AI could help speed antibiotic selection for sepsis
Key takeaways:
- An AI system may speed up sepsis diagnosis and treatment compared with relying on culture growth.
- The system accurately matched diagnoses and treatment more than 90% of the time.
An AI system correctly chose antibiotics for sepsis treatment faster than the standard method using blood cultures, according to a study presented at ASM Microbe.
Researchers said the AI system, called Keynome gAST, can rapidly analyze bacterial whole genomes from patient blood samples without a culture and accurately select a treatment path.
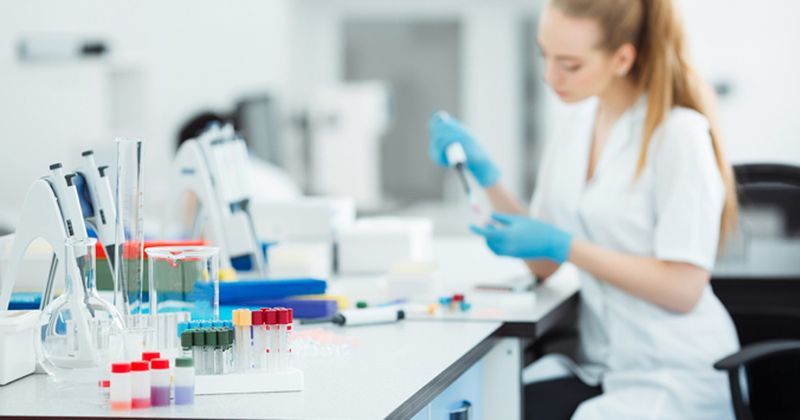
“The result is a first-of-its-kind demonstration of comprehensive and high-accuracy antimicrobial susceptibility and resistance predictions on direct-from-blood clinical samples,” Jason Wittenbach, PhD, director of data science at Day Zero Diagnostics and lead author of the study, said in a press release.
AI systems, from natural language processing to large learning models such as ChatGPT, are increasingly being studied for assistance in screening, diagnosis and treatment of infectious diseases.
Annually in the United States, at least 1.7 million adults develop sepsis, roughly 350,000 of them die during hospitalization for sepsis or are discharged to hospice, and one in three people who dies in a hospital had sepsis during their hospitalization, according to the CDC.
The current diagnostic standard for sepsis relies on culture growth, which can take 2 to 3 days and can delay treatment, increasing the risk for death.
Wittenbach and colleagues collected blood samples from patients suspected of bacteremia at four Boston-area hospitals between July 2023 and March 2024.
They processed the samples using the Day Zero Diagnostics process for ultra-high-enrichment of DNA from blood. Once sequenced, the bacterial genomes were analyzed by the company’s predictive antimicrobial susceptibility testing algorithm.
Overall, the researchers reported that predictive accuracy was achieved for 13 of 16 patient samples for 65 high-confidence models and an additional panel of 70 developmental panels.
According to the study, high-confidence categorical agreement predictions for diagnosis and treatment were reached 92.3% of the time, and the extended panels achieved categorical agreement 88.2% of the time.
Additionally, the panel produced predictions for all bacterial species encountered during the study and was just under 88% correct in predicting the drugs tested in hospitals. The panel was accurate 100% of the time for Escherichia coli and Pseudomonas aeruginosa, as well as for several clinically important drug selections against bacteria.
Although further study is needed because of the limited sample size, the study shows the potential for AI-assisted methods of antimicrobial susceptibility testing, Wittenbach said.
“This represents a critical demonstration of the feasibility of rapid machine learning-based diagnostics for antimicrobial resistance that could revolutionize treatment, reduce hospital stays and save lives,” Wittenbach said.
References:
- AI enables faster, more effective antibiotic treatment of sepsis. https://asm.org/press-releases/2024/june/ai-enables-faster,-more-effective-antibiotic-treat. Published June 14, 2024. Accessed June 20, 2024.
- CDC. Sepsis: About sepsis. https://www.cdc.gov/sepsis/about/index.html. Updated March 8, 2024. Accessed June 20, 2024.
- Wittenbach J, et al. CPHM12. Presented at: ASM Microbe; June 13-17, 2024; Atlanta.