MRI-guided radiation with AI allows for real-time monitoring of glioblastoma treatment response
Researchers at Sylvester Comprehensive Cancer Center at University of Miami devised an MRI-guided radiation therapy technique using machine learning that may allow for real-time monitoring of glioblastoma treatment response.
The technique — which combines daily MRI with radiation — enables physicians to track a tumor’s progress through high-resolution images that offer insight into how the cancer is responding to treatment.
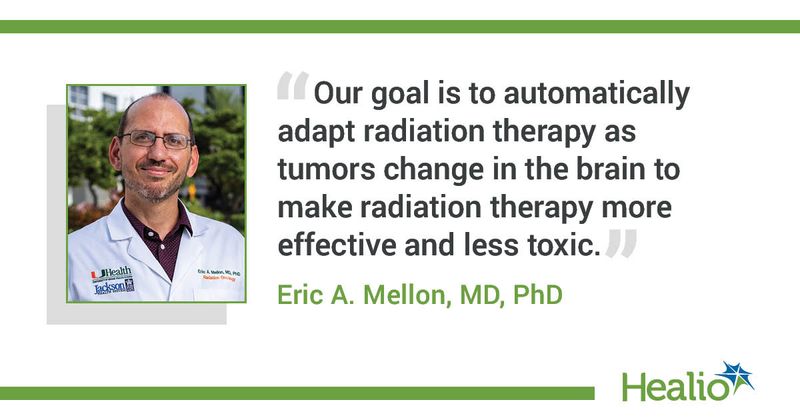
Traditional CT imaging only provides information about radiation beam positioning. The machine-learning component traces glioblastoma tumors and resection cavities in large MRI datasets and can process data in about 90 minutes.
The team is conducting a study in which physicians will evaluate glioblastoma tumor progression weekly among patients undergoing MRI-guided radiation therapy.
“Our goal is to automatically adapt radiation therapy as tumors change in the brain to make radiation therapy more effective and less toxic by treating only what needs to be treated,” Eric A. Mellon, MD, PhD, co-leader of Sylvester’s Neurologic Cancer Site Disease Group, told Healio. “Also, we want to know quickly when tumors are not responding appropriately, so that we can adapt therapy and use a different approach.”
Healio spoke with Mellon, along with co-first author Adrian L. Breto, a doctoral student and programmer in the laboratory of Radka Stoyanova, PhD, and co-first author Kaylie Cullison, an MD/PhD student in Miller School of Medicine’s medical scientist training program, about how this approach using artificial intelligence (AI) can more efficiently extract and interpret real-time information to guide glioblastoma treatment.
Healio: How does MRI-guided radiation therapy work, and how does it differ from traditional approaches?
Mellon: Glioblastoma evolves during radiation therapy. With new combination MRI and radiation therapy systems, we get MRIs every day during 6 weeks of the typical glioblastoma radiation therapy treatment.
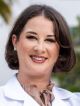
Cullison: Traditionally, glioblastoma is treated on radiation therapy systems that utilize cone-beam CT instead of MRI as the daily imaging for treatment set-up. You can only see bone on these CT images, not the brain inside the skull. For this traditional approach, patients only receive one MRI before treatment and one MRI after treatment, and physicians treat a large area of brain to attempt to cover any areas that change. This excess radiation can cause symptoms such as fatigue, changes in blood counts or cognitive changes after treatment. By using MRIs acquired daily during treatment, we can see how the tumor grows, shrinks or becomes inflamed with radiation therapy and chemotherapy during the course of treatment and adapt therapy. We also can see how the brain shifts and changes after surgery. All of these factors influence radiation therapy, which is a focal treatment. We previously published how taking these changes into account can spare parts of the brain known to influence cognitive outcomes after radiation.
Mellon: What AI is doing for us is automatically outlining the tumor structures within the brain so a human does not have to draw it every day by hand, which is time consuming and hard to standardize among different members of the research team. These outlines allow us to automatically track the volumes of the tumor structures during treatment.
Healio: What types of real-time information does this approach provide, and how can it guide decision-making?
