Big Data in Rheumatology: Is it a Cautionary Note?
Click Here to Manage Email Alerts
Let me introduce this cautiously entitled Editorial by first expressing how pleased we are to have engaged such a talented and productive group of experts on the use of “big data” in rheumatology. I am wholeheartedly enthusiastic about what we have already reaped from big data and firmly believe the greatest potential for gain in terms of research and care lie ahead.
I have personally used multiple big data sets in numerous research projects ranging from our work examining the risks for progressive multifocal leukoencephalopathy in rheumatic diseases to exploiting its power in the study of the vascular microbiome. With that said and since this is an Editorial, I want to explore this arena as a futurist of sorts, and ask whether there may be limits to the natural progression of how big data can and will be incorporated into rheumatologic practice.
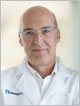
Machine Learning
While not primarily the focus of our Cover Story, I want to highlight an area many consider a natural extension and desired use of big data: to generate what is known as “machine learning.” Machine learning is not merely the application of expert-driven algorithms, but a process whereby extraordinary amounts of data can be combined in non-linear and creative ways to answer clinical questions ranging from the interpretation of a radiograph to the likelihood of how successful a patient’s ICU admission will be. Superficially, machine learning is akin to our traditional use of regression models, but now on big-time steroids.

A recent editorial in the New England Journal of Medicine was giddy over the potential and prospects of machine learning tools for transforming decision making traditionally left to physicians. It just gave me a moment to wonder whether there may be a downside to the continued application of big data in the patient care arena. Now, I hope you all know that I am far from a Luddite, but when I read the New England Journal of Medicine editorial, I was struck by something that was absent – I never found even a reference or mention made for the role of empathy in complex care models.
Empathy and Compassion
As rheumatologists, we are experts in applying empathy, which I define as our ability to stand in our patients’ shoes — a sort of cognitive and emotional perspective-taking that allows us to meet our patients where they are. Perhaps in the future, machine learning will solve our ongoing problem of being able to predict accurately which single or combination disease-modifying antirheumatic drugs and in which order will be best for our patients with rheumatoid arthritis. Instead of looking at 10 or 50 variables, machine learning will be able to incorporate 10,000 variables and then can tell us, to the highest degree of accuracy, that drug X is essential for patient Y. How nice would that be?
On one hand, this may solve our current uncertainty as how to predict efficacy and toxicity of powerful and expensive drugs to a degree we never thought possible. However, on the other hand, this output will likely neglect to tell us that this patient, woman and devoted wife we have known for years is more driven right now by her desire to be at home with her ailing husband and is too overwhelmed to even think about changing her course of therapy.
Cautionary Note
My cautionary note is we must seek the benefits of technology, but remain vigilant for when this must be informed by empathy and compassion. The translation of the Tao Te Ching Poem 2, which was written 2,500 years ago, should remind us that we define the world in opposites. Let me ask you if you can think of any other great technologic advances that were designed and touted to solve many of the problems of modern medicine but have inadvertently introduced many problems of their own? Can anyone say “electronic medical records?”
One last note is if you find yourself becoming too enamored of a world driven by numbers and data, read (or re-read) Solar Lottery by Philip K. Dick. It is a cautionary tale that is more relevant now than when it was written more than 60 years ago.
Thanks for reading this issue of Healio Rheumatology. Please email me at calabrl@ccf.org with your comments on the use of big data as well as other ideas for coverage in the publication. You can also follow me on Twitter @LCalabreseDO or @HealioRheum.
- Reference:
- Obermeyer Z, et al. N Engl J Med. 2016;doi:10.1056/NEJMp1606181.
- Leonard H. Calabrese, DO, is the Chief Medical Editor, Healio Rheumatology, and Professor of Medicine, Cleveland Clinic Lerner College of Medicine of Case Western Reserve University, and RJ Fasenmyer Chair of Clinical Immunology at the Cleveland Clinic.
Disclosure: Calabrese reports he is a consultant for Genentech, Pfizer, Bristol-Myers Squibb, Glaxo-SmithKline, Sanofi, Jansen and AbbVie; and is on the speakers bureau for Genentech, AbbVie and Bristol-Myers Squibb and Crescendo Bioscience.