AI tool addresses ‘clinical conundrum’ by accurately identifying rare blood cancers
Click Here to Manage Email Alerts
Key takeaways:
- The AI-based algorithm demonstrated better than 90% accuracy for differentiating between diagnoses.
- The final trained model processed previously unseen diagnostic slides in approximately 6 seconds.
SAN DIEGO — An international group of researchers developed an artificial intelligence-based algorithm that differentiates prefibrotic primary myelofibrosis from essential thrombocythemia during pathological analysis, study findings showed.
Based on the model’s accuracy, researchers hope the algorithm can be incorporated into a clinical decision-making support tool that can fill a pressing need to improve diagnosis of these rare myeloproliferative neoplasms.

The results presented at ASH Annual Meeting and Exposition are “both enticing and provocative,” according to study investigator Andrew Srisuwananukorn, MD, clinical assistant professor of hematology at The Ohio State University Comprehensive Cancer Center.
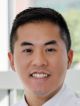
“We developed an algorithm that was able to differentiate between these two diagnoses with over 90% accuracy,” Srisuwananukorn told Healio. “The use of this algorithm as a practical clinical tool is a dream of mine — to eventually have artificial intelligence tools side-by-side with physicians to help support decision-making.”
Background
Diagnosis of prefibrotic primary myelofibrosis and essential thrombocythemia present a “clinical conundrum” because of overlapping clinical and histopathological characteristics, according to Srisuwananukorn.
Attaining the correct diagnosis is critical because despite characteristics similar to essential thrombocythemia, patients with prefibrotic primary myelofibrosis have significantly higher risk for developing constitutional symptoms or experiencing disease progression to overt myelofibrosis or leukemia, Srisuwananukorn said.
“These two diseases have quite different outcomes and therapeutic strategies, and as physicians it behooves us to know which disease these patients have upfront,” he added. “With the advent of artificial intelligence and machine learning, we posited that we could develop an algorithm to differentiate these two diagnoses by having a computer look at a bone marrow biopsy slide.”
Methodology
Srisuwananukorn and colleagues developed a deep-learning algorithm aimed at diagnosing prefibrotic primary myelofibrosis and essential thrombocythemia using digitized diagnostic bone marrow biopsy whole-slide images. The researchers created a model to provide hematopathologists with an accurate, faster and less expensive diagnostic tool to differentiate between the two conditions.
The training cohort used to inform the deep-learning algorithm comprised patients with a diagnosis of prefibrotic primary myelofibrosis or essential thrombocythemia who received care at University of Florence between June 2007 and May 2023 and at Moffitt Cancer Center between January 2013 and January 2022.
The final training cohort included 100 patients with each condition. Researchers also developed an additional external testing cohort of 26 patients.
The model used 32,226 digitized bone marrow biopsy slides from the training cohort to inform the algorithm.
Investigators measured the model’s diagnostic performance using the area under the receiver operating characteristic curve (AUC) analysis.
Key findings
Results showed that 5-fold cross validation within the training cohort yielded a mean AUC of 0.90 ± 0.04.
Further analysis using a finalized model retrained with slides from the entire training cohort revealed an AUC of 0.90 for the external testing cohort.
Researchers reported 92.3% diagnostic classification accuracy for the external testing cohort, with 66.6% sensitivity and 100% specificity for a diagnosis of prefibrotic primary myelofibrosis.
The model processed previously unseen slides in approximately 6.1 seconds using consumer-grade computing hardware. This included 4.9 seconds for preprocessing and 1.2 seconds for slide evaluation.
Clinical implications
Despite its accuracy, the AI-powered algorithm is not ready to navigate the regulatory framework for clinical practice use, Srisuwananukorn said.
“With these results, we hope to show physicians that these types of AI-based tools are upcoming,” he added.
Srisuwananukorn said there are many valid criticisms about the use of AI in medicine and he believes a regulatory framework governing the use of AI tools in a clinical setting is necessary to ensure accuracy and consistency.
“There is hesitation among the scientific and clinical communities to implement artificial intelligence decision tools within clinical practice — and there should be resistance,” he said. “It is unlikely that a tool like this will replace human judgement, but I hope it will instead complement the physician.”