Digital tool aids stroke rehabilitation by tracking arm movements
A sensor-equipped computer program was nearly 80% effective in identifying and counting arm movements in patients undergoing stroke rehabilitation, researchers from NYU Grossman School of Medicine announced.
According to the CDC, stroke affects nearly 800,000 Americans each year, and arm mobility is seriously reduced in more than half of stroke survivors.

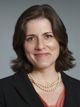
“Knowing how much physical rehabilitation stroke patients need to recover has been hampered by the inability to easily count training movements,” Heidi Schambra, MD, co-senior study investigator and associate professor of neurology and rehabilitation medicine at NYU Langone Health, told Healio. “Here, we show that it is possible to identify and count training movements in the impaired arm with an approach that uses wearable motion sensors and machine learning. Our measurement tool is an exciting step toward objectively capturing and dosing rehabilitation to maximize recovery.”
Schambra and colleagues used the NYU-developed tool PrimSeq to assess recordings of upper body movements in 41 adult stroke patients during routine exercises for regaining use of their arms and hands, a release from NYU announced.
Investigators recorded more than 51,616 upper body movements from nine sensors, with digital recordings of each arm movement, and matched the movements to functional categories, including reaching for an object or holding it still.
Schambra and colleagues then used machine-learning software to detect patterns within the data, which were linked to specific movements. The PrimSeq program was again tested on a separate group of stroke patients and successfully assessed most movements in patients with mild to moderate arm impairments from stroke.
“Ultimately, it will be important to have this tool readily available to researchers and therapists, and to this end we have made our machine-learning algorithm freely available,” Schambra said. “This tool could be used by researchers to identify training intensities that work best to maximize recovery.”
According to Schambra, she and her colleagues plan to make the tool more user-friendly by streamlining the wearable sensor array and packaging the algorithm in clinically relevant software.