Deep learning may reduce need for lung biopsies in patients with ILD
Compared with visual assessment, a deep learning model improved CT imaging prediction of histopathologic usual interstitial pneumonitis in patients with interstitial lung disease, according to study results published in CHEST.
Thus, this method may serve as an alternative to invasive lung biopsies among patients presenting with interstitial lung disease.
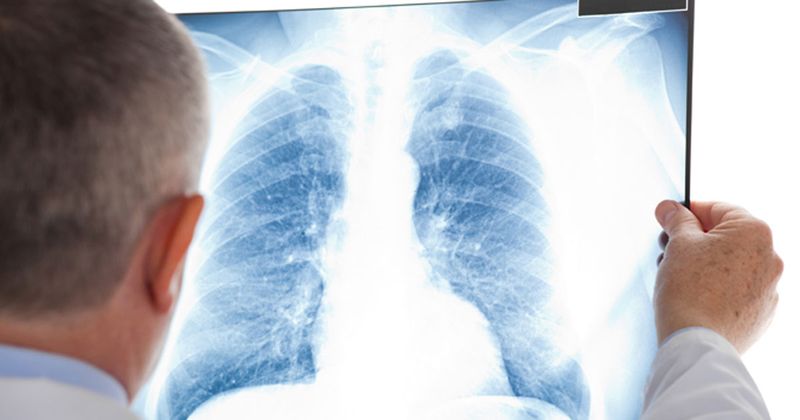
“To our knowledge, this study is the first to show superhuman performance in classifying [usual interstitial pneumonitis (UIP)] histopathology from chest CT scans using deep learning,” study investigator Alex Bratt, MD, thoracic and cardiovascular radiologist at Mayo Clinic in Rochester, Minnesota, and colleagues wrote.
For the study, Bratt and colleagues aimed to evaluate whether they could improve the noninvasive diagnosis of idiopathic pulmonary fibrosis, a progressive and often fatal form of ILD that presents with an UIP pattern on chest imaging, using deep learning, which, they wrote, “may perceive patterns that the human visual system cannot.” Deep learning may detect “subtle or imperceptible differences in imaging patterns among ILD subtypes” that can be difficult to perceive even among highly trained experts, they added.
The retrospective study included 1,239 patients (mean age, 62 years; 49% female) with pathologically proven ILD who underwent chest CT imaging. Researchers assigned a label based on histopathologic diagnosis (ie, UIP or non-UIP) to each case and trained a custom deep learning model to predict class labels from CT images in a training set (n = 894) which they then assessed in a test set (n = 198).
In addition, two subspecialty-trained radiologists manually labeled each CT scan in the test set using the 2018 American Thoracic Society IPF guidelines. Researchers compared the performance of the model with the performance of radiologists in predicting histopathologic class using area under the receiver-operating characteristic curves (AUC).
Results revealed that deep learning performed superiorly to visual analysis for the prediction of histopathologic diagnosis, with AUCs of 0.87 (95% CI, 0.82-0.92) vs. 0.8 (95% CI, 0.75-0.86; P = .03). Deep learning also outperformed the individual radiologists, with ratings between 0.7 and 0.78 (P < .05 for all).
Moreover, the reproducibility of the deep learning model was significantly increased compared with radiologist inter-rater and intra-rater reproducibility.
“We showed that our deep learning model provides higher diagnostic performance than visual evaluation ... with greater reproducibility,” Bratt and colleagues concluded. “Our model may reduce the need for invasive lung biopsy in patients with atypical or less evident UIP CT imaging patterns by increasing confidence in underlying UIP histopathologic diagnosis.”