Oncology leaders call for ‘ethical deployment’ and ‘responsible use’ of AI in cancer care
The AI revolution already has transformed delivery of cancer care.
New algorithms rapidly identify patterns or abnormalities on imaging, improving diagnostic accuracy. Large language models can craft responses to patient questions, and machine learning predicts treatments to which a patient is most likely to respond.

Other platforms can help match patients with appropriate clinical trials or perform ancillary tasks such as billing or documentation, potentially reducing physicians’ workload and the risk for burnout.
Much like other aspects of modern medicine, however, tremendous advances in AI have created new challenges related to practical implementation and appropriate use.
This Healio Exclusive provides insights into the complex issues oncologists are facing as they implement AI, a series of core principles ASCO released to guide responsible use, and recent research that highlights new ways AI may improve treatment decision-making and patient outcomes.
‘Ethnical deployment’
Oncologists are grappling with complex issues as they integrate AI into cancer care, according to results of a nationwide survey.
Most oncologists believe they should have the ability to explain how AI models work and must protect patients from biased AI, findings published in JAMA Network Open showed. Most respondents also indicated patients should consent to use of AI before it is implemented in practice.
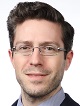
“Ethical deployment of AI in oncology must prioritize the development of infrastructure that supports oncologist training, as well as transparency, consent, accountability and equity,” Andrew Hantel, MD, faculty member in the divisions of leukemia and population sciences at Dana-Farber Cancer Institute and Harvard Medical School Center for Bioethics, told Healio. “It means that infrastructure needs to be developed around cancer AI to ensure its ethical deployment.”
Despite the potential benefits of AI to improve decision-making and outcomes, clinicians have expressed concerns about several aspects of implementation. These include AI bias; the ability of AI to detail its decision-making process; who bears responsibility for errors or misuse; and whose treatment recommendation takes precedence when a physician and AI do not agree.
“As AI begins to impact cancer care delivery, understanding the ethical implications from those who will be asked to implement it — oncologists — is crucial,” Hantel said.
Hantel and colleagues aimed to capture the views of practicing oncologists to ensure AI is deployed in an “ethnical way” that meets the needs of physicians and patients, he said.
Researchers sent a 24-question cross-sectional survey to nearly 400 oncologists in the U.S. Their analyses included responses from 204 oncologists (63.7% men; 62.7% non-Hispanic white) from 37 states. More than one-quarter (29.4%) of respondents worked in academic practices and about half (53.4%) had no AI training.
Most respondents (84.8%) agreed they should be able to explain AI decision-making before they use it. A greater percentage indicated patients should have to give consent to use AI treatment recommendations (81.4%) than to use it as part of diagnostic decisions (56.4%).
In cases when an AI model provides a different treatment recommendation than the oncologist, more than one-third (36.8%) of respondents felt the patient should be told about both options and make the decision.
“This finding highlights that many physicians are unsure about how to act in relation to an AI and counsel patients about such situations,” Hantel said.
Most respondents indicated they need to protect patients from biased AI (76.5%), but only 27.9% felt confident in their ability to do so.
“The alignment on these points underscores the urgent need for structured AI education and ethical guidelines within oncology,” Hantel said.
Nearly all respondents (90.7%) indicated AI developers bear responsibility for medical or legal problems that arise with the technology. Fewer than half suggested clinicians (47.1%) or hospitals (43.1%) share that responsibility.
“The FDA and regulatory agencies need to clearly define and delineate the responsibilities of all stakeholders involved in AI’s development and clinical application,” Hantel said. “This includes establishing standards for transparency, explainability and ethical oversight. Without this guidance, there will be no consensus. [That] poses risks for oncologists when an AI makes the wrong recommendation and they follow it, or when an AI tool is standard of care and they go against its recommendation.”
Education and training for oncologists will be essential, Hantel said. They also will need to understand the perspectives of patients — particularly those from historically marginalized or underrepresented groups.
“The ethical deployment of AI in cancer care is a shared responsibility,” Hantel said. “The ethics of its development and deployment need to be integrated from inception. Otherwise, we will be trying to fix something after it has harmed people rather than avoiding that harm altogether.”
Principles for ‘responsible use’
During a shareholder presentation in front of more than 500 physicians in April, Debra A. Patt, MD, PhD, MBA, FASCO, asked how many in attendance used large language models like ChatGPT and Claude.
Fewer than five raised their hands, and those who did seemed to use the AI software like a search engine without understanding of prompt optimization.
“There is a large opportunity to educate how physicians can best use AI tools to improve the care of the patients we serve,” Patt, executive vice president of Texas Oncology and chair of ASCO’s AI task force, told Healio.
“When you and I started driving, we used directions that we printed out or maps. It was difficult to learn how to do it differently,” Patt added. “The greatest fear I have is that health care organizations will be late adopters of digital health care tools that can improve care delivery.”
