Machine-learning method detects REM sleep behavior disorder with nearly 92% accuracy
Key takeaways:
-
The AI-based algorithm analyzed movements during REM sleep and used five features of movements to detect REM sleep behavior disorder.
- The highest accuracy was achieved by combining all five features.
An automated machine-learning method that analyzed sleep movements recorded with a conventional 2D camera may improve diagnosis of rapid eye movement sleep behavior disorder, according to a study published in Annals of Neurology.
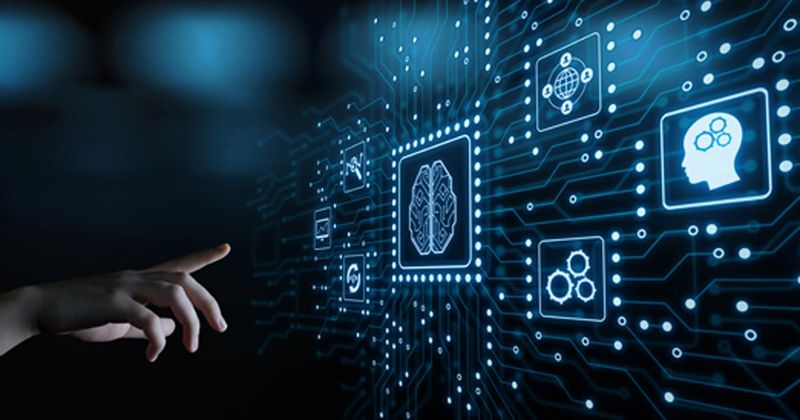
“Isolated rapid eye movement (REM) sleep behavior disorder (iRBD) is, in most cases, an early manifestation of synucleinopathy that affects 1% to 1.5% of the middle-aged and older populations,” Emmanuel During, MD, associate professor of neurology at Icahn School of Medicine at Mount Sinai, and colleagues wrote. “The neurophysiologic mechanism of RBD is motor disinhibition during REM sleep, causing repeated and brief movements of the extremities, head or trunk.”
While the definitive diagnosis of iRBD requires in-laboratory video polysomnography, which can be both costly and challenging to interpret, a recent study used a 3D time-of-flight camera in a sleep laboratory with high accuracy, the researchers wrote.
In this study, During and colleagues sought to replicate and expand on those results utilizing a conventional 2D camera and a dataset of 172 participants — 81 with iRBD and 91 non-RBD healthy controls — evaluated between 2016 and 2022 at Stanford Sleep Center. The control group included 28 healthy sleepers and 63 individuals with other sleep disorders, who were matched to iRBD patients by age and sex.
All video-EEG polysomnography recordings were made with a 2D camera, shooting at 30 frames per second and were manually scored by two blinded sleep experts. A blinded sleep specialist also reviewed all recordings of iRBD patients to determine which individuals had visible movements in REM sleep.
The researchers then used an optical flow computer vision algorithm to analyze these recordings and extract features of rate, ratio, magnitude and velocity of movements, and ratio of immobility.
After review, it was determined that the control group was comprised of 28 healthy individuals and 63 with a sleep disorder. Within the iRBD group, 11 individuals did not have visible movements during REM sleep.
According to the results, individuals with iRBD demonstrated more movements per hour of shorter duration (56.3 vs. 41.8; average movement duration, 3.4 vs. 4.2 seconds), as well as immobility periods, compared with controls.
The accuracy for distinguishing iRBD from other sleep disorders ranged from 84.9% to 87.2%, with the highest accuracy (91.9%) achieved when combining all five movement features and analyzing only short movements. Of 11 patients with iRBD who did not have visible movements during REM sleep, seven were correctly identified.
“This automated approach could be integrated into clinical workflow during the interpretation of sleep tests to enhance and facilitate diagnosis, and avoid missed diagnoses,” During said in a related Mount Sinai press release. “This method could also be used to inform treatment decisions based on the severity of movements displayed during the sleep tests and, ultimately, help doctors personalize care plans for individual patients.”
Reference:
- Mount Sinai-led team enhances automated method to detect common sleep disorder affecting millions. https://www.mountsinai.org/about/newsroom/2025/mount-sinai-led-team-enhances-automated-method-to-detect-common-sleep-disorder-affecting-millions. Published Jan. 9, 2025. Accessed Jan 14, 2025.