AI tool shows promise for detection of incidental PE
An artificial intelligence tool for detecting incidental pulmonary embolus on conventional contrast-enhanced chest CT had high negative predictive value and moderate positive predictive value for detection, researchers reported.
Moreover, the AI tool even found some incidental PEs that were missed by radiologists, according to a press release.
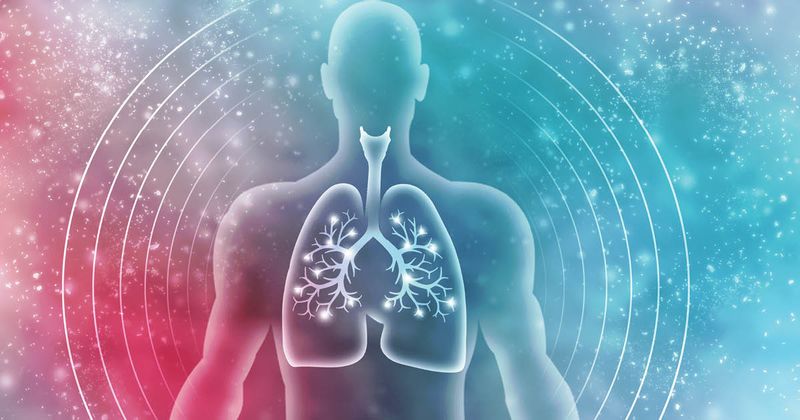
“AI algorithms for PE detection on CT pulmonary angiography examinations have shown high diagnostic performance, with a sensitivity of 92% for segmental PE and 90% for subsegmental PE,” Kiran Batra, MD, assistant professor of radiology at the University of Texas Southwestern Medical Center, Dallas, and colleagues wrote in the American Journal of Roentgenology. “However, the performance of AI algorithms for detection of incidental PE remains poorly investigated.”
The retrospective study enrolled 2,555 patients (mean age, 53.6 years; 52.4% women) who underwent 3,003 conventional contrast-enhanced chest CT examinations from September 2019 to February 2020. Researchers applied a commercial AI algorithm to the chest CT images to detect acute incidental PE. A vendor-supplied natural language processing algorithm was also applied to the reports to identify examinations identified as positive for incidental PE. The researchers then used a multi-reader adjudication process to establish a reference standard for incidental PE for all examinations identified as positive by the two algorithms.
The frequency of incidental PE was 1.3% based on the adjudication process.
The AI algorithm detected four incidental PEs that were missed by clinical reports, and clinical reports detected seven incidental PEs that were missed by the AI algorithm. Compared with the clinical reports, the AI tool demonstrated lower specificity (92.7% vs. 99.8%; P = .045) and positive predictive value (86.8% vs. 97.3%; P = .03). the researchers reported no differences in sensitivity (82.5% vs. 90%; P = .37) or negative predictive value (99.8% vs. 99.9%; P = .36).
Researchers observed no variations in sensitivity or specificity in association with age, sex, examination location or cancer-related clinical scenario for the AI algorithm (P > .05 for all).
According to the researchers, potential explanations for the five false positives detected by the AI algorithm included metastatic lymph nodes and pulmonary venous filling defect. Potential explanations for the seven false negatives by the AI algorithm included surgically altered anatomy and small caliber subsegmental vessel.
“Potential clinical applications of the AI tool include serving as a second reader to help detect additional incidental PEs missed by radiologists or as a worklist triage and prioritization tool to allow earlier incidental PE detection and intervention,” the researchers wrote.