ASCO’s AI task force — comprised of about a dozen individuals, including board members, ASCO member physicians, patients and policy experts — issued “Principles for the responsible use of artificial intelligence in oncology” to guide the implementation of AI and ensure its use benefits patients and clinicians.
The six guiding principles address the need for transparency of AI tools and applications; the importance that clinicians and patients be aware when AI is used in decision-making; the need for AI developers and users to protect against bias and ensure equitable access to AI tools; compliance of AI systems with legal, regulatory and ethnical requirements that govern data use; the need for institutional compliance policies to govern AI’s use; and the need for human-centered application of AI, ensuring it complements but does not replace human interaction.
AI holds tremendous potential but also carries some risks. These include AI hallucinations, when models generate misleading or inaccurate results. AI also can exacerbate disparities or biases, reduce trust, and change clinicians’ roles, which could affect the quality of patient-centered care.
“Science can be used for good and evil,” Patt said. “We want patients to benefit from this tremendous innovation, and it’s a very exciting time. We have to be cognizant and responsible about the fact that these tools might have bias and errors. We need responsible guiding principles to see us through how we optimally benefit from these important technologic advances.”
Paint the picture of ‘the possible’
Patt shared an example of software that helps with administrative tasks, such as appointment rescheduling. It reduces staff burden, but can contribute to care disparities.
“If you have someone who has canceled their appointments a lot, that may deprioritize their reschedule,” Patt said. “It may be that they had to cancel their appointments because they have socioeconomic burdens to health care, transportation insecurity [or] a job they can’t manage when they have cancer. Deprioritizing them with subsequent appointments would provide inequity in care delivery because you are disenfranchising an already vulnerable part of the patient population, just as an example.”
Clinicians must learn to integrate AI tools into their care without causing harm, Patt said. This requires training and communication with patients.
Patt used an AI tool NIH developed called logistic regression-based immunotherapy-response score (LORIS). NIH hopes LORIS can help physicians predict whether someone may respond to immune checkpoint inhibitors.
“If I told a patient that I was going to use an AI tool to try to decide their likelihood of responding to immunotherapy, they need to understand that I’m using a mathematical model to make that determination because there might be things about that model that make it more or less likely for them to benefit,” Patt said. “It could be influenced by all sorts of things.”
These risks may cause clinicians to avoid using AI, but Patt thinks that would be a mistake.
“These issues of bias, error, privacy and accountability are real,” she said. “We need to give appropriate, heightened awareness of those concerns, but I do feel like the greatest challenge we have to overcome in medicine is to paint the picture of ‘the possible’ for the oncology community [and] to educate the masses of clinicians on how to use these models to do what they do better.”
Patt recalled opening her browser to National Comprehensive Cancer Network guidelines on her computer 20 years ago when she finished her fellowship. Now, fellows can use large language models to access NCCN and ASCO guidelines, making it easier to access relevant content.
Clinicians may not feel at ease about AI, but if development and implementation adhere to the task force’s principles, Patt said the possibilities are “endless.”
“We had to go through the technical challenges of early smart devices like PalmPilot before we had other iterations of the smartphone that were much better,” Patt said. “AI application and use is probably the most important development of our time. It has amazing potential to help doctors, all medical professionals and, most importantly, the patients we serve together.”
‘Promising utility’ of chatbots
Several studies published or presented in the past few months highlight the potential of AI in oncology practice.
AI could help answer some patient questions about cancer, reducing clinician burden and improving access to care, according to findings published in JAMA Oncology. Multiple chatbots produced responses that had greater empathy and higher quality scores than physician responses.
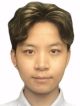
“Chatbots pose the promising potential to draft template responses for clinician review to patient questions,” David Chen, BMSc, medical student at University of Toronto, told Healio. “However, we remain cautious about the need for clinician oversight to ensure medical accuracy and alignment with humanistic elements of physician-patient relationships, such as building trust and rapport.”
Prior research showed chatbot responses produced more empathetic responses than physicians to general medicine questions in online forums.
“Given the widespread popularity of AI chatbots and emergent applications of these chatbots in clinical environments, we felt that it was important to evaluate the competency of chatbots in a more realistic clinical scenario where patients present with a question about their condition,” Chen said.
Researchers collected 200 random cancer-related questions posted on Reddit r/AskDocs between Jan. 1, 2018, and May 31, 2023. Investigators had three chatbots generate answers limited to the mean physician answer of 125 words.
Multiple indices measured readability, and attending physicians rated overall quality, empathy and readability. Tabulated scores ranged on a scale of 1 to 5, with 1 representing a “very poor” response and 5 signifying a “very good” reply.
