis and axial spondyloarthritis, and to determine a strong association between patient-reported flares and less physical activity, according to recent findings in Arthritis Care & Research.
“Machine-learning allow multiple analyses of large datasets and make the best use of the available data, with minimal data amalgamation,” Laure Gossec, MD, PhD, of Sorbonne University in Paris, and colleagues wrote. “Although machine-learning methods have been little used in rheumatology to date, their usefulness in other medical fields has been clearly shown. The specificity of such analyses is that the data is fed into a machine-learning operations tool, which will build — by itself — classification models, generated most often using an ‘averaging’ of numerous naïve Bayesian classifications.”
To evaluate the link between patient-reported flares and physical activity using machine learning, Gossec and colleagues revisited data from the ActConnect study, a 3-month longitudinal French study of patients with either RA or axial SpA in 2016. During the prospective, multicenter study, researchers assessed patient-reported flares on a weekly basis, while physical activity was tracked continuously through a smartphone or tablet app.
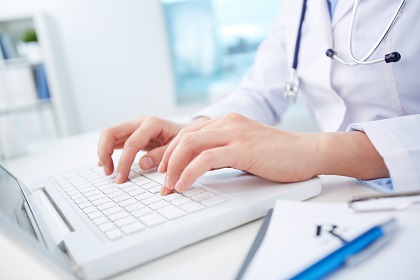
The trial included 82 patients with RA and 73 with axial SpA, representing 1,339 weekly flare assessments and 224,952 hours of physical activity. The study ultimately found that flares were related to a moderate decrease in physical activity.
In revisiting the study, the researchers applied machine learning techniques to the dataset. Calculations used to predict patient-reported fares were designed after completing intrapatient normalization of the physical activity data, using multiclass Bayesian methods, sensitivities, specificities and predictive values of the machinegenerated models of physical activity.
According to the researchers, patient-reported flares were frequent, with 72.2% of all participants noting at least one flare during the 3 months of follow-up. Patients reported flares in an average of 22.7% of all weekly assessments. The researchers’ model, generated through machine learning, was effective at predicting patient-reported flares, with a mean sensitivity of 96% (95% CI, 94-97), a mean specificity of 97% (95% CI, 96-97) and a mean positive and negative predictive value of 91%. A sensitivity analysis confirmed the findings.
“This pilot application of machine-learning to physical activity assessment will open the way to future studies,” Gossec and colleagues wrote. “The design of operational monitoring systems based on machine learning models would, however, require careful validations on much larger datasets and the present analyses should be considered as a proof of concept of such an approach.” – by Jason Laday
Disclosure: Gossec reports no relevant financial disclosures. Please see the study for all other authors’ relevant financial disclosures.