Omitting impact of race, ethnicity results in less accurate cancer risk prediction models
Key takeaways:
- Addition of race and ethnicity as a risk predictor improved recurrence risk algorithm fairness.
- The decision of whether to include race or ethnicity factors in risk models should be made on a case-by-case basis.
Prognostic models for risk for postoperative cancer recurrence that omit race and ethnicity as predictors may be less accurate for individuals from historically underserved populations, retrospective study results showed.
Removing race and ethnicity from risk prediction algorithms for colorectal cancer recurrence has a deleterious effect on model fairness that could result in “inappropriate care recommendations” for historically underserved patient populations, investigators wrote in JAMA Network Open.

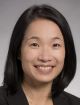
“It is important to evaluate subgroup-specific performances of risk models to ensure that the prediction models are not performing worse among minoritized racial and ethnic groups,” Sara Khor, MASc, a PhD student, and Aasthaa Bansal, PhD, associate professor at University of Washington’s Comparative Health Outcomes, Policy, and Economics (CHOICE) Institute, told Healio in a joint statement. “Poor predictive accuracy can lead to inappropriate health care decisions that can have real health consequences for these patients.”
Background
Motivation for this analysis stems from an ongoing debate about whether race and ethnicity should be included as risk factors in clinical risk prediction models, according to Khor and Bansal.
Many people in health care research called for the removal of race and ethnicity as risk factors in prediction models due to concerns about racial profiling, or because they feel race/ethnicity fail to represent a specific patient’s individual risk profile, they added.
“We wanted to better understand — using colorectal cancer recurrence as a case study — what some of the implications might be if we simply remove race and ethnicity as a predictor in a risk prediction algorithm,” Kohr and Bansal said. “We wanted to contribute to this debate by empirically studying whether the simple removal of race as a predictor in the colorectal cancer recurrence setting would ultimately benefit the minoritized racial groups in terms of recommending more appropriate care.”
Methodology
Khor and colleagues conducted a prognostic study to determine whether including race and ethnicity as a predictor is associated with racial bias when included as part of a colorectal cancer recurrence risk algorithm.
The investigators defined racial bias as “racial and ethnic differences in model accuracy that could potentially lead to unequal treatment.”
The retrospective analysis used linked cancer registry and electronic health record data from Kaiser Permanente Southern California. It included 4,230 patients (mean age, 65.3 ± 12.5 years; 48.1% women; 11.6 Asian, Hawaiian or Pacific Islander; 13.1% Black or African American; 22.1% Hispanic; 53.1% non-Hispanic white) diagnosed with stage I to stage III colorectal cancer who underwent surgical resection between 2008 and 2013.
Colorectal cancer recurrence served as the study’s primary outcome measurement.
Researchers developed four separate hazard regression prediction models: a race-neutral model that explicitly excluded race and ethnicity as a predictor; a race-sensitive model that included race and ethnicity; a model with two-way interactions between clinical predictors and race and ethnicity; and separate models by race and ethnicity.
Assessment of algorithmic fairness included model calibration, discriminative ability, false-positive and false-negative rates, positive predictive value and negative predictive value.
Key findings
Results showed the race-neutral model had worse calibration, negative predictive value and false-negative rates among patients from racial and ethnic minority groups compared with non-Hispanic white individuals.
For example, although researchers noted “excellent” overall calibration for the race-neutral model (calibration intercept, 0 [95% CI, 0.02 to 0.02]; slope = 1 [95% CI, 0.85 to 1.14]), they observed less robust calibration among Black or African American patients (slope = 1.51 (95% CI, 1.08 to 1.93).
This finding suggested the model tended to overpredict colorectal cancer recurrence risk for low-risk individuals and underpredict risk for high-risk individuals, according to the researchers.
The addition of race and ethnicity as a predictor improved recurrence risk algorithm fairness in calibration slope, discriminative ability, positive predictive value and false-negative rates.
Clinical implications
Context is paramount regarding the decision whether to include race or ethnicity factors in risk models, according to Kohr and Bansal.
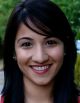
There is no one singular approach, and the benefits and drawbacks about whether to include such factors likely should be determined on a case-by-case basis, they added.
“When developing a clinical risk prediction algorithm, one should consider the potential racial or ethnic biases present in clinical practice that translate to bias in the data,” they told Healio. “Care must be taken to think through implications of such biases during the algorithm development and evaluation process in order to avoid further propagating those biases.”
Data analytics are increasingly being deployed as part of health care decision making, especially with respect to the use of risk prediction models, Ankur Pandya, PhD, of Harvard T.H. Chan School of Public Health, and Jinyi Zhu, PhD, of Vanderbilt University School of Medicine wrote in an accompanying editorial.
However, unrestrained use of data analytics-driven algorithms that are race or ethnicity-neutral may exacerbate existing inequalities in health care delivery, they added.
“As shown by Khor and colleagues, there is no one-size-fits-all solution ... to confronting algorithmic bias,” Pandya and Zhu wrote. “When possible, approaches for identifying and responding to algorithmic bias should focus on the decisions made by patients and policy makers as they relate to the ultimate outcomes of interest ... and the distribution of these outcomes across the subgroups that define important health disparities.”
References:
- Khor S, et al. JAMA Netw Open. 2023;doi:10.1001/jamanetworkopen.2023.18495.
- Pandya A, et al. JAMA Netw Open. 2023;doi:10.1001/jamanetworkopen.2023.18501.
For more information:
Sara Khor, MASc, and Aasthaa Bansal, PhD, can be reached at Comparative Health Outcomes, Policy, and Economics (CHOICE) Institute, University of Washington, 1959 NE Pacific St., HSB H-375, Box 357630, Seattle, WA 98195; email: khors@uw.edu or abansal@uw.edu.