Personalized models superior to population-based estimates for predicting SUDEP
Click Here to Manage Email Alerts
Models provided more accurate predictions of risk for sudden unexpected death in epilepsy than population-based estimates, according to results of a study that utilized a Bayesian logistic regression model.
The models remained more accurate even when they were “generalized to unseen data,” researchers noted in Neurology.
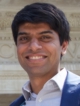
“Epilepsy is one of the leading causes of lost life-years worldwide,” Ashwani Jha, PhD, of the National Institute for Health Research University College London Hospitals Biomedical Research Center and the University College London Queen Square Institute of Neurology, told Healio Neurology. “Individuals with epilepsy often completely recover from seizures, but it is unclear why some tragically and unexpectedly die. Although we know of some factors that increase the risk for sudden unexpected death in epilepsy (SUDEP) on average, we cannot determine an individual's risk. In this study, we developed and validated the first predictive model for individualized SUDEP risk, based on routine clinical information only.”
Jha and colleagues re-analyzed data obtained via one cohort and three case-control studies conducted between 1980 and 2005. Using a Bayesian logistic regression model, they examined data from 1,273 epilepsy cases, of which 287 were SUDEP and 986 controls, and 22 clinical predictor variables.
Results showed superiority of cross-validated individualized model predictions compared with models created using only average population risk or generalized tonic-clonic seizure frequency. The researchers reported a mean cross-validated area under the receiver operating curve of 0.71 (95% CI, 0.68-0.74) for their model compared with 0.38 (95% CI, 0.33-0.42) and 0.63 (95% CI, 0.59-0.67) for the baseline average and generalized tonic-clonic seizure frequency models, respectively.
Upon application of the model to non-represented populations, Jha and colleagues observed a weaker performance. Generalized tonic-clonic and focal-onset seizure frequency, excess alcohol intake, younger age at epilepsy onset and epilepsy in one’s family history predicted SUDEP. Adherence to antiseizure medication reduced risk for SUDEP.
Although Jha said that the model should currently be restricted to clinical research use pending further validation in a future cohort, he expressed hope that the models will have two main benefits, with the first being clinical discussions regarding SUDEP potentially becoming more targeted and useful. This may reassure those at lower risk and motivate change, such as optimization of anti-seizure medications, among those at higher risk, according to Jha.
“Second, to understand the biological mechanisms of SUDEP, studies need to longitudinally follow up large groups for long periods of time, but such studies are impractical and difficult to get funded,” he said. “It is hoped that following up those at higher risk (as defined by this model) would make such studies easier to perform and quicker to turn around.”
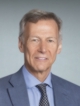
Orrin Devinsky, MD, professor in the departments of neurology, neurosurgery and psychiatry at NYU Grossman School of Medicine and lead study author, also commented on the potential impact of the study results in a press release.
"This study provides the first tool to assess a patient’s risk of dying from SUDEP and can allow them to see, for example, how improved medication adherence can translate into a longer life,” Devinsky said.
Reference:
University College London Queen Square Institute of Neurology. Sudden unexpected death in epilepsy a personalized prediction tool. Available at: https://www.ucl.ac.uk/ion/news/2021/may/sudden-unexpected-death-epilepsy-personalized-prediction-tool. Accessed May 12, 2021.