Artificial neural network has ‘excellent’ performance in predicting COPD exacerbations
Key takeaways:
- An AI model could predict COPD exacerbations using urinary biomarkers.
- Additional studies are needed before employing this model in practice.
The ability of an artificial neural network to predict COPD exacerbations from urine samples was “excellent,” according to results published in ERJ Open Research.
“A predictive test of an impending COPD exacerbation that can be done easily in a patient's home using a urine dipstick would allow the clinician to personalize an early intervention to reduce exposures, optimize standard therapy or make decisions on antiviral, antibiotic or anti-inflammatory therapy,” Christopher E. Brightling, PhD, FMedSci, NIHR senior investigator and clinical professor in respiratory medicine at the University of Leicester, told Healio.
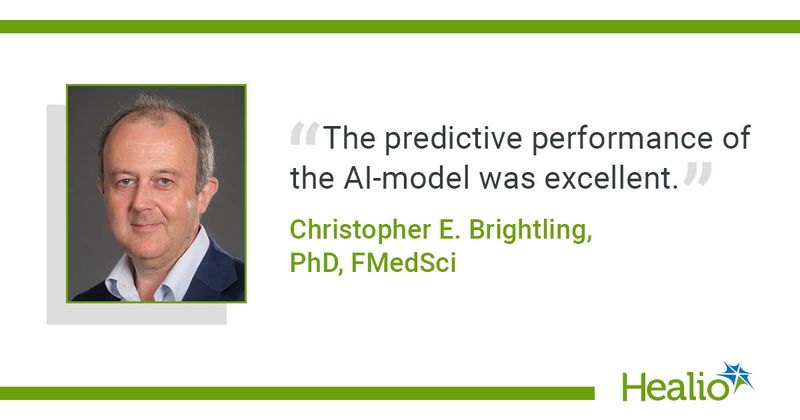
In this study, Brightling and colleagues found the 10 most discriminatory biomarkers (out of 35) for identifying a stable state vs. an exacerbation state in 55 patients with COPD via paired urine samples and then tested this biomarker panel using urine dipstick test data from 105 patients with COPD collected every day for 6 months.
Notably, the validation cohort was made up of 26 patients with paired stable and exacerbation urine samples, according to the study.
Researchers also created and tested an artificial neural network (ANN) to assess an AI model’s ability to predict exacerbations using urine biomarkers from 85 patients in the study population.
The biomarkers discovered as the most discriminatory included neutrophil gelatinase-associated lipocalin (NGAL), tissue inhibitor of metalloproteinase 1 (TIMP1), C-reactive protein (CRP), fibrinogen, club cell protein 16 (CC16), formyl-methionine-leucine-phenylalanine (fMLP), tissue inhibitor of metalloproteinase 2 (TIMP2), alpha-1-antitrypsin (A1AT), beta-2-microglobulin (B2M) and retinol-binding protein 4 (RBP4).
Of the 10 biomarkers, the study reported that five were measured by the urine test and included in the ANN: NGAL, TIMP1, CRP, fibrinogen and CC16.
“Our study included several steps from careful selection of the best mediators to include in the urine dipstick, design of the reader and testing its usability,” Brightling told Healio. “We were therefore confident that the device would give accurate and robust data to allow for the development of the AI model.”
In the cohort used to identify the biomarkers, researchers observed a receiver operating characteristic curve (ROC) area under the curve (AUC) of 0.84 in distinguishing exacerbation vs. stable state.
A similar ROC AUC was found in the validation cohort (0.81), according to the study.
Researchers reported an even higher/better AUC (0.89) when evaluating the ANN model’s ability to predict an exacerbation within a 13-day window.
In terms of how far ahead the AI model could predict an exacerbation before clinical diagnosis, 7 days was the median found, according to the study.
“The predictive performance of the AI-model was excellent and gives us encouragement that it will have an important role in the clinic,” Brightling told Healio.
“Future studies are required to further validate the predictive tests and the AI-model,” Brightling added.
Reference:
- AI analysis of urine can predict flare up of lung disease a week in advance. https://www.eurekalert.org/news-releases/1065172. Published Nov. 19, 2024. Accessed Nov. 19, 2024.
For more information:
Christopher E. Brightling, PhD, FMedSci, can be reached at ceb17@le.ac.uk.