All three chatbots had higher mean scores for response quality — based on medical accuracy, completeness and focus — as well as empathy.
The top performing chatbot had higher mean scores than clinicians in all three variables measured. Clinician responses scored higher for readability than two of the three chatbots.
“We were initially surprised at the positive performance of the tested chatbots given their lack of purpose-built design for medical question-answer scenarios, suggesting that these general-purpose, foundational AI chatbots harbor promising utility in specialized medical scenarios,” Chen said.
However, chatbot success does not mean they should be implemented without supervision, Chen said.
“Doctors remain in charge of chatbot oversight to ensure that chatbot responses are medically accurate,” Chen said. “The possible future implementation of chatbots to draft template responses to patient questions about cancer can help reduce physician burnout, so that physicians spend more quality, face-to-face time with patients rather than administrative clinical work such as drafting responses to patients.”
‘Practical framework’ to increase palliative care use
An algorithm-based referral system led to a fourfold increase in specialty palliative care use when implemented in a large community oncology network, results of a randomized study showed.
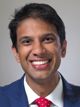
“We’ve done some prior work where we’ve used machine learning-based algorithms embedded in the electronic health record to prompt oncologists to have earlier conversations about symptom management and end-of-life care, and we’ve had pretty good effectiveness,” Ravi Bharat Parikh, MD, MPP, FACP, assistant professor of medicine and of medical ethics and health policy at Perelman School of Medicine at University of Pennsylvania, told Healio. “The challenge is oncologists are busy, so using that paradigm, that framework and applying it to palliative care referrals has been a strong interest area of ours. In this study, we sort of did an extension of that.”
The multiarmed BE-a-PAL trial included 562 adults (mean age, 68.5 years; 79.5% white; 48.8% women), 77% of whom had stage III or stage IV lung or noncolorectal gastrointestinal cancer. An automated EHR algorithm used prognostic or psychosocial factors to assign patients a risk score.
Researchers assigned 296 patients to the algorithm-based referral group. Oncologists received weekly default EHR notifications promoting specialty palliative care referral for high-risk patients, with patients whose oncologists did not opt out of the referral being introduced to specialty palliative care via a standard script with an offer to schedule a visit.
The 266 patients in the control group received referral to palliative care at their oncologist’s discretion.
Results showed a higher percentage of patients in the intervention group than control group completed palliative care visits (46.6% vs. 11.3%; adjusted OR = 5.4; 95% CI, 3.2–9.2).
Among patients who died during the study period, a lower percentage of those assigned the intervention received end-of-life chemotherapy (6.5% vs. 16.1%).
The results show the potential for a “practical, scalable framework to increase palliative care access with automated risk prediction,” researchers wrote.
However, additional factors need to be considered.
“There’s probably two or three areas that are a bit unanswered,” Parikh told Healio. “First, how do you design your algorithms in ways where you curate a higher-risk population? Our algorithms were still inaccurate some percentage of the time.”
The structure of the palliative care intervention also could be improved, Parikh said.
“In our case, the only intervention that we routed to was an initial palliative care consultation because we wanted it to be pragmatic. But any palliative care specialist will tell you that if you want to enjoy the benefits of palliative care most, it’s not just a single consultation that you need,” Parikh said. “Lastly, what happens if you try to deploy this where most patients receive their care, which are community-based oncology practices all around the country? It is a great question because it’s less about the technical capability and more about the preexisting motivations from these practices to be involved.”
References:
- AI tool predicts response to cancer therapy. Available at: https://www.nih.gov/news-events/nih-research-matters/ai-tool-predicts-response-cancer-therapy. Published June 25, 2024. Accessed Sept. 23, 2024.
- American Society of Clinical Oncology principles for the responsible use of artificial intelligence in oncology. Available at: https://society.asco.org/sites/new-www.asco.org/files/ASCO-AI-Principles-2024.pdf. Published May 31, 2024. Accessed Sept. 23, 2024.
- Ayers JW, et al. JAMA Intern Med. 2023;doi:10.1001/jamainternmed.2023.1838.
- Chen D, et al. JAMA Oncol. 2024;doi:10.1001/jamaoncol.2024.0836.
- Hantel A, et al. JAMA Netw Open. 2024;doi:10.1001/jamanetworkopen.2024.4077.
- Parikh RB, et al. Abstract 12002. Presented at: ASCO Annual Meeting; May 30–June 3, 2024; Chicago.
For more information:
Andrew Hantel, MD, can be reached at andrew_hantel@dfci.harvard.edu.
David Chen, BMSc, can be reached at davidc.chen@mail.utoronto.ca.
Ravi Bharat Parikh, MD, MPP, FACP, can be reached at ravi.parikh@uphs.upenn.edu
Debra A. Patt, MD, PhD, MBA, FASCO, can be reached at debra.patt@usoncology.com.