Mellon: One issue the field struggles with is when the tumor grows, how do we know whether the tumor is just inflamed or if it is not responding appropriately to treatment? If the tumor is not responding, how soon should we change our approach to try to gain control? Due to this confusion, we typically make such decisions many months after radiation therapy finishes. At this point, it's often too late to act or the patient is irreversibly damaged by the tumor progression.
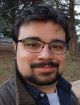
Breto: The AI identifies and outlines the lesion and surgical cavities automatically on the imaging, which provides information when taken day-by-day on how the tumor is changing.
Cullison: These automatic outlines allow the clinical team to see whether the tumor is changing in volume in real time during treatment, which means we can use this information to adapt the treatment field to the changing tumor anatomy. The next step for our research is designing a clinical trial that does just that — adapting the radiation treatment field to changing tumor anatomy and measuring outcomes to see whether survival time, cognitive ability or other outcomes improve among patients for whom we adapted therapy.
Healio: How can machine learning accelerate the speed at which information is available?
Mellon: Machine learning helps us because we get MRIs every day. This means we are getting 30 MRIs for each patient, each of which has multiple contrast types and many individual slices. For a person to manually draw out the tumor every day on dozens of images per day to analyze the tumor dynamics takes excessive amounts of time. Machine learning can do this in seconds instead of hours, speeding the pace of discovery in two important ways.
First, when the tumor is changing, machine learning can point out to us automatically that we need to do something. It’s easy to miss gradual changes when we look on a day-to-day basis. Second, for the research team to analyze the tumors to predict response, machine learning can allow us to gather that information constantly and immediately as the tumor grows so we can do further analysis of changes and act upon our models of response in real time. While this can be done manually, it’s so cumbersome to do without AI that it’s practically not feasible.
Breto: AI and machine learning models do a lot of heavy lifting in terms of time savings. You generate more structures in a shorter amount of time than a human given the same set of images. The net benefit to everyone involved is that the human becomes less of a ‘data laborer’ and more of a ‘data curator.’ You keep the strengths of raw power that AI has and combine it with the intuition and experience of a human observer, who can spot-check and curate results as needed. The net gain is higher data availability in a shorter time with less burden on clinicians, and that makes the entire system more reactive to potential changes in the patient’s care if needed.
Healio: What questions still need to be answered to refine this approach?
Mellon: First, how do we streamline the workflow so it goes straight from the MRI-radiation therapy system to analysis pipelines that will tell us automatically and rapidly whether the tumor is responding? Second, are there other approaches that might be more accurate in auto-segmentation?
Breto: On the computation and programming side, there are diminishing returns for adding more training data over time. So, one additional question is how can we leverage the data that exist to present it to the AI in a more effective way and let it capture more nuanced details present in imaging.
Healio: Is there anything else you feel is important to emphasize?
Breto: AI is seen in the public eye as something of a boogeyman, encroaching on many fields to try to make things faster and cheaper by removing the human element. In medicine, I doubt personally this will ever be the case. Treating humans requires the human touch. This tool is meant to be an assistant to the human physician, curating as much valuable data as possible to ensure the patient’s optimal care while freeing them from time-intensive tasks that impact the care of every patient they see. I believe this is the role of AI in medicine — to enhance patient outcomes as one of many supporting tools.
Cullison: The layperson’s reaction to my PhD project involving machine learning/AI is always a mix of intrigue, skepticism and fear, especially with ChatGPT and other language models receiving so much media attention in the past year or so. There is a lot of misunderstanding about machine learning in general, which feeds into the fear response. The tool we developed is meant to be a time-saver for a process that is manually laborious and time intensive. It still needs input from human experts to work properly. However, the overall goal is to create a pipeline that will allow radiation oncology clinical teams to be alerted when the tumor or surgical cavities are changing significantly, and to adapt radiation therapy for glioblastoma to those changes in real time. Our hope is that these methods will improve outcomes and prolong survival times for patients with glioblastoma.
Reference:
For more information:
Adrian Breto can be reached at a.breto@umiami.edu.
Kaylie Cullison can be reached at kcullison@med.miami.edu.
Eric A. Mellon, MD, PhD, can be reached at eric.mellon@med.miami.edu.