AI tool accurately predicts colorectal cancer aggressiveness, patient survival outcomes
An artificial intelligence tool developed at Harvard Medical School and National Cheng Kung University in Taiwan correctly predicted the aggressiveness of colorectal cancer.
The tool, named MOMA (Multi-omics Multi-cohort Assessment), evaluates microscopic images of tumor samples to predict cancer aggressiveness, the likelihood of survival with or without recurrence, and actionable genomic mutations in colorectal cancer.

“There are two major things we can do with our published models — one is to tailor treatments based on the aggressiveness of the tumor as informed by our AI model,” researcher Kun-Hsing Yu, MD, PhD, assistant professor of biomedical informatics at Blavatnik Institute of Harvard Medical School, told Healio. “We also believe the molecular predictions we have developed will have direct clinical implications in terms of timely and affordable access to molecular testing.”
Yu spoke with Healio about the origin of this tool, how it works and plans for its future development.
Healio: How did this project come to be?
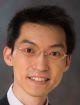
Yu: We started this project around 2 years ago when we observed a lot of heterogeneity in the survival outcomes of our patients. So, we plotted a few exploratory statistics showing that although patients with stage I and stage II cancer usually have better survival, some just don’t respond well to standard treatment, which may involve surgery plus or minus chemotherapy or radiotherapy.
We became very intrigued and looked into the available information from this patient cohort. In current clinical diagnosis, we always need to take a piece of tissue from the cancerous lesion and use a microscope to visualize the tumor samples. Based on the expertise of pathologists, we define different subtypes of cancer. Many of them are related to prognosis. But even when we factor in all the known information, we still cannot provide a definite prediction of patient survival outcomes.
Another thing we touch upon in this paper is the molecular subtype classification using microscopic images of the cancer samples. This is not something pathologists do using their microscopes, because it would require additional genomic sequencing. We simply don’t know what visual features are correlated with molecular patterns.
That was the start of the project. In our laboratory, we are primarily focused on AI for pathology. So, we aimed to devise new AI tools to solve this clinical problem. Fortunately, the project went well and we had some exciting results in terms of predicting early-stage and also late-stage patient survival outcomes using pathology imaging, as well as predicting molecular subtypes.
Healio: How did you train this AI tool?
Yu: We used a weakly supervised machine learning approach. In the training phase, we needed to enter some input data and the desired output into our machine learning algorithm.
For example, in the case of survival prediction in the training phase, we showed the machine all pathology images and survival outcomes of the patients. After several rounds of training, our algorithms started to recognize the associations between input and output. They started to derive some signals from the images and used those signals to make future predictions.
We initially trained our model on a large study cohort from the Cancer Genome Atlas, which is funded by the NCI. We developed a preliminary model from that. We collected additional data from multiple patient populations, including the Prostate, Lung, Colorectal and Ovarian (PLCO) trial, another NCI-funded study, and also our local Nurses’ Health Study and our Health Professional Follow-up Study led here by investigators at Harvard.
Healio: How did the tool perform in your study?
Yu: Based on our extensive validation, we showed that the association found by our AI model truly works and is generalizable.
The model was able to identify some features that were associated with poor outcomes, including a greater density of cells within the tumor, the presence of stroma around tumor cells and the interaction of tumor cells with smooth muscle cells. It also found patterns within tumor stroma that were indicative of longer recurrence-free survival and identified which patients could benefit the most from immune checkpoint inhibitors.
Healio: What is next in your plans for this project?
Yu: One missing piece we are working on in our follow-up development will be a user-friendly interface, where clinicians without any training in informatics can easily assess our tools to make clinical judgments.
The immediate next step, in addition to the user interface, would be the prospective clinical trial that we are setting up. We want to show prospectively that our tool is making a difference in the clinic and doesn’t disrupt the current clinical workflow for cancer diagnosis.
Healio: Is there anything else you would like to mention?
Yu: Another major accomplishment of our work is that we have devised an explainable AI approach. In previous studies, people who used AI for clinical decision-making largely treated AI as a black box. This is simply because the current machine learning approach is so complicated, even experts can’t truly understand all parameters a model used. We attempted to connect our AI model with explainable pathology concepts that clinicians and biologists already know. We connect our prediction model, which is quite powerful, to those pathology concepts in order to communicate what our machines found to researchers and clinicians. With the pathology insights from AI, in the future we might be able to redesign our treatment based on the relationships we find between the tumor microenvironment and treatment responses.
For more information:
Kun-Hsing Yu, MD, PhD, can be reached at Yu Lab, Harvard Medical School, 10 Shattuck St., Boston, MA 02115; email: kun-hsing_yu@hms.harvard.edu.